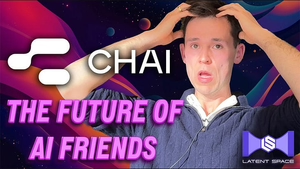
Outlasting Noam Shazeer, crowdsourcing Chat + AI with >1.4m DAU, and becoming the "Western DeepSeek" — with William Beauchamp, Chai Research
01/26/25 • 75 min
One last Gold sponsor slot is available for the AI Engineer Summit in NYC. Our last round of invites is going out soon - apply here - If you are building AI agentsor AI engteams , this will be the single highest-signal conference of the year for you!
While the world melts down over DeepSeek, few are talking about the OTHER notable group of former hedge fund traders who pivoted into AI and built a remarkably profitable consumer AI business with a tiny team with incredibly cracked engineering team — Chai Research. In short order they have:
Started a Chat AI company well before Noam Shazeer started Character AI, and outlasted his departure.
Crossed 1m DAU in 2.5 years - William updates us on the pod that they’ve hit 1.4m DAU now, another +40% from a few months ago. Revenue crossed >$22m.
Launched the Chaiverse model crowdsourcing platform - taking 3-4 week A/B testing cycles down to 3-4 hours, and deploying >100 models a week.
While they’re not paying million dollar salaries, you can tell they’re doing pretty well for an 11 person startup:
The Chai Recipe: Building infra for rapid evals
Remember how the central thesis of LMarena (formerly LMsys) is that the only comprehensive way to evaluate LLMs is to let users try them out and pick winners?
At the core of Chai is a mobile app that looks like Character AI, but is actually the largest LLM A/B testing arena in the world, specialized on retaining chat users for Chai’s usecases (therapy, assistant, roleplay, etc). It’s basically what LMArena would be if taken very, very seriously at one company (with $1m in prizes to boot):
Chai publishes occasional research on how they think about this, including talks at their Palo Alto office:
William expands upon this in today’s podcast (34 mins in):
Fundamentally, the way I would describe it is when you're building anything in life, you need to be able to evaluate it. And through evaluation, you can iterate, we can look at benchmarks, and we can say the issues with benchmarks and why they may not generalize as well as one would hope in the challenges of working with them. But something that works incredibly well is getting feedback from humans. And so we built this thing where anyone can submit a model to our developer backend, and it gets put in front of 5000 users, and the users can rate it.
And we can then have a really accurate ranking of like which model, or users finding more engaging or more entertaining. And it gets, you know, it's at this point now, where every day we're able to, I mean, we evaluate between 20 and 50 models, LLMs, every single day, right. So even though we've got only got a team of, say, five AI researchers, they're able to iterate a huge quantity of LLMs, right. So our team ships, let's just say minimum 100 LLMs a week is what we're able to iterate through. Now, before that moment in time, we might iterate through three a week, we might, you know, there was a time when even doing like five a month was a challenge, right? By being able to change the feedback loops to the point where it's not, let's launch these three models, let's do an A-B test, let's assign, let's do different cohorts, let's wait 30 days to see what the day 30 retention is, which is the kind of the, if you're doing an app, that's like A-B testing 101 would be, do a 30-day retention test, assign different treatments to different cohorts and come back in 30 days. So that's insanely slow. That's just, it's too slow. And so we were able to get that 30-day feedback loop all the way down to something like three hours.
One last Gold sponsor slot is available for the AI Engineer Summit in NYC. Our last round of invites is going out soon - apply here - If you are building AI agentsor AI engteams , this will be the single highest-signal conference of the year for you!
While the world melts down over DeepSeek, few are talking about the OTHER notable group of former hedge fund traders who pivoted into AI and built a remarkably profitable consumer AI business with a tiny team with incredibly cracked engineering team — Chai Research. In short order they have:
Started a Chat AI company well before Noam Shazeer started Character AI, and outlasted his departure.
Crossed 1m DAU in 2.5 years - William updates us on the pod that they’ve hit 1.4m DAU now, another +40% from a few months ago. Revenue crossed >$22m.
Launched the Chaiverse model crowdsourcing platform - taking 3-4 week A/B testing cycles down to 3-4 hours, and deploying >100 models a week.
While they’re not paying million dollar salaries, you can tell they’re doing pretty well for an 11 person startup:
The Chai Recipe: Building infra for rapid evals
Remember how the central thesis of LMarena (formerly LMsys) is that the only comprehensive way to evaluate LLMs is to let users try them out and pick winners?
At the core of Chai is a mobile app that looks like Character AI, but is actually the largest LLM A/B testing arena in the world, specialized on retaining chat users for Chai’s usecases (therapy, assistant, roleplay, etc). It’s basically what LMArena would be if taken very, very seriously at one company (with $1m in prizes to boot):
Chai publishes occasional research on how they think about this, including talks at their Palo Alto office:
William expands upon this in today’s podcast (34 mins in):
Fundamentally, the way I would describe it is when you're building anything in life, you need to be able to evaluate it. And through evaluation, you can iterate, we can look at benchmarks, and we can say the issues with benchmarks and why they may not generalize as well as one would hope in the challenges of working with them. But something that works incredibly well is getting feedback from humans. And so we built this thing where anyone can submit a model to our developer backend, and it gets put in front of 5000 users, and the users can rate it.
And we can then have a really accurate ranking of like which model, or users finding more engaging or more entertaining. And it gets, you know, it's at this point now, where every day we're able to, I mean, we evaluate between 20 and 50 models, LLMs, every single day, right. So even though we've got only got a team of, say, five AI researchers, they're able to iterate a huge quantity of LLMs, right. So our team ships, let's just say minimum 100 LLMs a week is what we're able to iterate through. Now, before that moment in time, we might iterate through three a week, we might, you know, there was a time when even doing like five a month was a challenge, right? By being able to change the feedback loops to the point where it's not, let's launch these three models, let's do an A-B test, let's assign, let's do different cohorts, let's wait 30 days to see what the day 30 retention is, which is the kind of the, if you're doing an app, that's like A-B testing 101 would be, do a 30-day retention test, assign different treatments to different cohorts and come back in 30 days. So that's insanely slow. That's just, it's too slow. And so we were able to get that 30-day feedback loop all the way down to something like three hours.
Previous Episode
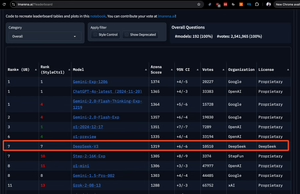
Everything you need to run Mission Critical Inference (ft. DeepSeek v3 + SGLang)
Sponsorships and applications for the AI Engineer Summit in NYC are live ! (Speaker CFPs have closed) If you are building AI agents or leading teams of AI Engineers, this will be the single highest-signal conference of the year for you.
Right after Christmas, the Chinese Whale Bros ended 2024 by dropping the last big model launch of the year: DeepSeek v3. Right now on LM Arena, DeepSeek v3 has a score of 1319, right under the full o1 model, Gemini 2, and 4o latest. This makes it the best open weights model in the world in January 2025.
There has been a big recent trend in Chinese labs releasing very large open weights models, with TenCent releasing Hunyuan-Large in November and Hailuo releasing MiniMax-Text this week, both over 400B in size. However these extra-large language models are very difficult to serve.
Baseten was the first of the Inference neocloud startups to get DeepSeek V3 online, because of their H200 clusters, their close collaboration with the DeepSeek team and early support of SGLang, a relatively new VLLM alternative that is also used at frontier labs like X.ai. Each H200 has 141 GB of VRAM with 4.8 TB per second of bandwidth, meaning that you can use 8 H200's in a node to inference DeepSeek v3 in FP8, taking into account KV Cache needs.
We have been close to Baseten since Sarah Guo introduced Amir Haghighat to swyx, and they supported the very first Latent Space Demo Day in San Francisco, which was effectively the trial run for swyx and Alessio to work together!
Since then, Philip Kiely also led a well attended workshop on TensorRT LLM at the 2024 World's Fair.
We worked with him to get two of their best representatives, Amir and Lead Model Performance Engineer Yineng Zhang, to discuss DeepSeek, SGLang, and everything they have learned running Mission Critical Inference workloads at scale for some of the largest AI products in the world.
The Three Pillars of Mission Critical Inference
We initially planned to focus the conversation on SGLang, but Amir and Yineng were quick to correct us that the choice of inference framework is only the simplest, first choice of 3 things you need for production inference at scale:
“I think it takes three things, and each of them individually is necessary but not sufficient:
Performance at the model level: how fast are you running this one model running on a single GPU, let's say. The framework that you use there can, can matter. The techniques that you use there can matter. The MLA technique, for example, that Yineng mentioned, or the CUDA kernels that are being used. But there's also techniques being used at a higher level, things like speculative decoding with draft models or with Medusa heads. And these are implemented in the different frameworks, or you can even implement it yourself, but they're not necessarily tied to a single framework. But using speculative decoding gets you massive upside when it comes to being able to handle high throughput. But that's not enough. Invariably, that one model running on a single GPU, let's say, is going to get too much traffic that it cannot handle.
Horizontal scaling at the cluster/region level: And at that point, you need to horizontally scale it. That's not an ML problem. That's not a PyTorch problem. That's an infrastructure problem. How quickly do you go from, a single replica of that model to 5, to 10, to 100. And so that's the second, that's the second pillar that is necessary for running these machine critical inference workloads....
Next Episode
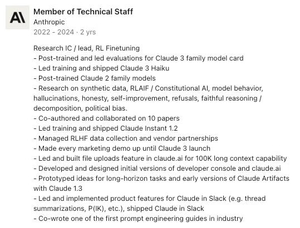
The Agent Reasoning Interface: o1/o3, Claude 3, ChatGPT Canvas, Tasks, and Operator — with Karina Nguyen of OpenAI
Sponsorships and tickets for the AI Engineer Summit are selling fast ! See the new website with speakers and schedules live!
If you are building AI agents or leading teams of AI Engineers, this will be the single highest-signal conference of the year for you, this Feb 20-22nd in NYC.
We’re pleased to share that Karina will be presenting OpenAI’s closing keynote at the AI Engineer Summit. We were fortunate to get some time with her today to introduce some of her work, and hope this serves as nice background for her talk!
There are very few early AI careers that have been as impactful as Karina Nguyen’s. After stints at Notion, Square, Dropbox, Primer, the New York Times, and UC Berkeley, She joined Anthropic as employee ~60 and worked on a wide range of research/product roles for Claude 1, 2, and 3. We’ll just let her LinkedIn speak for itself:
Now, as Research manager and Post-training lead in Model Behavior at OpenAI, she creates new interaction paradigms for reasoning interfaces and capabilities, like ChatGPT Canvas, Tasks, SimpleQA, streaming chain-of-thought for o1 models, and more via novel synthetic model training.
Ideal AI Research+Product Process
In the podcast we got a sense of what Karina has found works for her and her team to be as productive as they have been:
Write PRD (Define what you want)
Funding (Get resources)
Prototype Prompted Baseline (See what’s possible)
Write and Run Evals (Get failures to hillclimb)
Model training (Exceed baseline without overfitting)
Bugbash (Find bugs and solve them)
Ship (Get users!)
We could turn this into a snazzy viral graphic but really this is all it is. Simple to say, difficult to do well. Hopefully it helps you define your process if you do similar product-research work.
Show Notes
Our Reasoning Price War post
Karina LinkedIn, Website, Twitter
Karina on Claude Artifacts
Karina on Claude 3 Benchmarks
Inspiration for Artifacts / Canvas from early UX work she did on GPT-3
“i really believe that things like canvas and tasks should and could have happened like 2 yrs ago, idk why we are lagging in the form factors” (tweet)
Our article on prompting o1 vs Karina’s Claude prompting principles
Canvas:
If you like this episode you’ll love
Episode Comments
Generate a badge
Get a badge for your website that links back to this episode
<a href="https://goodpods.com/podcasts/latent-space-the-ai-engineer-podcast-262472/outlasting-noam-shazeer-crowdsourcing-chat-ai-with-14m-dau-and-becomin-82917955"> <img src="https://storage.googleapis.com/goodpods-images-bucket/badges/generic-badge-1.svg" alt="listen to outlasting noam shazeer, crowdsourcing chat + ai with >1.4m dau, and becoming the "western deepseek" — with william beauchamp, chai research on goodpods" style="width: 225px" /> </a>
Copy