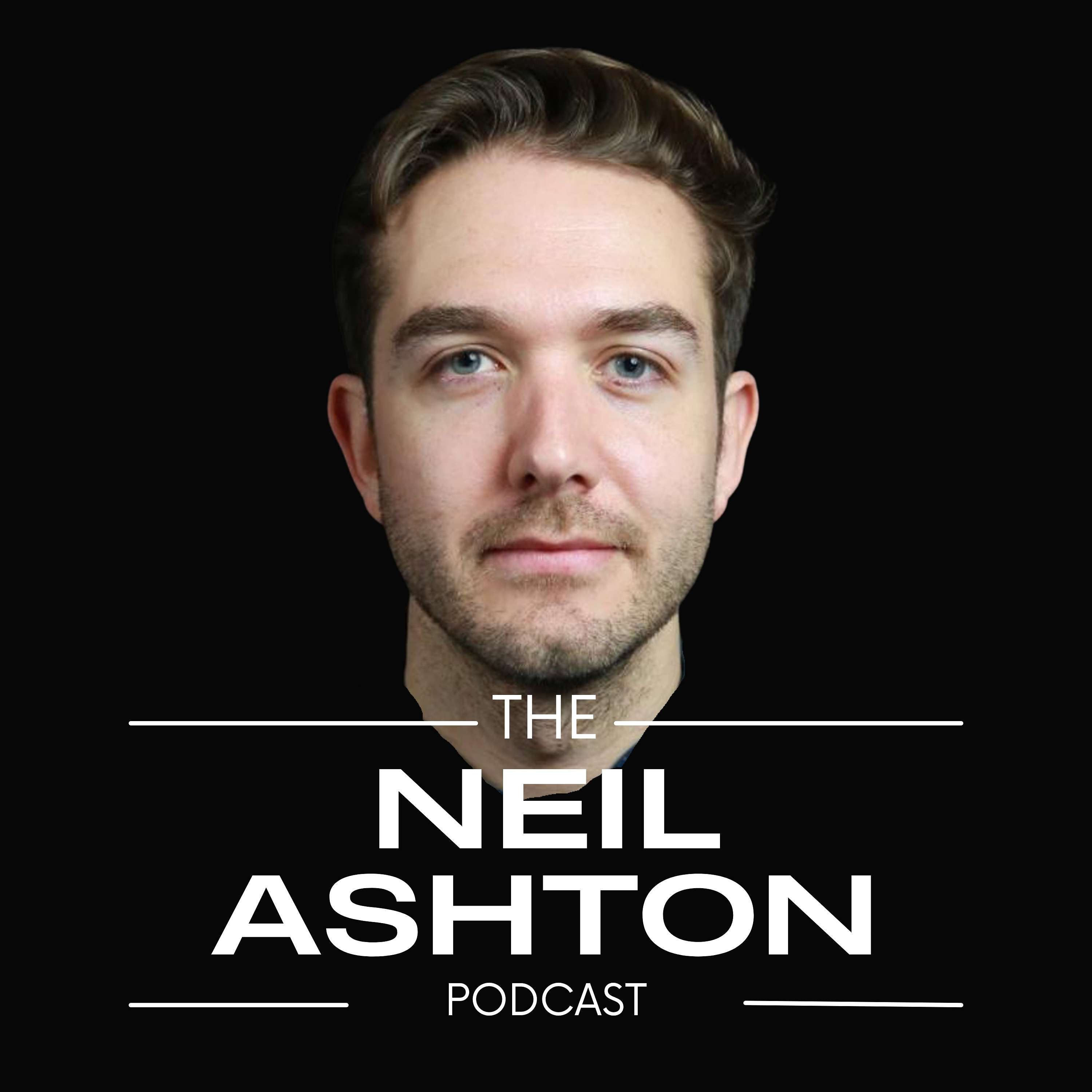
S1, EP12 - Prof Karthik Duraisamy - Scientific Foundational Models
07/23/24 • 92 min
Prof. Karthik Duraisamy is a Professor at the University of Michigan, the Director of the Michigan Institute for Computational Discovery and Engineering (MICDE) and the founder of the startup Geminus.AI. In this episode, we discusses AI4Science, with a particular focus on fluid dynamics and computational fluid dynamics. Prof. Duraisamy talks about the progress and challenges of using machine learning in turbulence modeling and the potential of surrogate models (both data-driven and physics-informed neural networks). He also explores the concept of foundational models for science and the role of data and physics in AI applications. The discussion highlights the importance of using machine learning as a tool in the scientific process and the potential benefits of large language models in scientific discovery. We also discuss the need for collaboration between academia, tech companies, and startups to achieve the vision of a new platform for scientific discovery. Prof. Duraisamy predicts that in the next few years, there may be major advancements in foundation models for science however he cautions against unrealistic expectations and emphasizes the importance of understanding the limitations of AI.
Links:
Summer school tutorials https://github.com/scifm/summer-school-2024 (scroll down for links to specific tutorials)
SciFM24 recordings : https://micde.umich.edu/news-events/annual-symposia/2024-symposium/
SciFM24 Summary : https://drive.google.com/file/d/1eC2HJdpfyZZ42RaT9KakcuACEo4nqAsJ/view
Trillion parameter consortium : https://tpc.dev
Turbulence Modelling in the age of data: https://www.annualreviews.org/content/journals/10.1146/annurev-fluid-010518-040547
LinkedIn: https://www.linkedin.com/showcase/micde/
Chapters
00:00 Introduction
09:41 Turbulence Modeling and Machine Learning
21:30 Surrogate Models and Physics-Informed Neural Networks
28:42 Foundational Models for Science
35:23 The Power of Large Language Models
47:43 Tools for Foundation Models
48:39 Interfacing with Specialized Agents
53:31 The Importance of Collaboration
58:57 The Role of Agents and Solvers
01:08:26 Balancing AI and Existing Expertise
01:21:28 Predicting the Future of AI in Fluid Dynamics
01:23:18 Closing Gaps in Turbulence Modeling
01:25:42 Achieving Productivity Benefits with Existing Tools
Takeaways
-Machine learning is a valuable tool in the development of turbulence modeling and other scientific applications.
-Data-driven modeling can provide additional insights and improve the accuracy of scientific models.
-Physics-informed neural networks have potential in solving inverse problems but may not be as effective in solving complex PDEs.
-Foundational models for science can benefit from a combination of data-driven approaches and physics-based knowledge.
-Large language models have the potential to assist in scientific discovery and provide valuable insights in various scientific domains. Having a strong foundation in the domain of study is crucial before applying AI techniques.
-Collaboration between academia, tech companies, and startups is necessary to achieve the vision of a new platform for scientific discovery.
-Understanding the limitations of AI and managing expectations is important.
-AI can be a valuable tool for productivity gains and scientific assistance, but it will not replace human expertise.
Keywords
#computationalfluiddynamics , #ailearning #largelanguagemodels , #cfd , #supercomputing , #fluiddynamics
Prof. Karthik Duraisamy is a Professor at the University of Michigan, the Director of the Michigan Institute for Computational Discovery and Engineering (MICDE) and the founder of the startup Geminus.AI. In this episode, we discusses AI4Science, with a particular focus on fluid dynamics and computational fluid dynamics. Prof. Duraisamy talks about the progress and challenges of using machine learning in turbulence modeling and the potential of surrogate models (both data-driven and physics-informed neural networks). He also explores the concept of foundational models for science and the role of data and physics in AI applications. The discussion highlights the importance of using machine learning as a tool in the scientific process and the potential benefits of large language models in scientific discovery. We also discuss the need for collaboration between academia, tech companies, and startups to achieve the vision of a new platform for scientific discovery. Prof. Duraisamy predicts that in the next few years, there may be major advancements in foundation models for science however he cautions against unrealistic expectations and emphasizes the importance of understanding the limitations of AI.
Links:
Summer school tutorials https://github.com/scifm/summer-school-2024 (scroll down for links to specific tutorials)
SciFM24 recordings : https://micde.umich.edu/news-events/annual-symposia/2024-symposium/
SciFM24 Summary : https://drive.google.com/file/d/1eC2HJdpfyZZ42RaT9KakcuACEo4nqAsJ/view
Trillion parameter consortium : https://tpc.dev
Turbulence Modelling in the age of data: https://www.annualreviews.org/content/journals/10.1146/annurev-fluid-010518-040547
LinkedIn: https://www.linkedin.com/showcase/micde/
Chapters
00:00 Introduction
09:41 Turbulence Modeling and Machine Learning
21:30 Surrogate Models and Physics-Informed Neural Networks
28:42 Foundational Models for Science
35:23 The Power of Large Language Models
47:43 Tools for Foundation Models
48:39 Interfacing with Specialized Agents
53:31 The Importance of Collaboration
58:57 The Role of Agents and Solvers
01:08:26 Balancing AI and Existing Expertise
01:21:28 Predicting the Future of AI in Fluid Dynamics
01:23:18 Closing Gaps in Turbulence Modeling
01:25:42 Achieving Productivity Benefits with Existing Tools
Takeaways
-Machine learning is a valuable tool in the development of turbulence modeling and other scientific applications.
-Data-driven modeling can provide additional insights and improve the accuracy of scientific models.
-Physics-informed neural networks have potential in solving inverse problems but may not be as effective in solving complex PDEs.
-Foundational models for science can benefit from a combination of data-driven approaches and physics-based knowledge.
-Large language models have the potential to assist in scientific discovery and provide valuable insights in various scientific domains. Having a strong foundation in the domain of study is crucial before applying AI techniques.
-Collaboration between academia, tech companies, and startups is necessary to achieve the vision of a new platform for scientific discovery.
-Understanding the limitations of AI and managing expectations is important.
-AI can be a valuable tool for productivity gains and scientific assistance, but it will not replace human expertise.
Keywords
#computationalfluiddynamics , #ailearning #largelanguagemodels , #cfd , #supercomputing , #fluiddynamics
Previous Episode
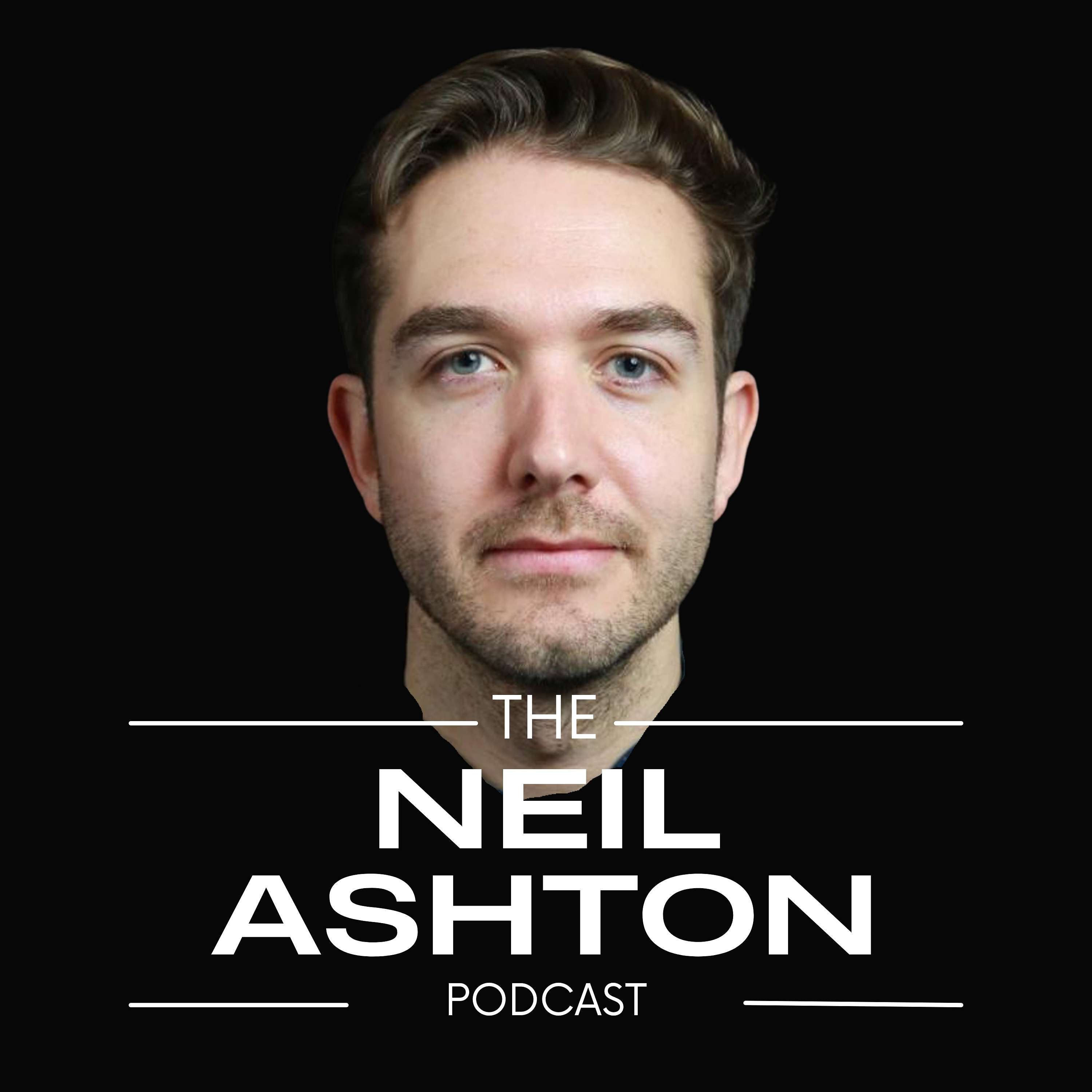
S1, EP11 - Prof. Max Welling - Machine Learning Pioneer & AI4Science Visionary
In this episode, Neil interviews Professor Max Welling, one of the foremost experts in Machine Learning about AI4Science: the use of machine learning and AI to solve challenges in various scientific disciplines. They discuss and debate between data-driven and physics-driven approaches, the potential for foundational models, the importance of open sourcing models and data, the challenges of data sharing in science, and the ethical considerations of releasing powerful models. The conversation covers the role of academia, industry, and startups in driving innovation, with a focus on the field of AI. Professor Welling discusses the advantages and limitations of each sector and shares his experience in academia, big tech companies, and startups. The conversation then shifts to Professor Wellings new company; CuspAI, which focuses on material discovery for carbon capture using metal organic frameworks and machine learning. Prof. Welling provides insights into the potential applications of this technology and the importance of addressing sustainability challenges. The conversation concludes with a discussion on career advice and the future of AI for science.
Links
CuspAI : https://www.cusp.ai
University website: https://staff.fnwi.uva.nl/m.welling/
Google scholar: https://scholar.google.com/citations?user=8200InoAAAAJ&hl=en
AI4Science NeurIPS 2023 workshop: https://neurips.cc/virtual/2023/workshop/66548
AI4Science NeurIPS 2022 workshop: https://nips.cc/virtual/2022/workshop/50019
Aurora paper: https://arxiv.org/abs/2405.13063
Chapters
00:00 Introduction to the Neil Ashton Podcast
00:39 Guest Introduction: Professor Max Welling
11:12 Data-Driven vs. Physics-Driven Approaches in Machine Learning for Science
17:00 Foundational models for science
23:08 Discussion around Open-Sourcing Models and Data
29:26 Ethical Considerations in Releasing Powerful Models for Public Use
33:14 Collaboration and Shared Resources in Addressing Global Challenges
34:07 The Role of Academia, Industry, and Startups
43:27 Material Discovery for Carbon Capture
52:02 Career Advice for Early-stage Researchers
01:01:07 The Future of AI for Science and Sustainability
Keywords
AI for science, machine learning, data-driven approaches, physics-driven approaches, foundational models, open sourcing, data sharing, ethical considerations, blockchain technology, academia, industry, startups, AI, material discovery, carbon capture, metal organic frameworks, machine learning, sustainability, career advice, future of AI for science
Next Episode
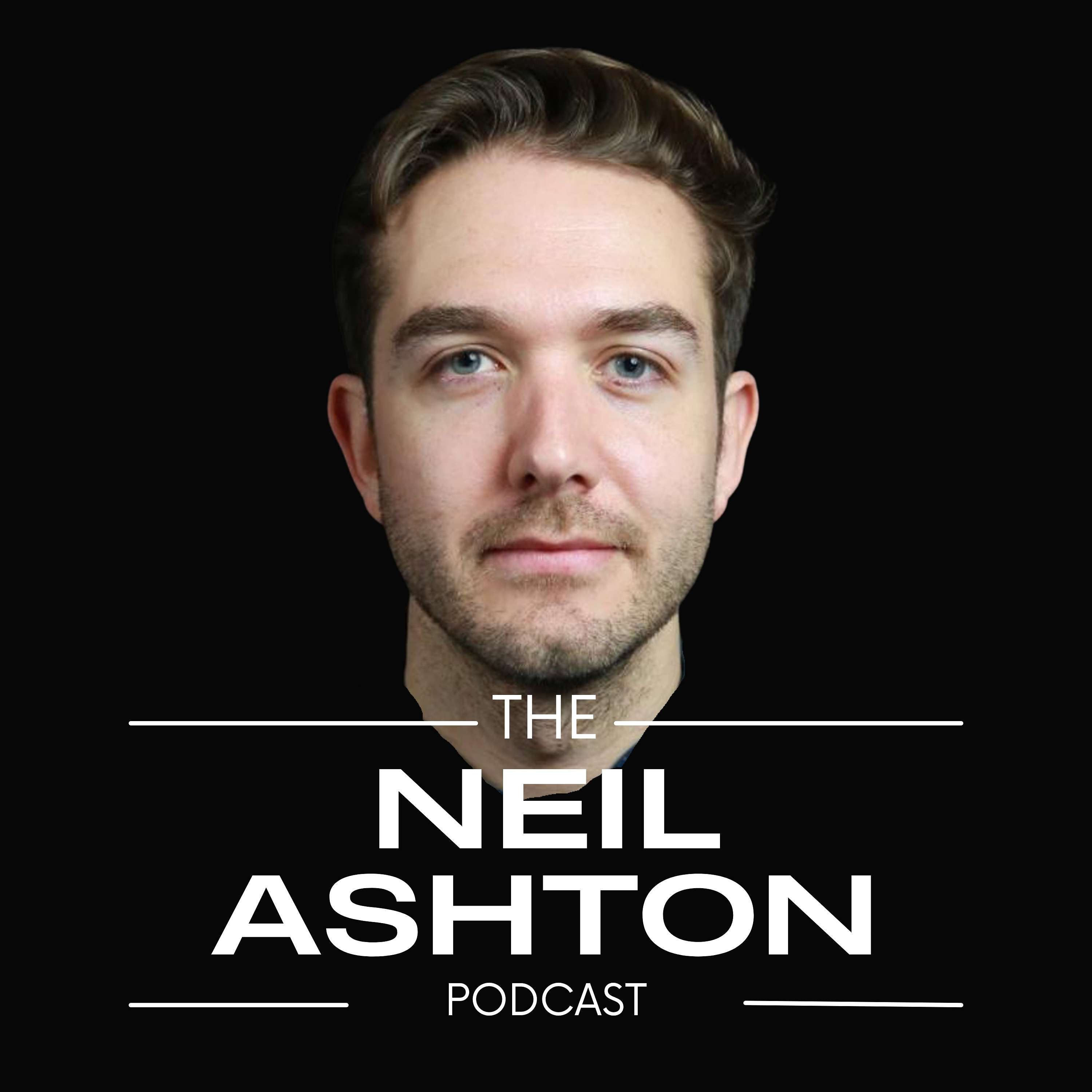
S1, EP13 - Prof. Anima Anandkumar - The future of AI+Science
Professor Anima Anandkumar is one of the worlds leading scientists in the field of AI & ML with more than 30k citations, a h-index of 80 and numerous landmark papers such as FourCastNet, which got world-wide coverage for demonstrating how AI can be used to speed up weather prediction. She is the Bren Professor at Caltech, leading a large team of PhD students and post-docs in her AI+Science lab, and has had extensive experience in industry, previously being the Senior Director of AI Resarch at Nvidia.
In this episode I speak to her about her background in academia and industry, her journey into machine learning, and the importance of AI for science. We discuss the integration of AI and scientific research, the potential of AI in weather modeling, and the challenges of applying AI to other areas of science. Prof Anandkumar shares examples of successful AI applications in science and explains the concept of AI + science. We also touch on the skepticism surrounding machine learning in physics and the need for data-driven approaches. The conversation explores the potential of AI in the field of science and engineering, specifically in the context of physics-based simulations. Prof. Anandkumar discusses the concept of neural operators, highlights the advantages of neural operators, such as their ability to handle multiple domains and resolutions, and their potential to revolutionize traditional simulation methods. Prof. Anandkumar also emphasizes the importance of integrating AI with scientific knowledge and the need for interdisciplinary collaboration between ML specialists and domain experts. She also emphasizes the importance of integrating AI with traditional numerical solvers and the need for interdisciplinary collaboration between ML specialists and domain experts. Finall she provides advice for PhD students and highlights the significance of attending smaller workshops and conferences to stay updated on emerging ideas in the field.
Links:
LinkedIn: https://www.linkedin.com/in/anima-anandkumar/
Ted Video: https://www.youtube.com/watch?v=6bl5XZ8kOzI
FourCastNet: https://arxiv.org/abs/2202.11214
Google Scholar: https://scholar.google.com/citations?hl=en&user=bEcLezcAAAAJ
Lab page: http://tensorlab.cms.caltech.edu/users/anima/
Takeaways
- Anima's background includes both academia and industry, and she sees value in bridging the gap between the two.
- AI for science is the integration of AI and scientific research, with the goal of enhancing and accelerating scientific developments.
- AI has shown promise in weather modeling, with AI-based weather models outperforming traditional numerical models in terms of speed and accuracy.
- The skepticism surrounding machine learning in physics can be addressed by verifying the accuracy of AI models against known physics principles.
- Applying AI to other areas of science, such as aircraft design and fluid dynamics, presents challenges in terms of data availability and computational cost. Neural operators have the potential to revolutionize traditional simulation methods in science and engineering.
- Integrating AI with scientific knowledge is crucial for the development of effective AI models in the field of physics-based simulations.
- Interdisciplinary collaboration between ML specialists and domain experts is essential for advancing AI in science and engineering.
- The future of AI in science and engineering lies in the integration of various modalities, such as text, observational data, and physical understanding.
Chapters
00:00 Introduction and Overview
04:29 Professor Anima Anandkumar's Career Journey
09:14 Moving to the US for PhD and Transitioning to Industry
13:00 Academia vs Industry: Personal Choices and Opportunities
17:49 Defining AI for Science and Its Importance
22:05 AI's Promise in Enhancing Scientific Discovery
28:18 The Success of AI-Based Wea
If you like this episode you’ll love
Episode Comments
Generate a badge
Get a badge for your website that links back to this episode
<a href="https://goodpods.com/podcasts/the-neil-ashton-podcast-467936/s1-ep12-prof-karthik-duraisamy-scientific-foundational-models-63758240"> <img src="https://storage.googleapis.com/goodpods-images-bucket/badges/generic-badge-1.svg" alt="listen to s1, ep12 - prof karthik duraisamy - scientific foundational models on goodpods" style="width: 225px" /> </a>
Copy