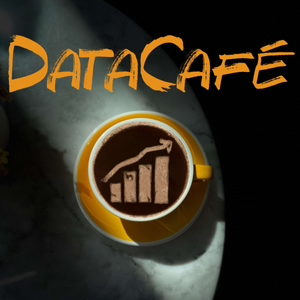
Changepoint Detection: Secret Weapon of the Data Scientist
07/13/20 • 31 min
How can we spot a change in a jet engine vibration that might mean it’s about to fail catastrophically? How can a service forecast adapt to unexpected changes brought about by a pandemic? How might we spot an increase in rate of change of pollution in the atmosphere? The answer to all these questions is changepoints, or rather changepoint detection.
Common to all these systems is a set of ordered data, usually a time series of observations or measurements that may be noisy but have some underlying pattern. As the world changes, so those changes might lead to dramatic changes in the measurements and a disruption of the usual pattern. Unless these forecasts or failure-detection systems are updated quickly to take account of a change in measurement data, they will likely produce erroneous or unpredictable results.
Changepoints have many important applications in areas such as:
- Climatology
- Genetic sequencing
- Finance
- Medical imaging
- Forecasting in industry
We speak to statistician Dr. Rebecca Killick from Lancaster University about her work in changepoint detection and how it is a critical part of the statistical toolkit for analysing time series and other ordered data sets. In particular:
- In forecasting where most methods tend to work on the basis of extrapolating trends, it is essential to know if a changepoint has occurred so that a refreshed model calculation can be started.
- If there is a change in the underlying dynamics of a system that causes a complex change in the observed output then this can often be detected with a changepoint. This might be indicative of a mechanical failure or impending change in operation or an unobserved event buried deep in a difficult-to-measure environment, like a nuclear reactor.
With interview guest Dr. Rebecca Killick, Associate Professor of Statistics at Lancaster University.
Further reading
- Rebecca Killick’s publications (via Lancaster University)
- Changepoints Overview Paper: changepoint: An R package for changepoint analysis (pdf via Journal of Statistical Software)
- R Package: changepoint: Methods for Changepoint Detection (R package via CRAN library)
- PELT algorithm paper: Optimal detection of changepoints with a linear computational cost (pdf via arXiv)
- Paper: Distinguishing Trends and Shifts from Memory in Climate Data (paper via American Meteorological Society)
- R Package: EnvCpt: Detection of Structural Changes in Climate and Environment Time Series (R package via CRAN library)
Some links above may require payment or login. We are not endorsing them or receiving any payment for mentioning them. They are provided as is. Often free versions of papers are available and we would encourage you to investigate.
Recording date: 10 June 2020
Interview date: 5 June 2020
Thanks for joining us in the DataCafé. You can follow us on twitter @DataCafePodcast and feel free to contact us about anything you've heard here or think would be an interesting topic in the future.
How can we spot a change in a jet engine vibration that might mean it’s about to fail catastrophically? How can a service forecast adapt to unexpected changes brought about by a pandemic? How might we spot an increase in rate of change of pollution in the atmosphere? The answer to all these questions is changepoints, or rather changepoint detection.
Common to all these systems is a set of ordered data, usually a time series of observations or measurements that may be noisy but have some underlying pattern. As the world changes, so those changes might lead to dramatic changes in the measurements and a disruption of the usual pattern. Unless these forecasts or failure-detection systems are updated quickly to take account of a change in measurement data, they will likely produce erroneous or unpredictable results.
Changepoints have many important applications in areas such as:
- Climatology
- Genetic sequencing
- Finance
- Medical imaging
- Forecasting in industry
We speak to statistician Dr. Rebecca Killick from Lancaster University about her work in changepoint detection and how it is a critical part of the statistical toolkit for analysing time series and other ordered data sets. In particular:
- In forecasting where most methods tend to work on the basis of extrapolating trends, it is essential to know if a changepoint has occurred so that a refreshed model calculation can be started.
- If there is a change in the underlying dynamics of a system that causes a complex change in the observed output then this can often be detected with a changepoint. This might be indicative of a mechanical failure or impending change in operation or an unobserved event buried deep in a difficult-to-measure environment, like a nuclear reactor.
With interview guest Dr. Rebecca Killick, Associate Professor of Statistics at Lancaster University.
Further reading
- Rebecca Killick’s publications (via Lancaster University)
- Changepoints Overview Paper: changepoint: An R package for changepoint analysis (pdf via Journal of Statistical Software)
- R Package: changepoint: Methods for Changepoint Detection (R package via CRAN library)
- PELT algorithm paper: Optimal detection of changepoints with a linear computational cost (pdf via arXiv)
- Paper: Distinguishing Trends and Shifts from Memory in Climate Data (paper via American Meteorological Society)
- R Package: EnvCpt: Detection of Structural Changes in Climate and Environment Time Series (R package via CRAN library)
Some links above may require payment or login. We are not endorsing them or receiving any payment for mentioning them. They are provided as is. Often free versions of papers are available and we would encourage you to investigate.
Recording date: 10 June 2020
Interview date: 5 June 2020
Thanks for joining us in the DataCafé. You can follow us on twitter @DataCafePodcast and feel free to contact us about anything you've heard here or think would be an interesting topic in the future.
Previous Episode
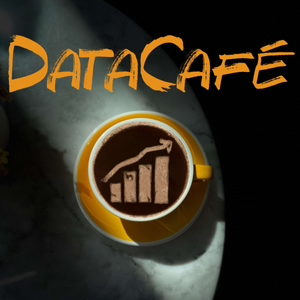
Optimal Control in Price Decision Making
Optimal Control is the science of making decisions in a way that optimises a key quantity such as revenue, customer satisfaction, or quality of service.
Cake example
Bertrand has a cake. He likes cake a lot but he can overeat cake sometimes in which case he doesn’t enjoy it so much. He would like to work out how much cake he should eat today and the next and the next so that he maximises his overall enjoyment of the cake, possibly making it last a long time (but not so long that it goes stale). The development of this decision strategy is a good example of optimal control.
Airline example
When selling tickets to customers, airlines face the problem of setting the right price, which allows them to both get a satisfactory instantaneous reward but also to reserve some capacity for later demand, typically associated with a higher willingness to pay. In this context, how can they make sure such a right price is offered to the customer at each moment of time?
Interview guest Dr. Manuel Offidani, Data Scientist at easyJet.
https://uk.linkedin.com/in/manuel-offidani
Further Reading
Paper: An Optimal Control Problem of Dynamic Pricing (summary via researchgate)
Book: The Theory and Practice of Revenue Management (contents via Springer)
Book: Dynamic Programming and Optimal Control (summary via researchgate)
Some links above may require payment or login. We are not endorsing them or receiving any payment for mentioning them. They are provided as is. Often free versions of papers are available and we would encourage you to investigate.
Recording date: 6 Mar. 2020
Interview date: 7 Feb. 2020
Additional sound effects from https://www.zapsplat.com
Thanks for joining us in the DataCafé. You can follow us on twitter @DataCafePodcast and feel free to contact us about anything you've heard here or think would be an interesting topic in the future.
Next Episode
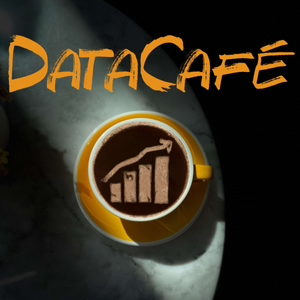
Viruses: Keep Calm and Use Statistics
What is a virus? How can we spot human viruses in danger of becoming pandemics? How can we use statistics to understand their origins and transmission? This turns out to be a hard problem - not least because there can be many hundreds or thousands of slightly modified strains of a virus in a small sample of blood. It is of great importance which version of a virus will become a pandemic in a population and which will merely peter out.
Viral geneticists have to be expert statisticians to be able to disentangle this story. Fundamentally if we can use statistical techniques to understand which versions of a virus are prevalent and where they originated from we can start to design counter measures to defeat the further spread of the virus.
We speak to statistician and data scientist Dr. Kat James about her DPhil and post-doctoral work on the statistical genetics of animal-human viruses, in particular HIV-2, at the Nuffield Department of Medicine and the Wellcome Trust Centre for Human Genetics, University of Oxford. She is now Head of Data Science at Royal Mail and has some some valuable insights on the crossover between statistical genetics and data science.
As we discover, the current coronavirus pandemic is a so-called zoonotic virus - which means it transitioned from animals to humans at some point and has become a very successful virus in the human population. COVID-19 has similarities to influenza, HIV-1 and HIV-2, MERS and SARS as we will discover in this episode and Kat gives us some interesting lessons to learn from previous pandemics.
Background on HIV
HIV-1 is one of the major viral pandemics of the 20th century . Untreated, it has a greater than 95% probability of death and it has killed 33 million people (it still accounts for 750,000 deaths per year).
Using statistical genetics, researchers have been able to identify 3 spillover events into humans for HIV-1. Human viruses often interact with developments in human geography as part of the infection dynamics and this is certainly true of HIV-1 over the course of its emergence as a pandemic virus.
HIV-2 is a distinct but similar virus to HIV-1 and people who are infected with HIV-2 often demonstrate resistance to HIV-1. Eight spillover events from Mangabey monkeys have been identified for HIV-2.
With interview guest Dr. Kat James who is now Head of Data Science at Royal Mail.
Further reading
- Paper: Low-Bias RNA Sequencing of the HIV-2 Genome from Blood Plasma (via Journal of Virology, American Society for Microbiology)
- Article: Introduction to PCR amplification (via Kahn Academy)
- Article: Tracking COVID19: Coronavirus came to UK 'on at least 1,300 separate occasions' (reporting on work by Universities of Birmingham and Oxford via BBC News website)
Some links above may require payment or login. We are not endorsing them or receiving any payment for mentioning them. They are provided as is. Often free versions of papers are available and we would encourage you to investigate.
Recording date: 7 July 2020
Interview date: 9 June 2020
Thanks for joining us in the DataCafé. You can follow us on twitter @DataCafePodcast and feel free to contact us about anything you've heard here or think would be an interesting topic in the future.
If you like this episode you’ll love
Episode Comments
Generate a badge
Get a badge for your website that links back to this episode
<a href="https://goodpods.com/podcasts/datacaf%c3%a9-241830/changepoint-detection-secret-weapon-of-the-data-scientist-26902054"> <img src="https://storage.googleapis.com/goodpods-images-bucket/badges/generic-badge-1.svg" alt="listen to changepoint detection: secret weapon of the data scientist on goodpods" style="width: 225px" /> </a>
Copy