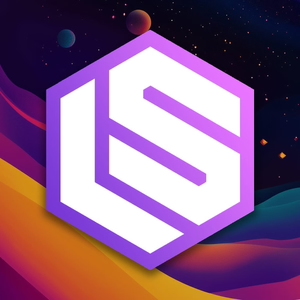
Latent Space: The AI Engineer Podcast
swyx + Alessio

2 Listeners
All episodes
Best episodes
Top 10 Latent Space: The AI Engineer Podcast Episodes
Goodpods has curated a list of the 10 best Latent Space: The AI Engineer Podcast episodes, ranked by the number of listens and likes each episode have garnered from our listeners. If you are listening to Latent Space: The AI Engineer Podcast for the first time, there's no better place to start than with one of these standout episodes. If you are a fan of the show, vote for your favorite Latent Space: The AI Engineer Podcast episode by adding your comments to the episode page.
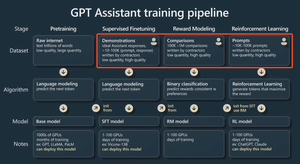
From RLHF to RLHB: The Case for Learning from Human Behavior - with Jeffrey Wang and Joe Reeve of Amplitude
Latent Space: The AI Engineer Podcast
06/08/23 • 49 min
Welcome to the almost 3k latent space explorers that joined us last month! We’re holding our first SF listener meetup with Practical AI next Monday; join us if you want to meet past guests and put faces to voices! All events are in /community.
Who among you regularly click the ubiquitous 👍 /👎 buttons in ChatGPT/Bard/etc?
Anyone? I don’t see any hands up.
OpenAI has told us how important reinforcement learning from human feedback (RLHF) is to creating the magic that is ChatGPT, but we know from our conversation with Databricks’ Mike Conover just how hard it is to get just 15,000 pieces of explicit, high quality human responses.
We are shockingly reliant on good human feedback. Andrej Karpathy’s recent keynote at Microsoft Build on the State of GPT demonstrated just how much of the training process relies on contractors to supply the millions of items of human feedback needed to make a ChatGPT-quality LLM (highlighted by us in red):
But the collection of good feedback is an incredibly messy problem. First of all, if you have contractors paid by the datapoint, they are incentivized to blast through as many as possible without much thought. So you hire more contractors and double, maybe triple, your costs. Ok, you say, lets recruit missionaries, not mercenaries. People should volunteer their data! Then you run into the same problem we and any consumer review platform run into - the vast majority of people send nothing at all, and those who do are disproportionately representing negative reactions. More subtle problems emerge when you try to capture subjective human responses - the reason that ChatGPT responses tend to be inhumanly verbose, is because humans have a well documented “longer = better” bias when classifying responses in a “laboratory setting”.
The fix for this, of course, is to get out of the lab and learn from real human behavior, not artificially constructed human feedback. You don’t see a thumbs up/down button in GitHub Copilot nor Codeium nor Codium. Instead, they work an implicit accept/reject event into the product workflow, such that you cannot help but to give feedback while you use the product. This way you hear from all your users, in their natural environments doing valuable tasks they are familiar with. The prototypal example in this is Midjourney, who unobtrusively collect 1 of 9 types of feedback from every user as part of their workflow, in exchange for much faster first draft image generations:
The best known public example of AI product telemetry is in the Copilot-Explorer writeup, which checks for the presence of generated code after 15-600 second intervals, which enables GitHub to claim that 40% of code is generated by Copilot.
This is fantastic and “obviously” the future of productized AI. Every AI application should figure out how to learn from all their real users, not some contractors in a foreign country. Most prompt engineers and prompt engineering tooling also tend to focus on pre-production prototyping, but could also benefit from A/B testing their prompts in the real world.
In short, AI may need Analytics more than Analytics needs AI.
Amplitude’s Month of AI
This is why Amplitude is going hard on AI - and why we recently spent a weekend talking to Jeffrey Wang, cofounder and chief architect at Amplitude, and Joe Reeve, head of AI, recording a li...
1 Listener
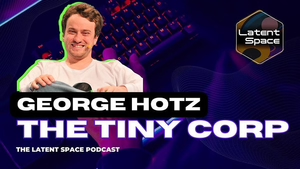
Commoditizing the Petaflop — with George Hotz of the tiny corp
Latent Space: The AI Engineer Podcast
06/20/23 • 72 min
We are now launching our dedicated new YouTube and Twitter! Any help in amplifying our podcast would be greatly appreciated, and of course, tell your friends!
Notable followon discussions collected on Twitter, Reddit, Reddit, Reddit, HN, and HN. Please don’t obsess too much over the GPT4 discussion as it is mostly rumor; we spent much more time on tinybox/tinygrad on which George is the foremost authority!
We are excited to share the world’s first interview with George Hotz on the tiny corp!
If you don’t know George, he was the first person to unlock the iPhone, jailbreak the PS3, went on to start Comma.ai, and briefly “interned” at the Elon Musk-run Twitter.
Tinycorp is the company behind the deep learning framework tinygrad, as well as the recently announced tinybox, a new $15,000 “luxury AI computer” aimed at local model training and inference, aka your “personal compute cluster”:
738 FP16 TFLOPS
144 GB GPU RAM
5.76 TB/s RAM bandwidth
30 GB/s model load bandwidth (big llama loads in around 4 seconds)
AMD EPYC CPU
1600W (one 120V outlet)
Runs 65B FP16 LLaMA out of the box (using tinygrad, subject to software development risks)
(In the episode, we also talked about the future of the tinybox as the intelligence center of every home that will help run models, at-home robots, and more. Make sure to check the timestamps 👀 )
The tiny corp manifesto
There are three main theses to tinycorp:
If XLA/PrimTorch are CISC, tinygrad is RISC: CISC (Complex Instruction Set Computing) are more complex instruction sets where a single instruction can execute many low-level operations. RISC (Reduced Instruction Set Computing) are smaller, and only let you execute a single low-level operation per instruction, leading to faster and more efficient instruction execution. If you’ve used the Apple Silicon M1/M2, AMD Ryzen, or Raspberry Pi, you’ve used a RISC computer.
If you can’t write a fast ML framework for GPU, you can’t write one for your own chip: there are many “AI chips” companies out there, and they all started from taping the chip. Some of them like Cerebras are still building, while others like Graphcore seem to be struggling. But building chips with higher TFLOPS isn’t enough: “There’s a great chip already on the market. For $999, you get a 123 TFLOP card with 24 GB of 960 GB/s RAM. This is the best FLOPS per dollar today, and yet...nobody in ML uses it.”, referring to the AMD RX 7900 XTX. NVIDIA’s lead is not only thanks to high-performing cards, but also thanks to a great developer platform in CUDA. Starting with the chip development rather than the dev toolkit is much more cost-intensive, so tinycorp is starting by writing a framework for off-the-shelf hardware rather than taping their own chip.
Turing completeness considered harmful: Once you call in to Turing complete kernels, you can 1 Listener
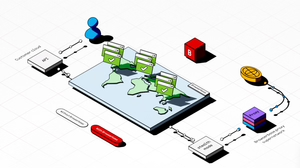
Open Operator, Serverless Browsers and the Future of Computer-Using Agents
Latent Space: The AI Engineer Podcast
02/28/25 • 61 min
Today's episode is with Paul Klein, founder of Browserbase. We talked about building browser infrastructure for AI agents, the future of agent authentication, and their open source framework Stagehand.
[00:00:00] Introductions
[00:04:46] AI-specific challenges in browser infrastructure
[00:07:05] Multimodality in AI-Powered Browsing
[00:12:26] Running headless browsers at scale
[00:18:46] Geolocation when proxying
[00:21:25] CAPTCHAs and Agent Auth
[00:28:21] Building “User take over” functionality
[00:33:43] Stagehand: AI web browsing framework
[00:38:58] OpenAI's Operator and computer use agents
[00:44:44] Surprising use cases of Browserbase
[00:47:18] Future of browser automation and market competition
[00:53:11] Being a solo founder
Transcript
Alessio [00:00:04]: Hey everyone, welcome to the Latent Space podcast. This is Alessio, partner and CTO at Decibel Partners, and I'm joined by my co-host Swyx, founder of Smol.ai.
swyx [00:00:12]: Hey, and today we are very blessed to have our friends, Paul Klein, for the fourth, the fourth, CEO of Browserbase. Welcome.
Paul [00:00:21]: Thanks guys. Yeah, I'm happy to be here. I've been lucky to know both of you for like a couple of years now, I think. So it's just like we're hanging out, you know, with three ginormous microphones in front of our face. It's totally normal hangout.
swyx [00:00:34]: Yeah. We've actually mentioned you on the podcast, I think, more often than any other Solaris tenant. Just because like you're one of the, you know, best performing, I think, LLM tool companies that have started up in the last couple of years.
Paul [00:00:50]: Yeah, I mean, it's been a whirlwind of a year, like Browserbase is actually pretty close to our first birthday. So we are one years old. And going from, you know, starting a company as a solo founder to... To, you know, having a team of 20 people, you know, a series A, but also being able to support hundreds of AI companies that are building AI applications that go out and automate the web. It's just been like, really cool. It's been happening a little too fast. I think like collectively as an AI industry, let's just take a week off together. I took my first vacation actually two weeks ago, and Operator came out on the first day, and then a week later, DeepSeat came out. And I'm like on vacation trying to chill. I'm like, we got to build with this stuff, right? So it's been a breakneck year. But I'm super happy to be here and like talk more about all the stuff we're seeing. And I'd love to hear kind of what you guys are excited about too, and share with it, you know?
swyx [00:01:39]: Where to start? So people, you've done a bunch of podcasts. I think I strongly recommend Jack Bridger's Scaling DevTools, as well as Turner Novak's The Peel. And, you know, I'm sure there's others. So you covered your Twilio story in the past, talked about StreamClub, you got acquired to Mux, and then you left to start Browserbase. So maybe we just start with what is Browserbase? Yeah.
Paul [00:02:02]: Browserbase is the web browser for your AI. We're building headless browser infrastructure, which are browsers that run in a server environment that's accessible to developers via APIs and SDKs. It's really hard to run a web browser in the cloud. You guys are probably running Chrome on your computers, and that's using a lot of resources, right? So if you want to run a web browser or thousands of web browsers, you can't just spin up a bunch of lambdas. You actually need to use a secure containerized environment. You have to scale it up and down. It's a stateful system. And that infrastructure is, like, super painful. And I know that firsthand, because at my last company, StreamClub, I was CTO, and I was building our own internal headless browser infrastructure. That's actually why we sold the company, is because Mux really wanted to buy our headless browser infrastructure that we'd built. And it's just a super hard problem. And I actually told my co-founders, I would never start another company unless it was a browser infrastructure company. And it turns out that's really necessary in the age of AI, when AI can actually go out and interact with websites, click on buttons, fill in forms. You need AI to do all of that work in an actual browser running somewhere on a server. And BrowserBase powers that.
swyx [00:03:08]: While you're talking about it, it occurred to me, not that you're going to be acquired or anything, but it occurred to me that it would be really funny if you became the Nikita Beer of headless browser companies. You just have one trick, ...

1 Listener
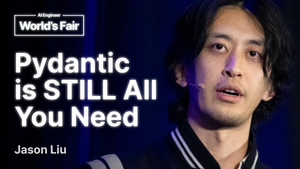
Agent Engineering with Pydantic + Graphs — with Samuel Colvin
Latent Space: The AI Engineer Podcast
02/06/25 • 64 min
Did you know that adding a simple Code Interpreter took o3 from 9.2% to 32% on FrontierMath? The Latent Space crew is hosting a hack night Feb 11th in San Francisco focused on CodeGen use cases, co-hosted with E2B and Edge AGI; watch E2B’s new workshop and RSVP here!
We’re happy to announce that today’s guest Samuel Colvin will be teaching his very first Pydantic AI workshop at the newly announced AI Engineer NYC Workshops day on Feb 22! 25 tickets left.
If you’re a Python developer, it’s very likely that you’ve heard of Pydantic. Every month, it’s downloaded >300,000,000 times, making it one of the top 25 PyPi packages. OpenAI uses it in its SDK for structured outputs, it’s at the core of FastAPI, and if you’ve followed our AI Engineer Summit conference, Jason Liu of Instructor has given two great talks about it: “Pydantic is all you need” and “Pydantic is STILL all you need”.
Now, Samuel Colvin has raised $17M from Sequoia to turn Pydantic from an open source project to a full stack AI engineer platform with Logfire, their observability platform, and PydanticAI, their new agent framework.
Logfire: bringing OTEL to AI
OpenTelemetry recently merged Semantic Conventions for LLM workloads which provides standard definitions to track performance like gen_ai.server.time_per_output_token. In Sam’s view at least 80% of new apps being built today have some sort of LLM usage in them, and just like web observability platform got replaced by cloud-first ones in the 2010s, Logfire wants to do the same for AI-first apps.
If you’re interested in the technical details, Logfire migrated away from Clickhouse to Datafusion for their backend. We spent some time on the importance of picking open source tools you understand and that you can actually contribute to upstream, rather than the more popular ones; listen in ~43:19 for that part.
Agents are the killer app for graphs
Pydantic AI is their attempt at taking a lot of the learnings that LangChain and the other early LLM frameworks had, and putting Python best practices into it. At an API level, it’s very similar to the other libraries: you can call LLMs, create agents, do function calling, do evals, etc.
They define an “Agent” as a container with a system prompt, tools, structured result, and an LLM. Under the hood, each Agent is now a graph of function calls that can orchestrate multi-step LLM interactions. You can start simple, then move toward fully dynamic graph-based control flow if needed.
“We were compelled enough by graphs once we got them right that our agent implementation [...] is now actually a graph under the hood.”
Why Graphs?
More natural for complex or multi-step AI workflows.
Easy to visualize and debug with mermaid diagrams.
Potential for distributed runs, or “waiting days” between steps in certain flows.
In parallel, you see folks like Emil Eifrem of Neo4j talk about GraphRAG as another place where graphs fit really well in the AI stack, so it might be time for more people to take them seriously.
Full Video Episode
Chapters
00:00:00 Introductions
00:00:24 Origins of Pydantic
...
1 Listener
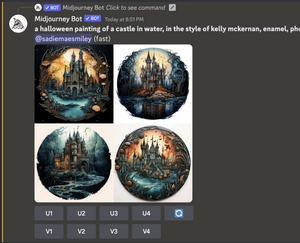
AI Engineering for Art — with comfyanonymous, of ComfyUI
Latent Space: The AI Engineer Podcast
01/04/25 • 55 min
Applications for the NYC AI Engineer Summit, focused on Agents at Work, are open!
When we first started Latent Space, in the lightning round we’d always ask guests: “What’s your favorite AI product?”. The majority would say Midjourney. The simple UI of prompt → very aesthetic image turned it into a $300M+ ARR bootstrapped business as it rode the first wave of AI image generation.
In open source land, StableDiffusion was congregating around AUTOMATIC1111 as the de-facto web UI. Unlike Midjourney, which offered some flags but was mostly prompt-driven, A1111 let users play with a lot more parameters, supported additional modalities like img2img, and allowed users to load in custom models. If you’re interested in some of the SD history, you can look at our episodes with Lexica, Replicate, and Playground.
One of the people involved with that community was comfyanonymous, who was also part of the Stability team in 2023, decided to build an alternative called ComfyUI, now one of the fastest growing open source projects in generative images, and is now the preferred partner for folks like Black Forest Labs’s Flux Tools on Day 1. The idea behind it was simple: “Everyone is trying to make easy to use interfaces. Let me try to make a powerful interface that's not easy to use.”
Unlike its predecessors, ComfyUI does not have an input text box. Everything is based around the idea of a node: there’s a text input node, a CLIP node, a checkpoint loader node, a KSampler node, a VAE node, etc. While daunting for simple image generation, the tool is amazing for more complex workflows since you can break down every step of the process, and then chain many of them together rather than manually switching between tools. You can also re-start execution halfway instead of from the beginning, which can save a lot of time when using larger models.
To give you an idea of some of the new use cases that this type of UI enables:
Sketch something → Generate an image with SD from sketch → feed it into SD Video to animate
Generate an image of an object → Turn into a 3D asset → Feed into interactive experiences
Input audio → Generate audio-reactive videos
Their Examples page also includes some of the more common use cases like AnimateDiff, etc. They recently launched the Comfy Registry, an online library of different nodes that users can pull from rather than having to build everything from scratch. The project has >60,000 Github stars, and as the community grows, some of the projects that people build have gotten quite complex:
The most interesting thing about Comfy is that it’s not a UI, it’s a runtime. You can build full applications on top of image models simply by using Comfy. You can expose Comfy workflows as an endpoint and chain them together just like you chain a single node. We’re seeing the rise of AI Engineering applied to art.
Major Tom’s ComfyUI Resources from the Latent Space Discord
Major shoutouts to Major Tom on the LS Discord who is a image generation expert, who offered these pointers:
“best thing about comfy is the fact it supports almost immediately every new thing that comes out - unlike A1111 or forge, which still don't support flux cnet for instance. It will be perfect tool when conflicting nodes will be resolved”
AP Workflows from Alessandro Perili are a nice example of an all-in-one train-evaluate-generate system built atop Comfy
ComfyUI YouTubers to learn from:
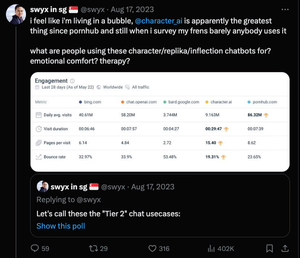
Emulating Humans with NSFW Chatbots - with Jesse Silver
Latent Space: The AI Engineer Podcast
05/16/24 • 54 min
Disclaimer: today’s episode touches on NSFW topics. There’s no graphic content or explicit language, but we wouldn’t recommend blasting this in work environments.
Product website: https://usewhisper.me/
For over 20 years it’s been an open secret that porn drives many new consumer technology innovations, from VHS and Pay-per-view to VR and the Internet. It’s been no different in AI - many of the most elite Stable Diffusion and Llama enjoyers and merging/prompting/PEFT techniques were born in the depths of subreddits and 4chan boards affectionately descibed by friend of the pod as The Waifu Research Department. However this topic is very under-covered in mainstream AI media because of its taboo nature.
That changes today, thanks to our new guest Jesse Silver.
The AI Waifu Explosion
In 2023, the Valley’s worst kept secret was how much the growth and incredible retention of products like Character.ai & co was being boosted by “ai waifus” (not sure what the “husband” equivalent is, but those too!).
And we can look at subreddit growth as a proxy for the general category explosion (10x’ed in the last 8 months of 2023):
While all the B2B founders were trying to get models to return JSON, the consumer applications made these chatbots extremely engaging and figured out how to make them follow their instructions and “personas” very well, with the greatest level of scrutiny and most demanding long context requirements. Some of them, like Replika, make over $50M/year in revenue, and this is -after- their controversial update deprecating Erotic Roleplay (ERP).
A couple of days ago, OpenAI announced GPT-4o (see our AI News recap) and the live voice demos were clearly inspired by the movie Her.
The Latent Space Discord did a watch party and both there and on X a ton of folks were joking at how flirtatious the model was, which to be fair was disturbing to many:
From Waifus to Fan Platforms
Where Waifus are known by human users to be explicitly AI chatbots, the other, much more challenging end of the NSFW AI market is run by AIs successfully (plausibly) emulating a specific human personality for chat and ecommerce.
You might have heard of fan platforms like OnlyFans. Users can pay for a subscription to a creator to get access to private content, similarly to Patreon and the likes, but without any NSFW restrictions or any other content policies. In 2023, OnlyFans had over $1.1B of revenue (on $5.6b of GMV).
The status quo today is that a lot of the creators outsource their chatting with fans to teams in the Philippines and other lower cost countries for ~$3/hr + 5% commission, but with very poor quality - most creators have fired multiple teams for poor service.
Today’s episode is with Jesse Silver; along with his co-founder Adam Scrivener, they run a SaaS platform that helps creators from fan platforms build AI chatbots for their fans to chat with, including selling from an inventory of digital content. Some users generate over $200,000/mo in revenue.
We talked a lot about their tech stack, why you need a state machine to successfully run multi-thousand-turn conversations, how they develop prompts and fine-tune models with DSPy, the NSFW limitations of commercial models, but one of the most interesting points is that often users know that they ...
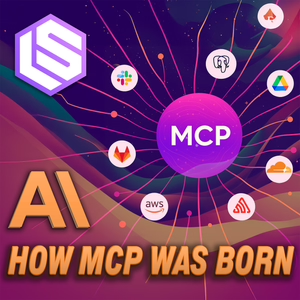
The Creators of Model Context Protocol
Latent Space: The AI Engineer Podcast
04/03/25 • 79 min
Today’s guests, David Soria Parra and Justin Spahr-Summers, are the creators of Anthropic’s Model Context Protocol (MCP). When we first wrote Why MCP Won, we had no idea how quickly it was about to win.
In the past 4 weeks, OpenAI and now Google have now announced the MCP support, effectively confirming our prediction that MCP was the presumptive winner of the agent standard wars. MCP has now overtaken OpenAPI, the incumbent option and most direct alternative, in GitHub stars (3 months ahead of conservative trendline):
For protocol and history nerds, we also asked David and Justin to tell the origin story of MCP, which we leave to the reader to enjoy (you can also skim the transcripts, or, the changelogs of a certain favored IDE). It’s incredible the impact that individual engineers solving their own problems can have on an entire industry.
Timestamps
00:00 Introduction and Guest Welcome
00:37 What is MCP?
02:00 The Origin Story of MCP
05:18 Development Challenges and Solutions
08:06 Technical Details and Inspirations
29:45 MCP vs Open API
32:48 Building MCP Servers
40:39 Exploring Model Independence in LLMs
41:36 Building Richer Systems with MCP
43:13 Understanding Agents in MCP
45:45 Nesting and Tool Confusion in MCP
49:11 Client Control and Tool Invocation
52:08 Authorization and Trust in MCP Servers
01:01:34 Future Roadmap and Stateless Servers
01:10:07 Open Source Governance and Community Involvement
01:18:12 Wishlist and Closing Remarks
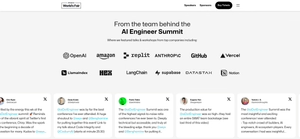
Presenting the AI Engineer World's Fair — with Sam Schillace, Deputy CTO of Microsoft
Latent Space: The AI Engineer Podcast
03/29/24 • 42 min
TL;DR: You can now buy tickets, apply to speak, or join the expo for the biggest AI Engineer event of 2024. We’re gathering *everyone* you want to meet - see you this June.
In last year’s the Rise of the AI Engineer we put our money where our mouth was and announced the AI Engineer Summit, which fortunately went well:
With ~500 live attendees and over ~500k views online, the first iteration of the AI Engineer industry affair seemed to be well received. Competing in an expensive city with 3 other more established AI conferences in the fall calendar, we broke through in terms of in-person experience and online impact.
So at the end of Day 2 we announced our second event: the AI Engineer World’s Fair. The new website is now live, together with our new presenting sponsor:
We were delighted to invite both Ben Dunphy, co-organizer of the conference and Sam Schillace, the deputy CTO of Microsoft who wrote some of the first Laws of AI Engineering while working with early releases of GPT-4, on the pod to talk about the conference and how Microsoft is all-in on AI Engineering.
Rise of the Planet of the AI Engineer
Since the first AI Engineer piece, AI Engineering has exploded:
and the title has been adopted across OpenAI, Meta, IBM, and many, many other companies:
1 year on, it is clear that AI Engineering is not only in full swing, but is an emerging global industry that is successfully bridging the gap:
between research and product,
between general-purpose foundation models and in-context use-cases,
and between the flashy weekend MVP (still great!) and the reliable, rigorously evaluated AI product deployed at massive scale, assisting hundreds of employees and driving millions in profit.
The greatly increased scope of the 2024 AI Engineer World’s Fair (more stages, more talks, more speakers, more attendees, more expo...) helps us reflect the growth of AI Engineering in three major dimensions:
Global Representation: the 2023 Summit was a mostly-American affair. This year we plan to have speakers from top AI companies across five continents, and explore the vast diversity of approaches to AI across global contexts.
Topic Coverage:
In 2023, the Summit focused on the initial questions that the community wrestled with - LLM frameworks, RAG and Vector Databases, Code Copilots and AI Agents. Those are evergreen problems that just got deeper.
This year the AI Engineering field has also embraced new core disciplines with more explicit focus on Multimodality, Evals and Ops, Open Source Models and GPU/Inference Hardware providers.
Maturity/Production-readiness: Two new tracks are dedicated toward AI in the Enterprise, government, education, finance, and more highly regulated industries or AI deployed at larger scale:
AI in the Fortune 500, covering at-scale production deployments of AI, and
AI Leadership, a closed-door, side event for technical AI leaders to discuss engineering and product leadership challenges as VPs and Heads of AI in their respective orgs.
We hope you will join Microsoft and the rest of us as either speaker, exhibitor, or attendee, in San Francisco this June. Contact us with any enquiries that don’t fall into the categories mentioned below.
Show Notes
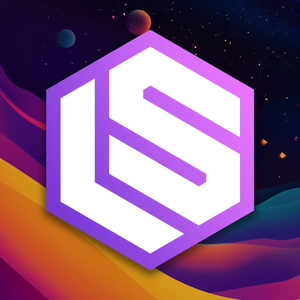
Unsupervised Learning x Latent Space Crossover Special
Latent Space: The AI Engineer Podcast
03/29/25 • -1 min
Unsupervised Learning is a podcast that interviews the sharpest minds in AI about what’s real today, what will be real in the future and what it means for businesses and the world - helping builders, researchers and founders deconstruct and understand the biggest breakthroughs.
Top guests: Noam Shazeer, Bob McGrew, Noam Brown, Dylan Patel, Percy Liang, David Luan
https://www.latent.space/p/unsupervised-learning
Timestamps
00:00 Introduction and Excitement for Collaboration
00:27 Reflecting on Surprises in AI Over the Past Year
01:44 Open Source Models and Their Adoption
06:01 The Rise of GPT Wrappers
06:55 AI Builders and Low-Code Platforms
09:35 Overhyped and Underhyped AI Trends
22:17 Product Market Fit in AI
28:23 Google's Current Momentum
28:33 Customer Support and AI
29:54 AI's Impact on Cost and Growth
31:05 Voice AI and Scheduling
32:59 Emerging AI Applications
34:12 Education and AI
36:34 Defensibility in AI Applications
40:10 Infrastructure and AI
47:08 Challenges and Future of AI
52:15 Quick Fire Round and Closing Remarks
Chapters
- 00:00:00 Introduction and Collab Excitement
- 00:00:58 Open Source and Model Adoption
- 00:01:58 Enterprise Use of Open Source Models
- 00:02:57 The Competitive Edge of Closed Source Models
- 00:03:56 DeepSea and Open Source Model Releases
- 00:04:54 Market Narrative and DeepSea Impact
- 00:05:53 AI Engineering and GPT Wrappers
- 00:06:53 AI Builders and Low-Code Platforms
- 00:07:50 Innovating Beyond Existing Paradigms
- 00:08:50 Apple and AI Product Development
- 00:09:48 Overhyped and Underhyped AI Trends
- 00:10:46 Frameworks and Protocols in AI Development
- 00:11:45 Emerging Opportunities in AI
- 00:12:44 Stateful AI and Memory Innovation
- 00:13:44 Challenges with Memory in AI Agents
- 00:14:44 The Future of Model Training Companies
- 00:15:44 Specialized Use Cases for AI Models
- 00:16:44 Vertical Models vs General Purpose Models
- 00:17:42 General Purpose vs Domain-Specific Models
- 00:18:42 Reflections on Model Companies
- 00:19:39 Model Companies Entering Product Space
- 00:20:38 Competition in AI Model and Product Sectors
- 00:21:35 Coding Agents and Market Dynamics
- 00:22:35 Defensibility in AI Applications
- 00:23:35 Investing in Underappreciated AI Ventures
- 00:24:32 Analyzing Market Fit in AI
- 00:25:31 AI Applications with Product Market Fit
- 00:26:31 OpenAI's Impact on the Market
- 00:27:31 Google and OpenAI Competition
- 00:28:31 Exploring Google's Advancements
- 00:29:29 Customer Support and AI Applications
- 00:30:27 The Future of AI in Customer Support
- 00:31:26 Cost-Cutting vs Growth in AI
- 00:32:23 Voice AI and Real-World Applications
- 00:33:23 Scaling AI Applications for Demand
- 00:34:22 Summarization and Conversational AI
- 00:35:20 Future AI Use Cases and Market Fit
- 00:36:20 AI Education and Model Capabilities
- 00:37:17 Reforming Education with AI
- 00:38:15 Defensibility in AI Apps
- 00:39:13 Network Effects and AI
- 00:40:12 AI Brand and Market Positioning
- 00:41:11 AI Application Defensibility
- 00:42:09 LLM OS and AI Infrastructure
- 00:43:06 Security and AI Application
- 00:44:06 OpenAI's Role in AI Infrastructure
- 00:45:02 The Balance of AI Applications and Infrastructure
- 00:46:02 Capital Efficiency in AI Infrastructure
- 00:47:01 Challenges in AI DevOps and Infrastructure
- 00:47:59 AI SRE and Monitoring
- 00:48:59 Scaling AI and Hardware Challenges
- 00:49:58 Reliability and Compute in AI
- 00:50:57 Nvidia's Dominance and AI Hardware
- 00:51:57 Emerging Competition in AI Silicon
- 00:52:54 Agent Authenticatio...
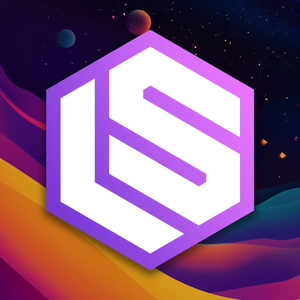
ChatGPT Codex: The Missing Manual
Latent Space: The AI Engineer Podcast
05/16/25 • 53 min
ChatGPT Codex is here - the first cloud hosted Autonomous Software Engineer (A-SWE) from OpenAI. We sat down for a quick pod with two core devs on the ChatGPT Codex team: Josh Ma and Alexander Embiricos to get the inside scoop on the origin story of Codex, from WHAM to its future roadmap.
Follow them: https://github.com/joshma and https://x.com/embirico
Chapters
00:00 Introduction to the Latent Space Podcast
- 00:59 The Launch of ChatGPT Codex
- 03:08 Personal Journeys into AI Development
- 05:50 The Evolution of Codex and AI Agents
- 08:55 Understanding the Form Factor of Codex
- 11:48 Building a Software Engineering Agent
- 14:53 Best Practices for Using AI Agents
- 17:55 The Importance of Code Structure for AI
- 21:10 Navigating Human and AI Collaboration
- 23:58 Future of AI in Software Development
- 28:18 Planning and Decision-Making in AI Development
- 31:37 User, Developer, and Model Dynamics
- 35:28 Building for the Future: Long-Term Vision
- 39:31 Best Practices for Using AI Tools
- 42:32 Understanding the Compute Platform
- 48:01 Iterative Deployment and Future Improvements
Show more best episodes

Show more best episodes
FAQ
How many episodes does Latent Space: The AI Engineer Podcast have?
Latent Space: The AI Engineer Podcast currently has 131 episodes available.
What topics does Latent Space: The AI Engineer Podcast cover?
The podcast is about Ai, Entrepreneurship, Software, Podcasts, Technology, Business and Engineering.
What is the most popular episode on Latent Space: The AI Engineer Podcast?
The episode title 'Commoditizing the Petaflop — with George Hotz of the tiny corp' is the most popular.
What is the average episode length on Latent Space: The AI Engineer Podcast?
The average episode length on Latent Space: The AI Engineer Podcast is 76 minutes.
How often are episodes of Latent Space: The AI Engineer Podcast released?
Episodes of Latent Space: The AI Engineer Podcast are typically released every 6 days, 14 hours.
When was the first episode of Latent Space: The AI Engineer Podcast?
The first episode of Latent Space: The AI Engineer Podcast was released on Feb 23, 2023.
Show more FAQ

Show more FAQ