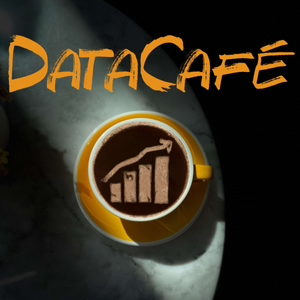
Apple Tasting: Reinforcement learning for quality control
02/22/21 • 35 min
Have you ever come home from the supermarket to discover one of the apples you bought is rotten? It's likely your trust for that grocer was diminished, or you might stop buying that particular brand of apples altogether.
In this episode, we discuss how the quality controls in a production line need to use smart sampling methods in order to avoid sending bad products to the customer, which could ruin the reputation of both the brand and seller.
To do this we describe a thought experiment called Apple Tasting. This allows us to demonstrate the concepts of regret and reward in a sampling process, giving rise to the use of Contextual Bandit Algorithms. Contextual Bandits come from the field of Reinforcement Learning which is a form of Machine Learning where an agent performs an action and tries to maximise the cumulative reward from its environment over time. Standard bandit algorithms simply choose between a number of actions and measure the reward in order to determine the average reward of each action. But a Contextual Bandit also uses information from its environment to inform both the likely reward and regret of subsequent actions. This is particularly useful in personalised product recommendation engines where the bandit algorithm is given some contextual information about the user.
Back to Apple Tasting and product quality control. The contextual bandit in this scenario, consumes a signal from a benign test that is indicative, but not conclusive, of there being a fault and then makes the decision to perform a more in-depth test or not. So the answer for when you should discard or test your product depends on the relative costs of making the right decision (reward) or wrong decision (regret) and how your experience of the environment affected these in the past.
We speak with Prof. David Leslie about how this logic can be applied to any manufacturing pipeline where there is a downside risk of not quality checking the product but a cost in a false positive detection of a bad product.
Other areas of application include:
- Anomalous behaviour in a jet engine e.g. low fuel efficiency, which could be nothing or could be serious, so it might be worth taking the plane in for repair.
- Changepoints in network data time series - does it mean there’s a fault on the line or does it mean the next series of The Queen’s Gambit has just been released? Should we send an engineer out?
With interview guest David Leslie, Professor of Statistical Learning in the Department of Mathematics and Statistics at Lancaster University.
Further Reading
- Publication list for Prof. David Leslie (http://bitly.ws/bQ4a via Lancaster University)
- Paper on "Selecting Multiple Web Adverts - a Contextual Multi-armed Bandit with State Uncertainty" in Journal of the ORS (http://bitly.ws/bQ3X via Lancaster University)
- Paper on "Apple tasting" (http://bitly.ws/bQeW via ScienceDirect)
- Paper by Google Inc. on "AutoML for Contextual Bandits" (https://arxiv.org/abs/1909.03212 via arXiv)
Thanks for joining us in the DataCafé. You can follow us on twitter @DataCafePodcast and feel free to contact us about anything you've heard here or think would be an interesting topic in the future.
Have you ever come home from the supermarket to discover one of the apples you bought is rotten? It's likely your trust for that grocer was diminished, or you might stop buying that particular brand of apples altogether.
In this episode, we discuss how the quality controls in a production line need to use smart sampling methods in order to avoid sending bad products to the customer, which could ruin the reputation of both the brand and seller.
To do this we describe a thought experiment called Apple Tasting. This allows us to demonstrate the concepts of regret and reward in a sampling process, giving rise to the use of Contextual Bandit Algorithms. Contextual Bandits come from the field of Reinforcement Learning which is a form of Machine Learning where an agent performs an action and tries to maximise the cumulative reward from its environment over time. Standard bandit algorithms simply choose between a number of actions and measure the reward in order to determine the average reward of each action. But a Contextual Bandit also uses information from its environment to inform both the likely reward and regret of subsequent actions. This is particularly useful in personalised product recommendation engines where the bandit algorithm is given some contextual information about the user.
Back to Apple Tasting and product quality control. The contextual bandit in this scenario, consumes a signal from a benign test that is indicative, but not conclusive, of there being a fault and then makes the decision to perform a more in-depth test or not. So the answer for when you should discard or test your product depends on the relative costs of making the right decision (reward) or wrong decision (regret) and how your experience of the environment affected these in the past.
We speak with Prof. David Leslie about how this logic can be applied to any manufacturing pipeline where there is a downside risk of not quality checking the product but a cost in a false positive detection of a bad product.
Other areas of application include:
- Anomalous behaviour in a jet engine e.g. low fuel efficiency, which could be nothing or could be serious, so it might be worth taking the plane in for repair.
- Changepoints in network data time series - does it mean there’s a fault on the line or does it mean the next series of The Queen’s Gambit has just been released? Should we send an engineer out?
With interview guest David Leslie, Professor of Statistical Learning in the Department of Mathematics and Statistics at Lancaster University.
Further Reading
- Publication list for Prof. David Leslie (http://bitly.ws/bQ4a via Lancaster University)
- Paper on "Selecting Multiple Web Adverts - a Contextual Multi-armed Bandit with State Uncertainty" in Journal of the ORS (http://bitly.ws/bQ3X via Lancaster University)
- Paper on "Apple tasting" (http://bitly.ws/bQeW via ScienceDirect)
- Paper by Google Inc. on "AutoML for Contextual Bandits" (https://arxiv.org/abs/1909.03212 via arXiv)
Thanks for joining us in the DataCafé. You can follow us on twitter @DataCafePodcast and feel free to contact us about anything you've heard here or think would be an interesting topic in the future.
Previous Episode
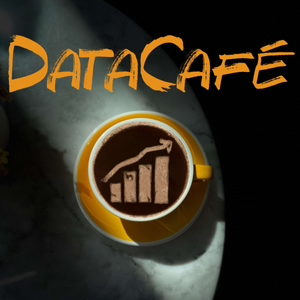
Optimising the Future
As we look ahead to a new year, and reflect on the last, we consider how data science can be used to optimise the future. But to what degree can we trust past experiences and observations, essentially relying on historical data to predict the future? And with what level of accuracy?
In this episode of the DataCafé we ask: how can we optimise our predictions of future scenarios to maximise the benefit we can obtain from them while minimising the risk of unknowns?
Data Science is made up of many diverse technical disciplines that can help to answer these questions. Two among them are mathematical optimisation and machine learning. We explore how these two fascinating areas interact and how they can both help to turbo charge the other's cutting edge in the future.
We speak with Dimitrios Letsios from King's College London about his work in optimisation and what he sees as exciting new developments in the field by working together with the field of machine learning.
With interview guest Dr. Dimitrios Letsios, lecturer (assistant professor) in the Department of Informatics at King's College London and a member of the Algorithms and Data Analysis Group.
Further reading
- Dimirios Letsios' publication list (https://bit.ly/35vHirH via King's College London)
- Paper on taking into account uncertainty in an optimisation model: Approximating Bounded Job Start Scheduling with Application in Royal Mail Deliveries under Uncertainty (https://bit.ly/3pLHICV via King's College London)
- Paper on lexicographic optimisation: Exact Lexicographic Scheduling and Approximate Rescheduling (https://bit.ly/3rS8Xxk via arXiv)
- Paper on combination of AI and Optimisation: Argumentation for Explainable Scheduling (https://bit.ly/3oobgGF via AAAI Conference on Artificial Intelligence)
Some links above may require payment or login. We are not endorsing them or receiving any payment for mentioning them. They are provided as is. Often free versions of papers are available and we would encourage you to investigate.
Recording date: 23 October 2020
Interview date: 21 February 2020
Intro music by Music 4 Video Library (Patreon supporter)
Thanks for joining us in the DataCafé. You can follow us on twitter @DataCafePodcast and feel free to contact us about anything you've heard here or think would be an interesting topic in the future.
Next Episode
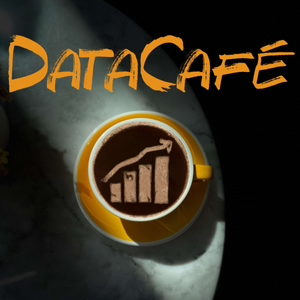
Bayesian Inference: The Foundation of Data Science
In this episode we talk about all things Bayesian. What is Bayesian inference and why is it the cornerstone of Data Science?
Bayesian statistics embodies the Data Scientist and their role in the data modelling process. A Data Scientist starts with an idea of how to capture a particular phenomena in a mathematical model - maybe derived from talking to experts in the company. This represents the prior belief about the model. Then the model consumes data around the problem - historical data, real-time data, it doesn't matter. This data is used to update the model and the result is called the posterior.
Why is this Data Science? Because models that react to data and refine their representation of the world in response to the data they see are what the Data Scientist is all about.
We talk with Dr Joseph Walmswell, Principal Data Scientist at life sciences company Abcam, about his experience with Bayesian modelling.
Further Reading
- Publication list for Dr. Joseph Walmswell (https://bit.ly/3s8xluH via researchgate.net)
- Blog on Bayesian Inference for parameter estimation (https://bit.ly/2OX46fV via towardsdatascience.com)
- Book Chapter on Bayesian Inference (https://bit.ly/2Pi9Ct9 via cmu.edu)
- Article on The Monty Hall problem (https://bit.ly/3f1pefr via Wikipedia)
- Podcast on "The truth about obesity and Covid-19", More or Less: Behind the Stats podcast (https://bbc.in/3lBqCGS via bbc.co.uk)
- Gov.uk guidance:
Some links above may require payment or login. We are not endorsing them or receiving any payment for mentioning them. They are provided as is. Often free versions of papers are available and we would encourage you to investigate.
Recording date: 16 March 2021
Interview date: 26 February 2021
Thanks for joining us in the DataCafé. You can follow us on twitter @DataCafePodcast and feel free to contact us about anything you've heard here or think would be an interesting topic in the future.
DataCafé - Apple Tasting: Reinforcement learning for quality control
Transcript
Jason 0:00
Hello, and welcome to the DataCafé. I'm Jason.
Jeremy 0:04
And I'm Jeremy. And today we're talking about Apple tasting.
Jason 0:10
Apple tasting, we're gonna have to give a bit more context for this one, I think, what are we talking about with Apple tasting? Jeremy?
Jeremy 0:17
Well, context is everything as we'll find out. Yeah, I mean, this is a bit of fun. This is a scenario where you have a conveyor belt of apples going in front of yo
If you like this episode you’ll love
Episode Comments
Generate a badge
Get a badge for your website that links back to this episode
<a href="https://goodpods.com/podcasts/datacaf%c3%a9-241830/apple-tasting-reinforcement-learning-for-quality-control-26902048"> <img src="https://storage.googleapis.com/goodpods-images-bucket/badges/generic-badge-1.svg" alt="listen to apple tasting: reinforcement learning for quality control on goodpods" style="width: 225px" /> </a>
Copy