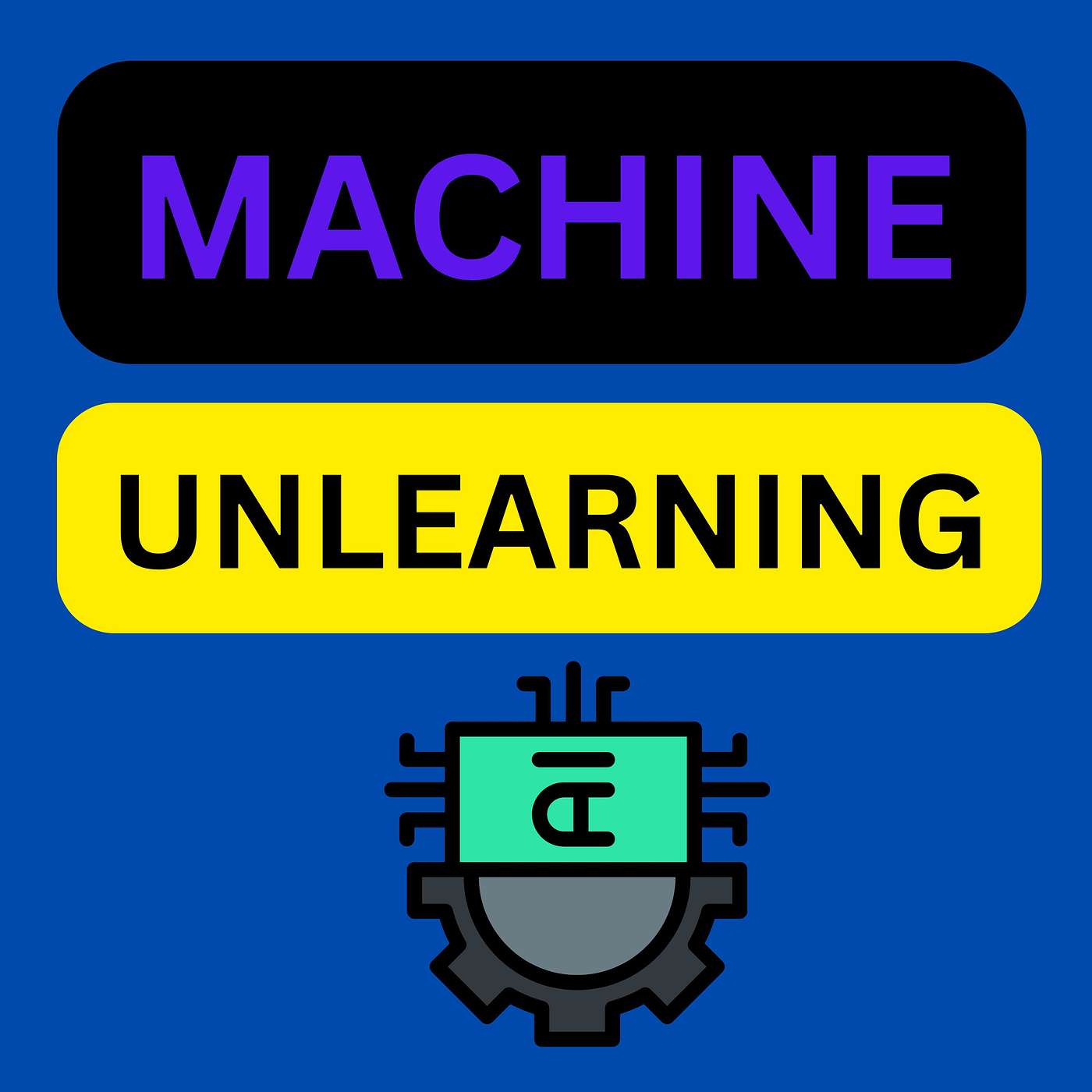
Machine Unlearning for Tech Startups
01/14/25 • 20 min
6 Listeners
Machine unlearning is a crucial technology allowing the selective removal of data from AI models without complete retraining. The text explores various techniques for machine unlearning, including exact, approximate, prompt-based, and decentralized methods, each with advantages and disadvantages for tech startups. These methods offer benefits like regulatory compliance, enhanced user trust, and mitigation of data risks. The text emphasizes the importance of integrating machine unlearning early in the development process and prioritizing transparency to foster responsible AI innovation. Startups can gain a competitive edge by embracing this technology and addressing growing privacy concerns.
Podcast:
https://kabir.buzzsprout.com
YouTube:
https://www.youtube.com/@kabirtechdives
Please subscribe and share.
Machine unlearning is a crucial technology allowing the selective removal of data from AI models without complete retraining. The text explores various techniques for machine unlearning, including exact, approximate, prompt-based, and decentralized methods, each with advantages and disadvantages for tech startups. These methods offer benefits like regulatory compliance, enhanced user trust, and mitigation of data risks. The text emphasizes the importance of integrating machine unlearning early in the development process and prioritizing transparency to foster responsible AI innovation. Startups can gain a competitive edge by embracing this technology and addressing growing privacy concerns.
Podcast:
https://kabir.buzzsprout.com
YouTube:
https://www.youtube.com/@kabirtechdives
Please subscribe and share.
Previous Episode
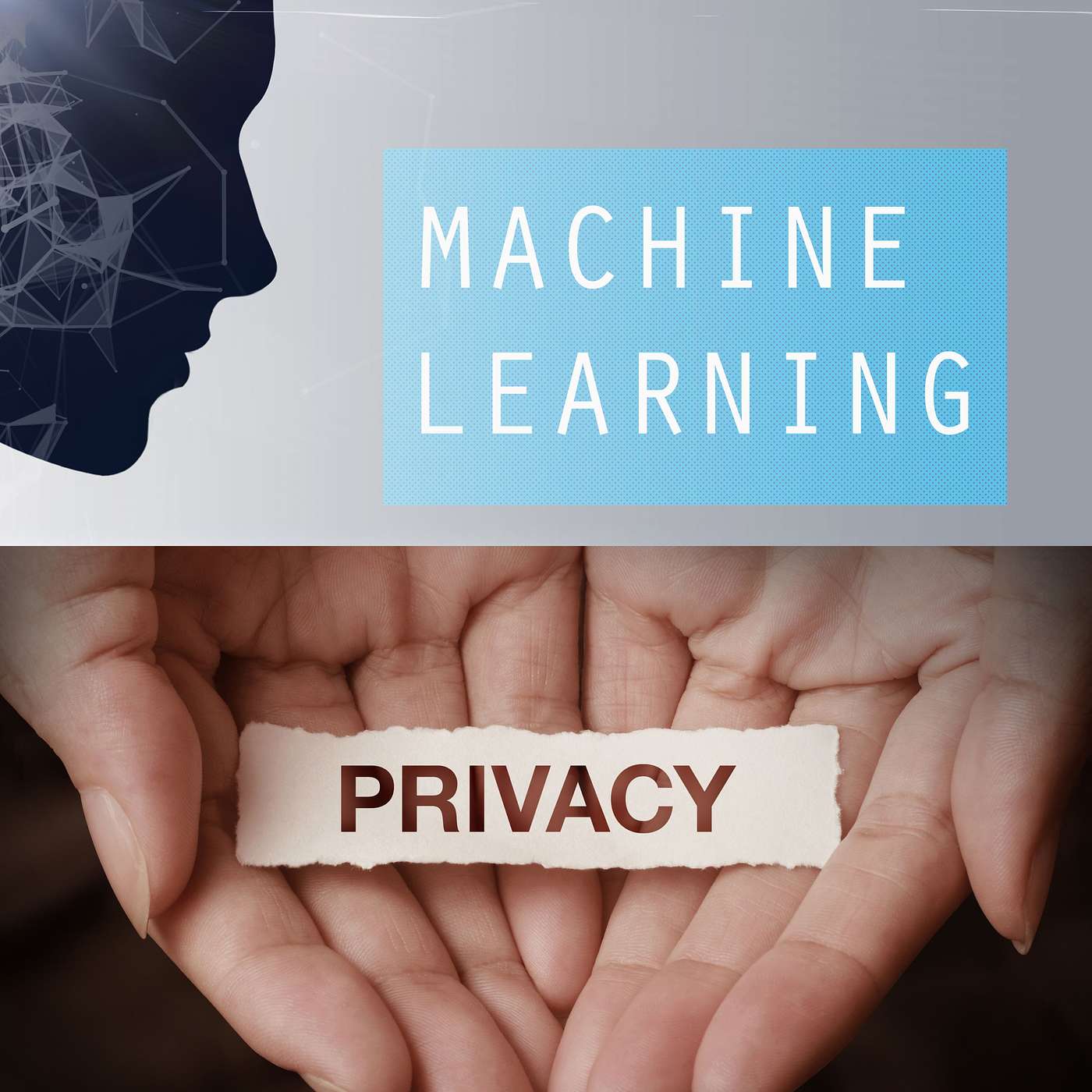
Privacy-First AI in 2025
The episode discusses the increasing importance of privacy in the field of artificial intelligence (AI) and machine learning (ML) in 2025. It highlights privacy-preserving techniques like Differential Privacy, Federated Learning, Zero-Knowledge Machine Learning, and Fully Homomorphic Encryption as crucial tools for startups aiming to develop ethical and responsible AI. The text emphasizes that prioritizing user privacy is not just a regulatory requirement but a significant competitive advantage. It concludes that startups integrating these privacy-first methods will be best positioned for success in the future of AI.
Podcast:
https://kabir.buzzsprout.com
YouTube:
https://www.youtube.com/@kabirtechdives
Please subscribe and share.
Next Episode
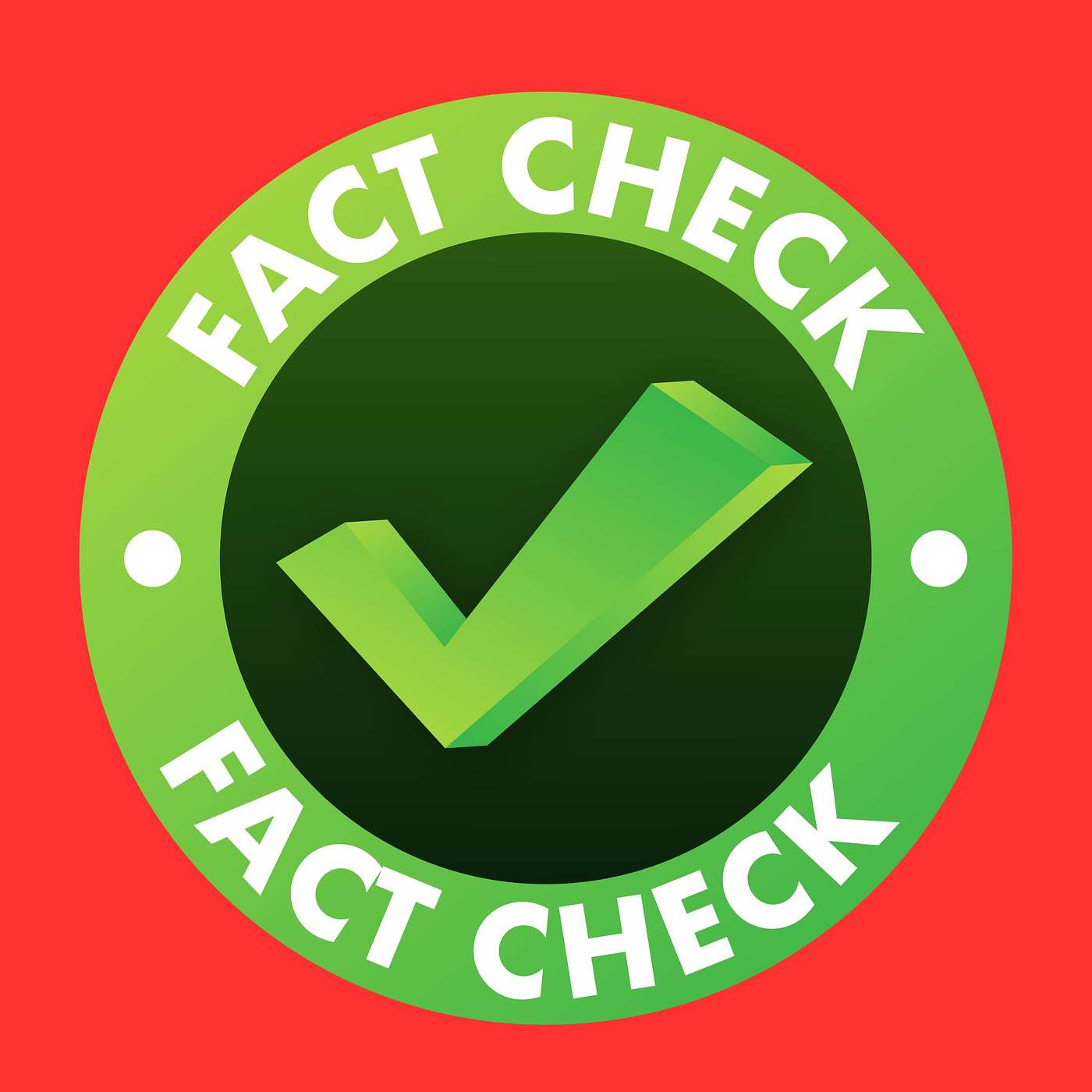
AI Fact Checking | Future of Truth Online Using
This episode is about Search-Augmented Factuality Evaluator (SAFE), a novel, cost-effective method for automatically evaluating the factuality of long-form text generated by large language models (LLMs). SAFE leverages LLMs and Google Search to assess the accuracy of individual facts within a response, outperforming human annotators in accuracy and efficiency. The researchers also created LongFact, a new benchmark dataset of 2,280 prompts designed to test long-form factuality across diverse topics, and proposed F1@K, a new metric that incorporates both precision and recall, accounting for the desired length of a factual response. Extensive benchmarking across thirteen LLMs demonstrates that larger models generally exhibit higher factuality, and the paper thoroughly addresses reproducibility and ethical considerations.
Podcast:
https://kabir.buzzsprout.com
YouTube:
https://www.youtube.com/@kabirtechdives
Please subscribe and share.
If you like this episode you’ll love
Episode Comments
Generate a badge
Get a badge for your website that links back to this episode
<a href="https://goodpods.com/podcasts/kabirs-tech-dives-629055/machine-unlearning-for-tech-startups-81806295"> <img src="https://storage.googleapis.com/goodpods-images-bucket/badges/generic-badge-1.svg" alt="listen to machine unlearning for tech startups on goodpods" style="width: 225px" /> </a>
Copy