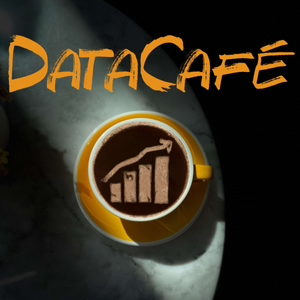
DataCafé
Jason & Jeremy
All episodes
Best episodes
Seasons
Top 10 DataCafé Episodes
Goodpods has curated a list of the 10 best DataCafé episodes, ranked by the number of listens and likes each episode have garnered from our listeners. If you are listening to DataCafé for the first time, there's no better place to start than with one of these standout episodes. If you are a fan of the show, vote for your favorite DataCafé episode by adding your comments to the episode page.
![DataCafé - [Bite] Data Science and the Scientific Method](https://storage.googleapis.com/goodpods-images-bucket/episode_images/569bce89842fdc0ba27d500e5cf174a7bee7dd3a5e753626e118db12bdadd236.avif)
05/03/21 • 17 min
The scientific method consists of systematic observation, measurement, and experiment, and the formulation, testing, and modification of hypotheses. But what does this mean in the context of Data Science, where a wealth of unstructured data and variety of computational models can be used to deduce an insight and inform a stakeholder's decision?
In this bite episode we discuss the importance of the scientific method for data scientists. Data science is, after all, the application of scientific techniques and processes to large data sets to obtain impact in a given application area. So we ask how the scientific method can be harnessed efficiently and effectively when there is so much uncertainty in the design and interpretation of an experiment or model.
Further Reading and Resources
- Paper: "Defining the scientific method" via Nature https://www.nature.com/articles/nmeth0409-237
- Paper: "Big data: the end of the scientific method" via The Royal Society https://royalsocietypublishing.org/doi/10.1098/rsta.2018.0145
- Article: "The Data Scientific Method" via Medium https://towardsdatascience.com/a-data-scientific-method-80caa190dbd4
- Article: "The scientific method of machine learning" via Datascience.aero https://datascience.aero/scientific-method-machine-learning/
- Article: "Putting the 'Science' Back in Data Science" via KDnuggets https://www.kdnuggets.com/2017/09/science-data-science.html
- Podcast: "In Our Time: The Scientific Method" via BBC Radio 4 https://www.bbc.co.uk/programmes/b01b1ljm
- Podcast: "The end of the scientific method" via The Economist https://www.economist.com/podcasts/2019/11/27/the-end-of-the-scientific-method
- Video: "The Scientific Method" via Coursera https://www.coursera.org/lecture/data-science-fundamentals-for-data-analysts/the-scientific-method-Ha5hq
- Cartoon: "Machine Learning" via xkcd https://xkcd.com/1838/
Some links above may require payment or login. We are not endorsing them or receiving any payment for mentioning them. They are provided as is. Often free versions of papers are available and we would encourage you to investigate.
Recording date: 30 April 2021
Intro music by Music 4 Video Library (Patreon supporter)
Thanks for joining us in the DataCafé. You can follow us on twitter @DataCafePodcast and feel free to contact us about anything you've heard here or think would be an interesting topic in the future.
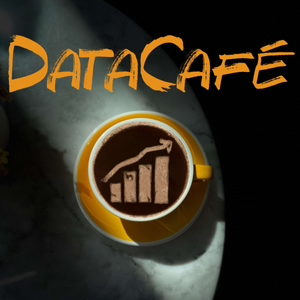
06/01/20 • 27 min
Optimal Control is the science of making decisions in a way that optimises a key quantity such as revenue, customer satisfaction, or quality of service.
Cake example
Bertrand has a cake. He likes cake a lot but he can overeat cake sometimes in which case he doesn’t enjoy it so much. He would like to work out how much cake he should eat today and the next and the next so that he maximises his overall enjoyment of the cake, possibly making it last a long time (but not so long that it goes stale). The development of this decision strategy is a good example of optimal control.
Airline example
When selling tickets to customers, airlines face the problem of setting the right price, which allows them to both get a satisfactory instantaneous reward but also to reserve some capacity for later demand, typically associated with a higher willingness to pay. In this context, how can they make sure such a right price is offered to the customer at each moment of time?
Interview guest Dr. Manuel Offidani, Data Scientist at easyJet.
https://uk.linkedin.com/in/manuel-offidani
Further Reading
Paper: An Optimal Control Problem of Dynamic Pricing (summary via researchgate)
Book: The Theory and Practice of Revenue Management (contents via Springer)
Book: Dynamic Programming and Optimal Control (summary via researchgate)
Some links above may require payment or login. We are not endorsing them or receiving any payment for mentioning them. They are provided as is. Often free versions of papers are available and we would encourage you to investigate.
Recording date: 6 Mar. 2020
Interview date: 7 Feb. 2020
Additional sound effects from https://www.zapsplat.com
Thanks for joining us in the DataCafé. You can follow us on twitter @DataCafePodcast and feel free to contact us about anything you've heard here or think would be an interesting topic in the future.
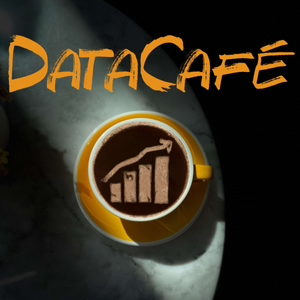
A Culture of Innovation
DataCafé
09/06/22 • 33 min
Culture is a key enabler of innovation in an organisation. Culture underpins the values that are important to people and the motivations for their behaviours. When these values and behaviours align with the goals of innovation, it can lead to high performance across teams that are tasked with the challenge of leading, inspiring and delivering innovation. Many scientists and researchers are faced with these challenges in various scenarios, yet may be unaware of the level of influence that comes from the culture they are part of.
In this episode we talk about what it means to design and embed a culture of innovation. We outline some of our findings in literature about the levels of culture that may be invisible or difficult to measure. Assessing culture helps understand the ways it can empower people to experiment and take risks, and the importance this has for innovation. And where a culture is deemed to be limiting innovation, action can be taken to motivate the right culture and steer the organisation towards a better chance of success.
Futher Reading
- Paper: Hogan & Coote (2014) Organizational Culture, Innovation and Performance (via www.researchgate.net)
- Book: Johnson & Scholes (1999) Exploring Corporate Strategy: Text and Cases
- Article: Understanding Organisational Culture - Checklist by CMI (via www.managers.org.uk)
- Article: The Cultural Web (via www.mindtools.com)
- Paper: Mossop et al. (2013) Analysing the hidden curriculum: use of a cultural web (via www.ncbi.nlm.nih.gov)
- Book: Bruch & Vogel (2011) Fully Charged: How Great Leaders Boost Their Organization’s Energy and Ignite High Performance (via reading.ac.uk)
- Webinar: Bruch (2012) Fully Charged: How Great Leaders Boost Their Organization’s Energy and Ignite High Performance (via hbr.org)
- Article: Pisano (2019) The Hard Truth About Innovative Cultures (via hbr.org)
Some links above may require payment or login. We are not endorsing them or receiving any payment for mentioning them. They are provided as is. Often free versions of papers are available and we would encourage you to investigate.
Recording date: 12 Aug 2022
Thanks for joining us in the DataCafé. You can follow us on twitter @DataCafePodcast and feel free to contact us about anything you've heard here or think would be an interesting topic in the future.
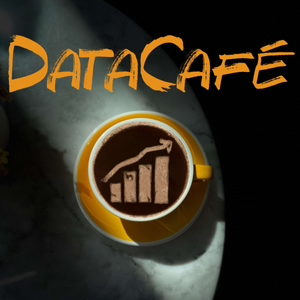
Series 2 Introduction
DataCafé
03/14/22 • 5 min
Looks like we might be about to have a new Series of DataCafé!
Recording date: 15 Feb 2022
Intro music by Music 4 Video Library (Patreon supporter)
Thanks for joining us in the DataCafé. You can follow us on twitter @DataCafePodcast and feel free to contact us about anything you've heard here or think would be an interesting topic in the future.
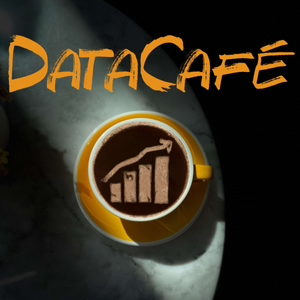
02/22/21 • 35 min
Have you ever come home from the supermarket to discover one of the apples you bought is rotten? It's likely your trust for that grocer was diminished, or you might stop buying that particular brand of apples altogether.
In this episode, we discuss how the quality controls in a production line need to use smart sampling methods in order to avoid sending bad products to the customer, which could ruin the reputation of both the brand and seller.
To do this we describe a thought experiment called Apple Tasting. This allows us to demonstrate the concepts of regret and reward in a sampling process, giving rise to the use of Contextual Bandit Algorithms. Contextual Bandits come from the field of Reinforcement Learning which is a form of Machine Learning where an agent performs an action and tries to maximise the cumulative reward from its environment over time. Standard bandit algorithms simply choose between a number of actions and measure the reward in order to determine the average reward of each action. But a Contextual Bandit also uses information from its environment to inform both the likely reward and regret of subsequent actions. This is particularly useful in personalised product recommendation engines where the bandit algorithm is given some contextual information about the user.
Back to Apple Tasting and product quality control. The contextual bandit in this scenario, consumes a signal from a benign test that is indicative, but not conclusive, of there being a fault and then makes the decision to perform a more in-depth test or not. So the answer for when you should discard or test your product depends on the relative costs of making the right decision (reward) or wrong decision (regret) and how your experience of the environment affected these in the past.
We speak with Prof. David Leslie about how this logic can be applied to any manufacturing pipeline where there is a downside risk of not quality checking the product but a cost in a false positive detection of a bad product.
Other areas of application include:
- Anomalous behaviour in a jet engine e.g. low fuel efficiency, which could be nothing or could be serious, so it might be worth taking the plane in for repair.
- Changepoints in network data time series - does it mean there’s a fault on the line or does it mean the next series of The Queen’s Gambit has just been released? Should we send an engineer out?
With interview guest David Leslie, Professor of Statistical Learning in the Department of Mathematics and Statistics at Lancaster University.
Further Reading
- Publication list for Prof. David Leslie (http://bitly.ws/bQ4a via Lancaster University)
- Paper on "Selecting Multiple Web Adverts - a Contextual Multi-armed Bandit with State Uncertainty" in Journal of the ORS (http://bitly.ws/bQ3X via Lancaster University)
- Paper on "Apple tasting" (http://bitly.ws/bQeW via ScienceDirect)
- Paper by Google Inc. on "AutoML for Contextual Bandits" (https://arxiv.org/abs/1909.03212 via arXiv)
Thanks for joining us in the DataCafé. You can follow us on twitter @DataCafePodcast and feel free to contact us about anything you've heard here or think would be an interesting topic in the future.
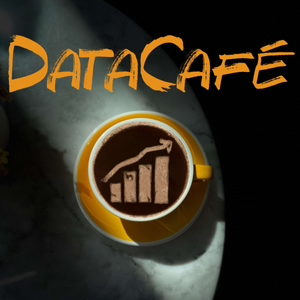
Optimising the Future
DataCafé
01/04/21 • 35 min
As we look ahead to a new year, and reflect on the last, we consider how data science can be used to optimise the future. But to what degree can we trust past experiences and observations, essentially relying on historical data to predict the future? And with what level of accuracy?
In this episode of the DataCafé we ask: how can we optimise our predictions of future scenarios to maximise the benefit we can obtain from them while minimising the risk of unknowns?
Data Science is made up of many diverse technical disciplines that can help to answer these questions. Two among them are mathematical optimisation and machine learning. We explore how these two fascinating areas interact and how they can both help to turbo charge the other's cutting edge in the future.
We speak with Dimitrios Letsios from King's College London about his work in optimisation and what he sees as exciting new developments in the field by working together with the field of machine learning.
With interview guest Dr. Dimitrios Letsios, lecturer (assistant professor) in the Department of Informatics at King's College London and a member of the Algorithms and Data Analysis Group.
Further reading
- Dimirios Letsios' publication list (https://bit.ly/35vHirH via King's College London)
- Paper on taking into account uncertainty in an optimisation model: Approximating Bounded Job Start Scheduling with Application in Royal Mail Deliveries under Uncertainty (https://bit.ly/3pLHICV via King's College London)
- Paper on lexicographic optimisation: Exact Lexicographic Scheduling and Approximate Rescheduling (https://bit.ly/3rS8Xxk via arXiv)
- Paper on combination of AI and Optimisation: Argumentation for Explainable Scheduling (https://bit.ly/3oobgGF via AAAI Conference on Artificial Intelligence)
Some links above may require payment or login. We are not endorsing them or receiving any payment for mentioning them. They are provided as is. Often free versions of papers are available and we would encourage you to investigate.
Recording date: 23 October 2020
Interview date: 21 February 2020
Intro music by Music 4 Video Library (Patreon supporter)
Thanks for joining us in the DataCafé. You can follow us on twitter @DataCafePodcast and feel free to contact us about anything you've heard here or think would be an interesting topic in the future.
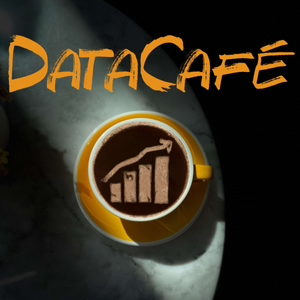
07/13/20 • 31 min
How can we spot a change in a jet engine vibration that might mean it’s about to fail catastrophically? How can a service forecast adapt to unexpected changes brought about by a pandemic? How might we spot an increase in rate of change of pollution in the atmosphere? The answer to all these questions is changepoints, or rather changepoint detection.
Common to all these systems is a set of ordered data, usually a time series of observations or measurements that may be noisy but have some underlying pattern. As the world changes, so those changes might lead to dramatic changes in the measurements and a disruption of the usual pattern. Unless these forecasts or failure-detection systems are updated quickly to take account of a change in measurement data, they will likely produce erroneous or unpredictable results.
Changepoints have many important applications in areas such as:
- Climatology
- Genetic sequencing
- Finance
- Medical imaging
- Forecasting in industry
We speak to statistician Dr. Rebecca Killick from Lancaster University about her work in changepoint detection and how it is a critical part of the statistical toolkit for analysing time series and other ordered data sets. In particular:
- In forecasting where most methods tend to work on the basis of extrapolating trends, it is essential to know if a changepoint has occurred so that a refreshed model calculation can be started.
- If there is a change in the underlying dynamics of a system that causes a complex change in the observed output then this can often be detected with a changepoint. This might be indicative of a mechanical failure or impending change in operation or an unobserved event buried deep in a difficult-to-measure environment, like a nuclear reactor.
With interview guest Dr. Rebecca Killick, Associate Professor of Statistics at Lancaster University.
Further reading
- Rebecca Killick’s publications (via Lancaster University)
- Changepoints Overview Paper: changepoint: An R package for changepoint analysis (pdf via Journal of Statistical Software)
- R Package: changepoint: Methods for Changepoint Detection (R package via CRAN library)
- PELT algorithm paper: Optimal detection of changepoints with a linear computational cost (pdf via arXiv)
- Paper: Distinguishing Trends and Shifts from Memory in Climate Data (paper via American Meteorological Society)
- R Package: EnvCpt: Detection of Structural Changes in Climate and Environment Time Series (R package via CRAN library)
Some links above may require payment or login. We are not endorsing them or receiving any payment for mentioning them. They are provided as is. Often free versions of papers are available and we would encourage you to investigate.
Recording date: 10 June 2020
Interview date: 5 June 2020
Thanks for joining us in the DataCafé. You can follow us on twitter @DataCafePodcast and feel free to contact us about anything you've heard here or think would be an interesting topic in the future.
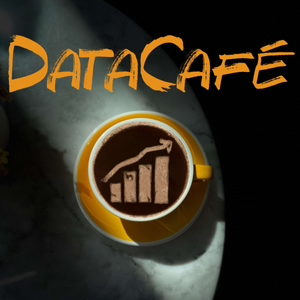
06/01/20 • 30 min
How do big grocery retailers maintain product availability for their customers day after day while minimising food wastage and storage costs? The answer is Inventory Optimisation, the science of maintaining sufficient stock levels of a set of products so that customers see an appropriate level of availability when they walk into your store.
The trade-off
It’s hard because it often costs money to maintain a large inventory of products, because of space that is given over to bulky stock as the cost of buying a large amount of potentially expensive items without getting a return on that investment until sale. A long lead time from stocking to use or sale means no value is extracted from those items and that can have cashflow implications for a company while obviously minimising risk of so-called stockout.
Wastage
A significant further complication comes from food and grocery retail where the items being stocked are themselves perishable with varying expiry dates. Further significant costs are incurred if the product expires while still being stocked. This leads to huge food waste problems around the world which in turn have a significant carbon and environmental impact on the direct supply chain for the retailer.
With interview guest Dr. Anna Lena-Sachs, Lecturer in Predictive Analytics at the Department of Management Sciences, Lancaster University.
https://www.lancaster.ac.uk/lums/people/anna-lena-sachs
Further Reading
- Anna-Lena Sach's list of papers (via University of Lancaster)
- Book: Retail Analytics: Integrated Forecasting and Inventory Management for Perishable Products in Retailing (contents via Springer)
- Paper: The data-driven newsvendor with censored demand observations (summary via ScienceDirect)
- Paper: A stochastic model for joint spare parts inventory and planned maintenance optimisation (summary via ScienceDirect)
Some links above may require payment or login. We are not endorsing them or receiving any payment for mentioning them. They are provided as is. Often free versions of papers are available and we would encourage you to investigate.
Recording date: 29 Jan. 2020
Interview date: 24 Jan. 2020
Thanks for joining us in the DataCafé. You can follow us on twitter @DataCafePodcast and feel free to contact us about anything you've heard here or think would be an interesting topic in the future.
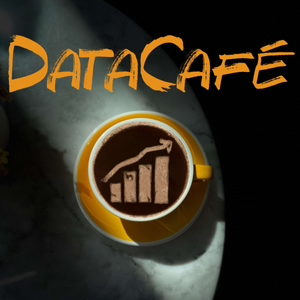
06/01/20 • 39 min
How can we generate efficient routes for a large fleets of vehicles that have to make many thousands of deliveries a day while taking into account breaks, shift patterns and traffic conditions? Now let's make those vehicles electric and we need to take into account vehicle battery charge level, recharging station locations and anticipated energy efficiency. It's a challenging problem!
Vehicle Routing Problem (VRP) is the optimisation problem that describes all manifested delivery operations. It provides an optimal way of sorting deliveries onto multiple vehicles and providing each vehicle with an optimal sequence for delivery. The problem is NP-hard and suffers from a combinatorial explosion of solutions as the number of vehicles and deliveries increases.
We speak to Merve Keskin of the Warwick Business School about extending VRP as an area of optimisation to electric vehicles with a number of interesting developments:
- Keeping track of battery level en route and inserting possible stops at nearby compatible recharging points
- Allowing delay for possible queues at recharge points
- Potential for inflight negotiation on charge point booking so as to minimise contention for resource and wait times on long journeys.
With interview guest Dr. Merve Keskin, Warwick Business School and KTP fellow.
https://www.wbs.ac.uk/about/person/merve-keskin/
Further reading
- Merve Keskin's publication list (via Google Scholar)
- Paper: A matheuristic method for the electric vehicle routing problem with time windows and fast chargers (summary via ScienceDirect)
- Paper: Electric vehicle routing problem with time-dependent waiting times at recharging stations (pdf via researchgate)
- Paper: Partial recharge strategies for the electric vehicle routing problem with time windows (summary via ScienceDirect)
- Overview: A concise guide to existing and emerging vehicle routing problem variants (pdf via arXiv)
Some links above may require payment or login. We are not endorsing them or receiving any payment for mentioning them. They are provided as is. Often free versions of papers are available and we would encourage you to investigate.
Recording date: 28 Feb. 2020
Interview date: 12 Feb. 2020
Thanks for joining us in the DataCafé. You can follow us on twitter @DataCafePodcast and feel free to contact us about anything you've heard here or think would be an interesting topic in the future.
![DataCafé - [Bite] Wordle: Winning against the algorithm](https://storage.googleapis.com/goodpods-images-bucket/episode_images/642929816cfd39f9681cadadc49df0f26f8cf1423b4eb741a37f7b1427a2b0af.avif)
03/14/22 • 11 min
The grey, green and yellow squares taking over social media in the last few weeks is an example of the fascinating field of study known as Game Theory. In this bite episode of DataCafé we talk casually about Wordle - the internet phenomenon currently challenging players to guess a new five letter word each day.
Six guesses inform players what letters they have gotten right and if they are in the right place. It’s a lovely example of the different ways people approach game strategy through their choice of guesses and ways to use the information presented within the game.
Wordles
- Wordle - the original
- Absurdle - it's Wordle but it fights you!
- Nerdle - Maths Wordle
- Quordle - when one Wordle is not enough!
- Foclach - Irish Wordle
Analysis
- Statistical analysis of hard-mode Wordle with Matlab by Matt Tearle (youtube)
- The science behind Wordle by Ido Frizler (medium.com)
Some links above may require payment or login. We are not endorsing them or receiving any payment for mentioning them. They are provided as is. Often free versions of papers are available and we would encourage you to investigate.
Recording date: 15 Feb 2022
Intro music by Music 4 Video Library (Patreon supporter)
Thanks for joining us in the DataCafé. You can follow us on twitter @DataCafePodcast and feel free to contact us about anything you've heard here or think would be an interesting topic in the future.
Show more best episodes

Show more best episodes
FAQ
How many episodes does DataCafé have?
DataCafé currently has 26 episodes available.
What topics does DataCafé cover?
The podcast is about Podcasts, Technology, Science, Data Analytics, Artificial Intelligence, Data Science and Machine Learning.
What is the most popular episode on DataCafé?
The episode title 'Scaling the Internet' is the most popular.
What is the average episode length on DataCafé?
The average episode length on DataCafé is 32 minutes.
How often are episodes of DataCafé released?
Episodes of DataCafé are typically released every 28 days, 14 hours.
When was the first episode of DataCafé?
The first episode of DataCafé was released on Jun 1, 2020.
Show more FAQ

Show more FAQ