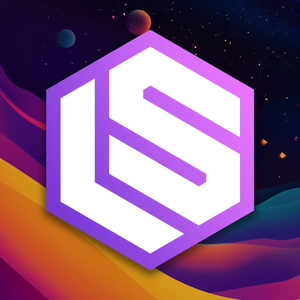
Unsupervised Learning x Latent Space Crossover Special
03/29/25 • -1 min
Unsupervised Learning is a podcast that interviews the sharpest minds in AI about what’s real today, what will be real in the future and what it means for businesses and the world - helping builders, researchers and founders deconstruct and understand the biggest breakthroughs.
Top guests: Noam Shazeer, Bob McGrew, Noam Brown, Dylan Patel, Percy Liang, David Luan
https://www.latent.space/p/unsupervised-learning
Timestamps
00:00 Introduction and Excitement for Collaboration
00:27 Reflecting on Surprises in AI Over the Past Year
01:44 Open Source Models and Their Adoption
06:01 The Rise of GPT Wrappers
06:55 AI Builders and Low-Code Platforms
09:35 Overhyped and Underhyped AI Trends
22:17 Product Market Fit in AI
28:23 Google's Current Momentum
28:33 Customer Support and AI
29:54 AI's Impact on Cost and Growth
31:05 Voice AI and Scheduling
32:59 Emerging AI Applications
34:12 Education and AI
36:34 Defensibility in AI Applications
40:10 Infrastructure and AI
47:08 Challenges and Future of AI
52:15 Quick Fire Round and Closing Remarks
Chapters
- 00:00:00 Introduction and Collab Excitement
- 00:00:58 Open Source and Model Adoption
- 00:01:58 Enterprise Use of Open Source Models
- 00:02:57 The Competitive Edge of Closed Source Models
- 00:03:56 DeepSea and Open Source Model Releases
- 00:04:54 Market Narrative and DeepSea Impact
- 00:05:53 AI Engineering and GPT Wrappers
- 00:06:53 AI Builders and Low-Code Platforms
- 00:07:50 Innovating Beyond Existing Paradigms
- 00:08:50 Apple and AI Product Development
- 00:09:48 Overhyped and Underhyped AI Trends
- 00:10:46 Frameworks and Protocols in AI Development
- 00:11:45 Emerging Opportunities in AI
- 00:12:44 Stateful AI and Memory Innovation
- 00:13:44 Challenges with Memory in AI Agents
- 00:14:44 The Future of Model Training Companies
- 00:15:44 Specialized Use Cases for AI Models
- 00:16:44 Vertical Models vs General Purpose Models
- 00:17:42 General Purpose vs Domain-Specific Models
- 00:18:42 Reflections on Model Companies
- 00:19:39 Model Companies Entering Product Space
- 00:20:38 Competition in AI Model and Product Sectors
- 00:21:35 Coding Agents and Market Dynamics
- 00:22:35 Defensibility in AI Applications
- 00:23:35 Investing in Underappreciated AI Ventures
- 00:24:32 Analyzing Market Fit in AI
- 00:25:31 AI Applications with Product Market Fit
- 00:26:31 OpenAI's Impact on the Market
- 00:27:31 Google and OpenAI Competition
- 00:28:31 Exploring Google's Advancements
- 00:29:29 Customer Support and AI Applications
- 00:30:27 The Future of AI in Customer Support
- 00:31:26 Cost-Cutting vs Growth in AI
- 00:32:23 Voice AI and Real-World Applications
- 00:33:23 Scaling AI Applications for Demand
- 00:34:22 Summarization and Conversational AI
- 00:35:20 Future AI Use Cases and Market Fit
- 00:36:20 AI Education and Model Capabilities
- 00:37:17 Reforming Education with AI
- 00:38:15 Defensibility in AI Apps
- 00:39:13 Network Effects and AI
- 00:40:12 AI Brand and Market Positioning
- 00:41:11 AI Application Defensibility
- 00:42:09 LLM OS and AI Infrastructure
- 00:43:06 Security and AI Application
- 00:44:06 OpenAI's Role in AI Infrastructure
- 00:45:02 The Balance of AI Applications and Infrastructure
- 00:46:02 Capital Efficiency in AI Infrastructure
- 00:47:01 Challenges in AI DevOps and Infrastructure
- 00:47:59 AI SRE and Monitoring
- 00:48:59 Scaling AI and Hardware Challenges
- 00:49:58 Reliability and Compute in AI
- 00:50:57 Nvidia's Dominance and AI Hardware
- 00:51:57 Emerging Competition in AI Silicon
- 00:52:54 Agent Authenticatio...
Unsupervised Learning is a podcast that interviews the sharpest minds in AI about what’s real today, what will be real in the future and what it means for businesses and the world - helping builders, researchers and founders deconstruct and understand the biggest breakthroughs.
Top guests: Noam Shazeer, Bob McGrew, Noam Brown, Dylan Patel, Percy Liang, David Luan
https://www.latent.space/p/unsupervised-learning
Timestamps
00:00 Introduction and Excitement for Collaboration
00:27 Reflecting on Surprises in AI Over the Past Year
01:44 Open Source Models and Their Adoption
06:01 The Rise of GPT Wrappers
06:55 AI Builders and Low-Code Platforms
09:35 Overhyped and Underhyped AI Trends
22:17 Product Market Fit in AI
28:23 Google's Current Momentum
28:33 Customer Support and AI
29:54 AI's Impact on Cost and Growth
31:05 Voice AI and Scheduling
32:59 Emerging AI Applications
34:12 Education and AI
36:34 Defensibility in AI Applications
40:10 Infrastructure and AI
47:08 Challenges and Future of AI
52:15 Quick Fire Round and Closing Remarks
Chapters
- 00:00:00 Introduction and Collab Excitement
- 00:00:58 Open Source and Model Adoption
- 00:01:58 Enterprise Use of Open Source Models
- 00:02:57 The Competitive Edge of Closed Source Models
- 00:03:56 DeepSea and Open Source Model Releases
- 00:04:54 Market Narrative and DeepSea Impact
- 00:05:53 AI Engineering and GPT Wrappers
- 00:06:53 AI Builders and Low-Code Platforms
- 00:07:50 Innovating Beyond Existing Paradigms
- 00:08:50 Apple and AI Product Development
- 00:09:48 Overhyped and Underhyped AI Trends
- 00:10:46 Frameworks and Protocols in AI Development
- 00:11:45 Emerging Opportunities in AI
- 00:12:44 Stateful AI and Memory Innovation
- 00:13:44 Challenges with Memory in AI Agents
- 00:14:44 The Future of Model Training Companies
- 00:15:44 Specialized Use Cases for AI Models
- 00:16:44 Vertical Models vs General Purpose Models
- 00:17:42 General Purpose vs Domain-Specific Models
- 00:18:42 Reflections on Model Companies
- 00:19:39 Model Companies Entering Product Space
- 00:20:38 Competition in AI Model and Product Sectors
- 00:21:35 Coding Agents and Market Dynamics
- 00:22:35 Defensibility in AI Applications
- 00:23:35 Investing in Underappreciated AI Ventures
- 00:24:32 Analyzing Market Fit in AI
- 00:25:31 AI Applications with Product Market Fit
- 00:26:31 OpenAI's Impact on the Market
- 00:27:31 Google and OpenAI Competition
- 00:28:31 Exploring Google's Advancements
- 00:29:29 Customer Support and AI Applications
- 00:30:27 The Future of AI in Customer Support
- 00:31:26 Cost-Cutting vs Growth in AI
- 00:32:23 Voice AI and Real-World Applications
- 00:33:23 Scaling AI Applications for Demand
- 00:34:22 Summarization and Conversational AI
- 00:35:20 Future AI Use Cases and Market Fit
- 00:36:20 AI Education and Model Capabilities
- 00:37:17 Reforming Education with AI
- 00:38:15 Defensibility in AI Apps
- 00:39:13 Network Effects and AI
- 00:40:12 AI Brand and Market Positioning
- 00:41:11 AI Application Defensibility
- 00:42:09 LLM OS and AI Infrastructure
- 00:43:06 Security and AI Application
- 00:44:06 OpenAI's Role in AI Infrastructure
- 00:45:02 The Balance of AI Applications and Infrastructure
- 00:46:02 Capital Efficiency in AI Infrastructure
- 00:47:01 Challenges in AI DevOps and Infrastructure
- 00:47:59 AI SRE and Monitoring
- 00:48:59 Scaling AI and Hardware Challenges
- 00:49:58 Reliability and Compute in AI
- 00:50:57 Nvidia's Dominance and AI Hardware
- 00:51:57 Emerging Competition in AI Silicon
- 00:52:54 Agent Authenticatio...
Previous Episode
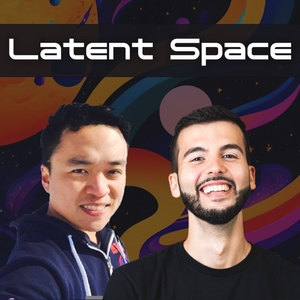
The Agent Network — Dharmesh Shah
If you’re in SF: Join us for the Claude Plays Pokemon hackathon this Sunday!
If you’re not: Fill out the 2025 State of AI Eng survey for $250 in Amazon cards!
We are SO excited to share our conversation with Dharmesh Shah, co-founder of HubSpot and creator of Agent.ai.
A particularly compelling concept we discussed is the idea of "hybrid teams" - the next evolution in workplace organization where human workers collaborate with AI agents as team members. Just as we previously saw hybrid teams emerge in terms of full-time vs. contract workers, or in-office vs. remote workers, Dharmesh predicts that the next frontier will be teams composed of both human and AI members. This raises interesting questions about team dynamics, trust, and how to effectively delegate tasks between human and AI team members.
The discussion of business models in AI reveals an important distinction between Work as a Service (WaaS) and Results as a Service (RaaS), something Dharmesh has written extensively about. While RaaS has gained popularity, particularly in customer support applications where outcomes are easily measurable, Dharmesh argues that this model may be over-indexed. Not all AI applications have clearly definable outcomes or consistent economic value per transaction, making WaaS more appropriate in many cases. This insight is particularly relevant for businesses considering how to monetize AI capabilities.
The technical challenges of implementing effective agent systems are also explored, particularly around memory and authentication. Shah emphasizes the importance of cross-agent memory sharing and the need for more granular control over data access. He envisions a future where users can selectively share parts of their data with different agents, similar to how OAuth works but with much finer control. This points to significant opportunities in developing infrastructure for secure and efficient agent-to-agent communication and data sharing.
Other highlights from our conversation
The Evolution of AI-Powered Agents – Exploring how AI agents have evolved from simple chatbots to sophisticated multi-agent systems, and the role of MCPs in enabling that.
Hybrid Digital Teams and the Future of Work – How AI agents are becoming teammates rather than just tools, and what this means for business operations and knowledge work.
Memory in AI Agents – The importance of persistent memory in AI systems and how shared memory across agents could enhance collaboration and efficiency.
Business Models for AI Agents – Exploring the shift from software as a service (SaaS) to work as a service (WaaS) and results as a service (RaaS), and what this means for monetization.
The Role of Standards Like MCP – Why MCP has been widely adopted and how it enables agent collaboration, tool use, and discovery.
The Future of AI Code Generation and Software Engineering – How AI-assisted coding is changing the role of software engineers and what skills will matter most in the future.
Domain Investing and Efficient Markets – Dharmesh’s approach to domain investing and how inefficiencies in digital asset markets create business opportunities.
The Philosophy of Saying No – Lessons from "Sorry, You Must Pass" and how prioritization leads to greater productivity and focus.
Timestamps
00:00 Introduction and Guest Welcome
02:29 Dharmesh Shah's Journey into AI
05:22 Defining AI Agents
06:45 The Evolution and Future of AI Agents
13:53 Graph Theory and Knowledge Representation
20:02 Engineering Practices and Overengineering
25:57 The Role of Junior Engineers in the AI Era
28:20 Multi-Agent Systems and MCP Standards
35:55 LinkedIn's Legal Battles and Data Scraping
37:32 The Future of AI and Hybrid Teams
39:19 Building Agent AI: A Professional Network for Agents
40:43 Challenges and Innovations in Agent AI
45:02 The Evolution of UI in AI Systems
01:00:25 Business Models: Work as a Service vs. Results as a Service
01:09:17 The Future Value of Engineers
01:09:51 Exploring the Role of Agents
01:10:28 The Importance of Memory in AI
01:11:02 Challenges and Opportunities in AI Memory
01:12:41 Selective Memory and...
Next Episode
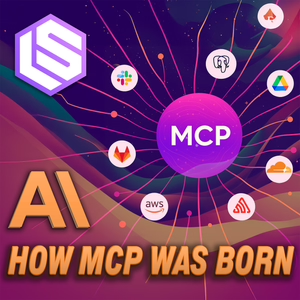
The Creators of Model Context Protocol
Today’s guests, David Soria Parra and Justin Spahr-Summers, are the creators of Anthropic’s Model Context Protocol (MCP). When we first wrote Why MCP Won, we had no idea how quickly it was about to win.
In the past 4 weeks, OpenAI and now Google have now announced the MCP support, effectively confirming our prediction that MCP was the presumptive winner of the agent standard wars. MCP has now overtaken OpenAPI, the incumbent option and most direct alternative, in GitHub stars (3 months ahead of conservative trendline):
For protocol and history nerds, we also asked David and Justin to tell the origin story of MCP, which we leave to the reader to enjoy (you can also skim the transcripts, or, the changelogs of a certain favored IDE). It’s incredible the impact that individual engineers solving their own problems can have on an entire industry.
Timestamps
00:00 Introduction and Guest Welcome
00:37 What is MCP?
02:00 The Origin Story of MCP
05:18 Development Challenges and Solutions
08:06 Technical Details and Inspirations
29:45 MCP vs Open API
32:48 Building MCP Servers
40:39 Exploring Model Independence in LLMs
41:36 Building Richer Systems with MCP
43:13 Understanding Agents in MCP
45:45 Nesting and Tool Confusion in MCP
49:11 Client Control and Tool Invocation
52:08 Authorization and Trust in MCP Servers
01:01:34 Future Roadmap and Stateless Servers
01:10:07 Open Source Governance and Community Involvement
01:18:12 Wishlist and Closing Remarks
If you like this episode you’ll love
Episode Comments
Generate a badge
Get a badge for your website that links back to this episode
<a href="https://goodpods.com/podcasts/latent-space-the-ai-engineer-podcast-262472/unsupervised-learning-x-latent-space-crossover-special-89819470"> <img src="https://storage.googleapis.com/goodpods-images-bucket/badges/generic-badge-1.svg" alt="listen to unsupervised learning x latent space crossover special on goodpods" style="width: 225px" /> </a>
Copy