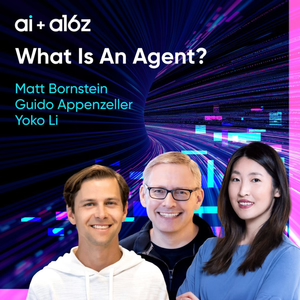
What Is an AI Agent?
04/28/25 • 36 min
In this episode of AI + a16z, a16z Infra partners Guido Appenzeller, Matt Bornstein, and Yoko Li discuss and debate one of the tech industry's buzziest words right now: AI agents. The trio digs into the topic from a number of angles, including:
- Whether a uniform definition of agent actually exists
- How to distinguish between agents, LLMs, and functions
- How to think about pricing agents
- Whether agents can actually replace humans, and
- The effects of data siloes on agents that can access the web.
They don't claim to have all the answers, but they raise many questions and insights that should interest anybody building, buying, and even marketing AI agents.
Learn more:
Benchmarking AI Agents on Full-Stack Coding
Automating Developer Email with MCP and Al Agents
A Deep Dive Into MCP and the Future of AI Tooling
Agent Experience: Building an Open Web for the AI Era
DeepSeek, Reasoning Models, and the Future of LLMs
Reasoning Models Are Remaking Professional Services
From NLP to LLMs: The Quest for a Reliable Chatbot
Can AI Agents Finally Fix Customer Support?
Follow everybody on X:
Check out everything a16z is doing with artificial intelligence here, including articles, projects, and more podcasts.
In this episode of AI + a16z, a16z Infra partners Guido Appenzeller, Matt Bornstein, and Yoko Li discuss and debate one of the tech industry's buzziest words right now: AI agents. The trio digs into the topic from a number of angles, including:
- Whether a uniform definition of agent actually exists
- How to distinguish between agents, LLMs, and functions
- How to think about pricing agents
- Whether agents can actually replace humans, and
- The effects of data siloes on agents that can access the web.
They don't claim to have all the answers, but they raise many questions and insights that should interest anybody building, buying, and even marketing AI agents.
Learn more:
Benchmarking AI Agents on Full-Stack Coding
Automating Developer Email with MCP and Al Agents
A Deep Dive Into MCP and the Future of AI Tooling
Agent Experience: Building an Open Web for the AI Era
DeepSeek, Reasoning Models, and the Future of LLMs
Reasoning Models Are Remaking Professional Services
From NLP to LLMs: The Quest for a Reliable Chatbot
Can AI Agents Finally Fix Customer Support?
Follow everybody on X:
Check out everything a16z is doing with artificial intelligence here, including articles, projects, and more podcasts.
Previous Episode
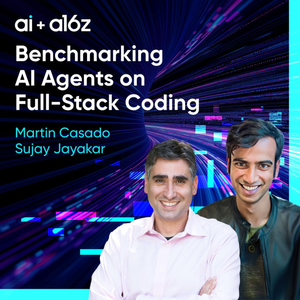
Benchmarking AI Agents on Full-Stack Coding
In this episode, a16z General Partner Martin Casado sits down with Sujay Jayakar, co-founder and Chief Scientist at Convex, to talk about his team’s latest work benchmarking AI agents on full-stack coding tasks. From designing Fullstack Bench to the quirks of agent behavior, the two dig into what’s actually hard about autonomous software development, and why robust evals—and guardrails like type safety—matter more than ever. They also get tactical: which models perform best for real-world app building? How should developers think about trajectory management and variance across runs? And what changes when you treat your toolchain like part of the prompt? Whether you're a hobbyist developer or building the next generation of AI-powered devtools, Sujay’s systems-level insights are not to be missed.
Drawing from Sujay’s work developing the Fullstack-Bench, they cover:
- Why full-stack coding is still a frontier task for autonomous agents
- How type safety and other “guardrails” can significantly reduce variance and failure
- What makes a good eval—and why evals might matter more than clever prompts
- How different models perform on real-world app-building tasks (and what to watch out for)
- Why your toolchain might be the most underrated part of the prompt
- And what all of this means for devs—from hobbyists to infra teams building with AI in the loop
Learn More:
Follow everyone on X:
Check out everything a16z is doing with artificial intelligence here, including articles, projects, and more podcasts.
Next Episode
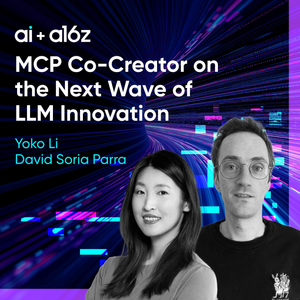
MCP Co-Creator on the Next Wave of LLM Innovation
In this episode of AI + a16z, Anthropic's David Soria Parra — who created MCP (Model Context Protocol) along with Justin Spahr-Summers — sits down with a16z's Yoko Li to discuss the project's inception, exciting use cases for connecting LLMs to external sources, and what's coming next for the project. If you're unfamiliar with the wildly popular MCP project, this edited passage from their discussion is a great starting point to learn:
David: "MCP tries to enable building AI applications in such a way that they can be extended by everyone else that is not part of the original development team through these MCP servers, and really bring the workflows you care about, the things you want to do, to these AI applications. It's a protocol that just defines how whatever you are building as a developer for that integration piece, and that AI application, talk to each other.
"It's a very boring specification, but what it enables is hopefully ... something that looks like the current API ecosystem, but for LLM interactions."
Yoko: "I really love the analogy with the API ecosystem, because they give people a mental model of how the ecosystem evolves ... Before, you may have needed a different spec to query Salesforce versus query HubSpot. Now you can use similarly defined API schema to do that.
"And then when I saw MCP earlier in the year, it was very interesting in that it almost felt like a standard interface for the agent to interface with LLMs. It's like, 'What are the set of things that the agent wants to execute on that it has never seen before? What kind of context does it need to make these things happen?' When I tried it out, it was just super powerful and I no longer have to build one tool per client. I now can build just one MCP server, for example, for sending emails, and I use it for everything on Cursor, on Claude Desktop, on Goose."
Learn more:
A Deep Dive Into MCP and the Future of AI Tooling
Benchmarking AI Agents on Full-Stack Coding
Agent Experience: Building an Open Web for the AI Era
Follow everyone on X:
Check out everything a16z is doing with artificial intelligence here, including articles, projects, and more podcasts.
If you like this episode you’ll love
Episode Comments
Generate a badge
Get a badge for your website that links back to this episode
<a href="https://goodpods.com/podcasts/ai-a16z-379277/what-is-an-ai-agent-90146265"> <img src="https://storage.googleapis.com/goodpods-images-bucket/badges/generic-badge-1.svg" alt="listen to what is an ai agent? on goodpods" style="width: 225px" /> </a>
Copy