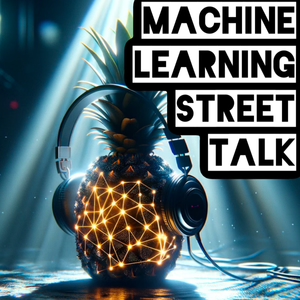
Machine Learning Street Talk (MLST)
Machine Learning Street Talk (MLST)

2 Listeners
All episodes
Best episodes
Seasons
Top 10 Machine Learning Street Talk (MLST) Episodes
Goodpods has curated a list of the 10 best Machine Learning Street Talk (MLST) episodes, ranked by the number of listens and likes each episode have garnered from our listeners. If you are listening to Machine Learning Street Talk (MLST) for the first time, there's no better place to start than with one of these standout episodes. If you are a fan of the show, vote for your favorite Machine Learning Street Talk (MLST) episode by adding your comments to the episode page.
Featured in these lists
FAQ
How many episodes does Machine Learning Street Talk (MLST) have?
Machine Learning Street Talk (MLST) currently has 199 episodes available.
What topics does Machine Learning Street Talk (MLST) cover?
The podcast is about Podcasts and Technology.
What is the most popular episode on Machine Learning Street Talk (MLST)?
The episode title 'Nicholas Carlini (Google DeepMind)' is the most popular.
What is the average episode length on Machine Learning Street Talk (MLST)?
The average episode length on Machine Learning Street Talk (MLST) is 97 minutes.
How often are episodes of Machine Learning Street Talk (MLST) released?
Episodes of Machine Learning Street Talk (MLST) are typically released every 5 days, 19 hours.
When was the first episode of Machine Learning Street Talk (MLST)?
The first episode of Machine Learning Street Talk (MLST) was released on Apr 24, 2020.
Show more FAQ

Show more FAQ