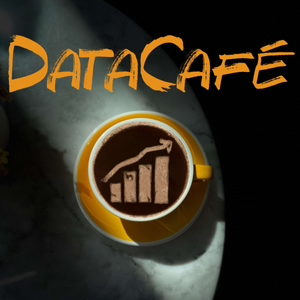
DataCafé
Jason & Jeremy
Welcome to the DataCafé: a special-interest Data Science podcast with Dr Jason Byrne and Dr Jeremy Bradley, interviewing leading data science researchers and domain experts in all things business, stats, maths, science and tech.
All episodes
Best episodes
Seasons
Top 10 DataCafé Episodes
Best episodes ranked by Goodpods Users most listened
No results found...
FAQ
How many episodes does DataCafé have?
DataCafé currently has 26 episodes available.
What topics does DataCafé cover?
The podcast is about Podcasts, Technology, Science, Data Analytics, Artificial Intelligence, Data Science and Machine Learning.
What is the most popular episode on DataCafé?
The episode title 'Scaling the Internet' is the most popular.
What is the average episode length on DataCafé?
The average episode length on DataCafé is 32 minutes.
How often are episodes of DataCafé released?
Episodes of DataCafé are typically released every 28 days, 14 hours.
When was the first episode of DataCafé?
The first episode of DataCafé was released on Jun 1, 2020.
Show more FAQ

Show more FAQ
Comments
0.0
out of 5
No ratings yet