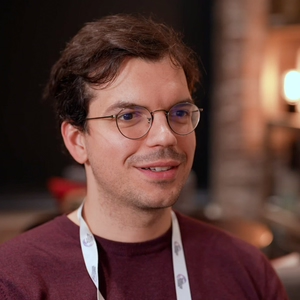
Prof. Randall Balestriero - LLMs without pretraining and SSL
04/23/25 • 34 min
Randall Balestriero joins the show to discuss some counterintuitive findings in AI. He shares research showing that huge language models, even when started from scratch (randomly initialized) without massive pre-training, can learn specific tasks like sentiment analysis surprisingly well, train stably, and avoid severe overfitting, sometimes matching the performance of costly pre-trained models. This raises questions about when giant pre-training efforts are truly worth it.
He also talks about how self-supervised learning (where models learn from data structure itself) and traditional supervised learning (using labeled data) are fundamentally similar, allowing researchers to apply decades of supervised learning theory to improve newer self-supervised methods.
Finally, Randall touches on fairness in AI models used for Earth data (like climate prediction), revealing that these models can be biased, performing poorly in specific locations like islands or coastlines even if they seem accurate overall, which has important implications for policy decisions based on this data.
SPONSOR MESSAGES:
***
Tufa AI Labs is a brand new research lab in Zurich started by Benjamin Crouzier focussed on o-series style reasoning and AGI. They are hiring a Chief Engineer and ML engineers. Events in Zurich.
Goto https://tufalabs.ai/
***
TRANSCRIPT + SHOWNOTES:
https://www.dropbox.com/scl/fi/n7yev71nsjso71jyjz1fy/RANDALLNEURIPS.pdf?rlkey=0dn4injp1sc4ts8njwf3wfmxv&dl=0
TOC:
1. Model Training Efficiency and Scale
[00:00:00] 1.1 Training Stability of Large Models on Small Datasets
[00:04:09] 1.2 Pre-training vs Random Initialization Performance Comparison
[00:07:58] 1.3 Task-Specific Models vs General LLMs Efficiency
2. Learning Paradigms and Data Distribution
[00:10:35] 2.1 Fair Language Model Paradox and Token Frequency Issues
[00:12:02] 2.2 Pre-training vs Single-task Learning Spectrum
[00:16:04] 2.3 Theoretical Equivalence of Supervised and Self-supervised Learning
[00:19:40] 2.4 Self-Supervised Learning and Supervised Learning Relationships
[00:21:25] 2.5 SSL Objectives and Heavy-tailed Data Distribution Challenges
3. Geographic Representation in ML Systems
[00:25:20] 3.1 Geographic Bias in Earth Data Models and Neural Representations
[00:28:10] 3.2 Mathematical Limitations and Model Improvements
[00:30:24] 3.3 Data Quality and Geographic Bias in ML Datasets
REFS:
[00:01:40] Research on training large language models from scratch on small datasets, Randall Balestriero et al.
https://openreview.net/forum?id=wYGBWOjq1Q
[00:10:35] The Fair Language Model Paradox (2024), Andrea Pinto, Tomer Galanti, Randall Balestriero
https://arxiv.org/abs/2410.11985
[00:12:20] Muppet: Massive Multi-task Representations with Pre-Finetuning (2021), Armen Aghajanyan et al.
https://arxiv.org/abs/2101.11038
[00:14:30] Dissociating language and thought in large language models (2023), Kyle Mahowald et al.
https://arxiv.org/abs/2301.06627
[00:16:05] The Birth of Self-Supervised Learning: A Supervised Theory, Randall Balestriero et al.
https://openreview.net/forum?id=NhYAjAAdQT
[00:21:25] VICReg: Variance-Invariance-Covariance Regularization for Self-Supervised Learning, Adrien Bardes, Jean Ponce, Yann LeCun
https://arxiv.org/abs/2105.04906
[00:25:20] No Location Left Behind: Measuring and Improving the Fairness of Implicit Representations for Earth Data (2025), Daniel Cai, Randall Balestriero, et al.
https://arxiv.org/abs/2502.06831
[00:33:45] Mark Ibrahim et al.'s work on geographic bias in computer vision datasets, Mark Ibrahim
https://arxiv.org/pdf/2304.12210
Randall Balestriero joins the show to discuss some counterintuitive findings in AI. He shares research showing that huge language models, even when started from scratch (randomly initialized) without massive pre-training, can learn specific tasks like sentiment analysis surprisingly well, train stably, and avoid severe overfitting, sometimes matching the performance of costly pre-trained models. This raises questions about when giant pre-training efforts are truly worth it.
He also talks about how self-supervised learning (where models learn from data structure itself) and traditional supervised learning (using labeled data) are fundamentally similar, allowing researchers to apply decades of supervised learning theory to improve newer self-supervised methods.
Finally, Randall touches on fairness in AI models used for Earth data (like climate prediction), revealing that these models can be biased, performing poorly in specific locations like islands or coastlines even if they seem accurate overall, which has important implications for policy decisions based on this data.
SPONSOR MESSAGES:
***
Tufa AI Labs is a brand new research lab in Zurich started by Benjamin Crouzier focussed on o-series style reasoning and AGI. They are hiring a Chief Engineer and ML engineers. Events in Zurich.
Goto https://tufalabs.ai/
***
TRANSCRIPT + SHOWNOTES:
https://www.dropbox.com/scl/fi/n7yev71nsjso71jyjz1fy/RANDALLNEURIPS.pdf?rlkey=0dn4injp1sc4ts8njwf3wfmxv&dl=0
TOC:
1. Model Training Efficiency and Scale
[00:00:00] 1.1 Training Stability of Large Models on Small Datasets
[00:04:09] 1.2 Pre-training vs Random Initialization Performance Comparison
[00:07:58] 1.3 Task-Specific Models vs General LLMs Efficiency
2. Learning Paradigms and Data Distribution
[00:10:35] 2.1 Fair Language Model Paradox and Token Frequency Issues
[00:12:02] 2.2 Pre-training vs Single-task Learning Spectrum
[00:16:04] 2.3 Theoretical Equivalence of Supervised and Self-supervised Learning
[00:19:40] 2.4 Self-Supervised Learning and Supervised Learning Relationships
[00:21:25] 2.5 SSL Objectives and Heavy-tailed Data Distribution Challenges
3. Geographic Representation in ML Systems
[00:25:20] 3.1 Geographic Bias in Earth Data Models and Neural Representations
[00:28:10] 3.2 Mathematical Limitations and Model Improvements
[00:30:24] 3.3 Data Quality and Geographic Bias in ML Datasets
REFS:
[00:01:40] Research on training large language models from scratch on small datasets, Randall Balestriero et al.
https://openreview.net/forum?id=wYGBWOjq1Q
[00:10:35] The Fair Language Model Paradox (2024), Andrea Pinto, Tomer Galanti, Randall Balestriero
https://arxiv.org/abs/2410.11985
[00:12:20] Muppet: Massive Multi-task Representations with Pre-Finetuning (2021), Armen Aghajanyan et al.
https://arxiv.org/abs/2101.11038
[00:14:30] Dissociating language and thought in large language models (2023), Kyle Mahowald et al.
https://arxiv.org/abs/2301.06627
[00:16:05] The Birth of Self-Supervised Learning: A Supervised Theory, Randall Balestriero et al.
https://openreview.net/forum?id=NhYAjAAdQT
[00:21:25] VICReg: Variance-Invariance-Covariance Regularization for Self-Supervised Learning, Adrien Bardes, Jean Ponce, Yann LeCun
https://arxiv.org/abs/2105.04906
[00:25:20] No Location Left Behind: Measuring and Improving the Fairness of Implicit Representations for Earth Data (2025), Daniel Cai, Randall Balestriero, et al.
https://arxiv.org/abs/2502.06831
[00:33:45] Mark Ibrahim et al.'s work on geographic bias in computer vision datasets, Mark Ibrahim
https://arxiv.org/pdf/2304.12210
Previous Episode
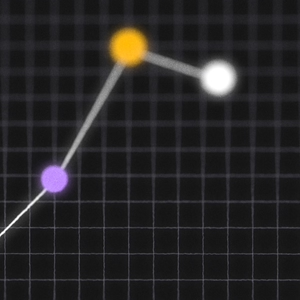
How Machines Learn to Ignore the Noise (Kevin Ellis + Zenna Tavares)
Prof. Kevin Ellis and Dr. Zenna Tavares talk about making AI smarter, like humans. They want AI to learn from just a little bit of information by actively trying things out, not just by looking at tons of data.
They discuss two main ways AI can "think": one way is like following specific rules or steps (like a computer program), and the other is more intuitive, like guessing based on patterns (like modern AI often does). They found combining both methods works well for solving complex puzzles like ARC.
A key idea is "compositionality" - building big ideas from small ones, like LEGOs. This is powerful but can also be overwhelming. Another important idea is "abstraction" - understanding things simply, without getting lost in details, and knowing there are different levels of understanding.
Ultimately, they believe the best AI will need to explore, experiment, and build models of the world, much like humans do when learning something new.
SPONSOR MESSAGES:
***
Tufa AI Labs is a brand new research lab in Zurich started by Benjamin Crouzier focussed on o-series style reasoning and AGI. They are hiring a Chief Engineer and ML engineers. Events in Zurich.
Goto https://tufalabs.ai/
***
TRANSCRIPT:
https://www.dropbox.com/scl/fi/3ngggvhb3tnemw879er5y/BASIS.pdf?rlkey=lr2zbj3317mex1q5l0c2rsk0h&dl=0
Zenna Tavares:
http://www.zenna.org/
Kevin Ellis:
https://www.cs.cornell.edu/~ellisk/
TOC:
1. Compositionality and Learning Foundations
[00:00:00] 1.1 Compositional Search and Learning Challenges
[00:03:55] 1.2 Bayesian Learning and World Models
[00:12:05] 1.3 Programming Languages and Compositionality Trade-offs
[00:15:35] 1.4 Inductive vs Transductive Approaches in AI Systems
2. Neural-Symbolic Program Synthesis
[00:27:20] 2.1 Integration of LLMs with Traditional Programming and Meta-Programming
[00:30:43] 2.2 Wake-Sleep Learning and DreamCoder Architecture
[00:38:26] 2.3 Program Synthesis from Interactions and Hidden State Inference
[00:41:36] 2.4 Abstraction Mechanisms and Resource Rationality
[00:48:38] 2.5 Inductive Biases and Causal Abstraction in AI Systems
3. Abstract Reasoning Systems
[00:52:10] 3.1 Abstract Concepts and Grid-Based Transformations in ARC
[00:56:08] 3.2 Induction vs Transduction Approaches in Abstract Reasoning
[00:59:12] 3.3 ARC Limitations and Interactive Learning Extensions
[01:06:30] 3.4 Wake-Sleep Program Learning and Hybrid Approaches
[01:11:37] 3.5 Project MARA and Future Research Directions
REFS:
[00:00:25] DreamCoder, Kevin Ellis et al.
https://arxiv.org/abs/2006.08381
[00:01:10] Mind Your Step, Ryan Liu et al.
https://arxiv.org/abs/2410.21333
[00:06:05] Bayesian inference, Griffiths, T. L., Kemp, C., & Tenenbaum, J. B.
https://psycnet.apa.org/record/2008-06911-003
[00:13:00] Induction and Transduction, Wen-Ding Li, Zenna Tavares, Yewen Pu, Kevin Ellis
https://arxiv.org/abs/2411.02272
[00:23:15] Neurosymbolic AI, Garcez, Artur d'Avila et al.
https://arxiv.org/abs/2012.05876
[00:33:50] Induction and Transduction (II), Wen-Ding Li, Kevin Ellis et al.
https://arxiv.org/abs/2411.02272
[00:38:35] ARC, François Chollet
https://arxiv.org/abs/1911.01547
[00:39:20] Causal Reactive Programs, Ria Das, Joshua B. Tenenbaum, Armando Solar-Lezama, Zenna Tavares
http://www.zenna.org/publications/autumn2022.pdf
[00:42:50] MuZero, Julian Schrittwieser et al.
http://arxiv.org/pdf/1911.08265
[00:43:20] VisualPredicator, Yichao Liang
https://arxiv.org/abs/2410.23156
[00:48:55] Bayesian models of cognition, Joshua B. Tenenbaum
https://mitpress.mit.edu/9780262049412/bayesian-models-of-cognition/
[00:49:30] The Bitter Lesson, Rich Sutton
http://www.incompleteideas.net/IncIdeas/BitterLesson.html
[01:06:35] Program induction, Kevin Ellis, Wen-Ding Li
https://arxiv.org/pdf/2411.02272
[01:06:50] DreamCoder (II), Kevin Ellis et al.
https://arxiv.org/abs/2006.08381
[01:11:55] Project MARA, Zenna Tavares, Kevin Ellis
https://www.basis.ai/blog/mara/
Next Episode
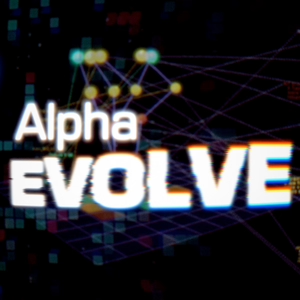
Google AlphaEvolve - Discovering new science (exclusive interview)
Today GoogleDeepMind released AlphaEvolve: a Gemini coding agent for algorithm discovery. It beat the famous Strassen algorithm for matrix multiplication set 56 years ago. Google has been killing it recently. We had early access to the paper and interviewed the researchers behind the work.
AlphaEvolve: A Gemini-powered coding agent for designing advanced algorithms
https://deepmind.google/discover/blog/alphaevolve-a-gemini-powered-coding-agent-for-designing-advanced-algorithms/
Authors: Alexander Novikov*, Ngân Vũ*, Marvin Eisenberger*, Emilien Dupont*, Po-Sen Huang*, Adam Zsolt Wagner*, Sergey Shirobokov*, Borislav Kozlovskii*, Francisco J. R. Ruiz, Abbas Mehrabian, M. Pawan Kumar, Abigail See, Swarat Chaudhuri, George Holland, Alex Davies, Sebastian Nowozin, Pushmeet Kohli, Matej Balog*
(* indicates equal contribution or special designation, if defined elsewhere)
SPONSOR MESSAGES:
***
Tufa AI Labs is a brand new research lab in Zurich started by Benjamin Crouzier focussed on o-series style reasoning and AGI. They are hiring a Chief Engineer and ML engineers. Events in Zurich.
Goto https://tufalabs.ai/
***
AlphaEvolve works like a very smart, tireless programmer. It uses powerful AI language models (like Gemini) to generate ideas for computer code. Then, it uses an "evolutionary" process – like survival of the fittest for programs. It tries out many different program ideas, automatically tests how well they solve a problem, and then uses the best ones to inspire new, even better programs.
Beyond this mathematical breakthrough, AlphaEvolve has already been used to improve real-world systems at Google, such as making their massive data centers run more efficiently and even speeding up the training of the AI models that power AlphaEvolve itself. The discussion also covers how humans work with AlphaEvolve, the challenges of making AI discover things, and the exciting future of AI helping scientists make new discoveries.
In short, AlphaEvolve is a powerful new AI tool that can invent new algorithms and solve complex problems, showing how AI can be a creative partner in science and engineering.
Guests:
Matej Balog: https://x.com/matejbalog
Alexander Novikov: https://x.com/SashaVNovikov
REFS:
MAP Elites [Jean-Baptiste Mouret, Jeff Clune]
https://arxiv.org/abs/1504.04909
FunSearch [Bernardino Romera-Paredes, Mohammadamin Barekatain, Alexander Novikov, Matej Balog, M. Pawan Kumar, Emilien Dupont, Francisco J. R. Ruiz, Jordan S. Ellenberg, Pengming Wang, Omar Fawzi, Pushmeet Kohli & Alhussein Fawzi]
https://www.nature.com/articles/s41586-023-06924-6
TOC:
[00:00:00] Introduction: Alpha Evolve's Breakthroughs, DeepMind's Lineage, and Real-World Impact
[00:12:06] Introducing AlphaEvolve: Concept, Evolutionary Algorithms, and Architecture
[00:16:56] Search Challenges: The Halting Problem and Enabling Creative Leaps
[00:23:20] Knowledge Augmentation: Self-Generated Data, Meta-Prompting, and Library Learning
[00:29:08] Matrix Multiplication Breakthrough: From Strassen to AlphaEvolve's 48 Multiplications
[00:39:11] Problem Representation: Direct Solutions, Constructors, and Search Algorithms
[00:46:06] Developer Reflections: Surprising Outcomes and Superiority over Simple LLM Sampling
[00:51:42] Algorithmic Improvement: Hill Climbing, Program Synthesis, and Intelligibility
[01:00:24] Real-World Application: Complex Evaluations and Robotics
[01:05:39] Role of LLMs & Future: Advanced Models, Recursive Self-Improvement, and Human-AI Collaboration
[01:11:22] Resource Considerations: Compute Costs of AlphaEvolve
This is a trial of posting videos on Spotify, thoughts? Email me or chat in our Discord
If you like this episode you’ll love
Episode Comments
Featured in these lists
Generate a badge
Get a badge for your website that links back to this episode
<a href="https://goodpods.com/podcasts/machine-learning-street-talk-mlst-213859/prof-randall-balestriero-llms-without-pretraining-and-ssl-89859338"> <img src="https://storage.googleapis.com/goodpods-images-bucket/badges/generic-badge-1.svg" alt="listen to prof. randall balestriero - llms without pretraining and ssl on goodpods" style="width: 225px" /> </a>
Copy