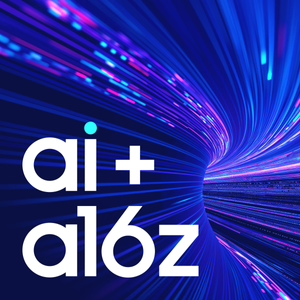
AI + a16z
a16z
2 Listeners
All episodes
Best episodes
Top 10 AI + a16z Episodes
Goodpods has curated a list of the 10 best AI + a16z episodes, ranked by the number of listens and likes each episode have garnered from our listeners. If you are listening to AI + a16z for the first time, there's no better place to start than with one of these standout episodes. If you are a fan of the show, vote for your favorite AI + a16z episode by adding your comments to the episode page.
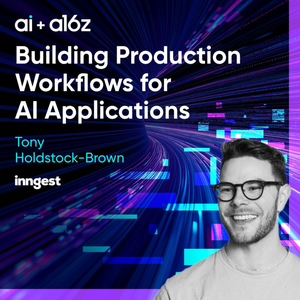
06/14/24 • 43 min
In this episode, Inngest cofounder and CEO Tony Holdstock-Brown joins a16z partner Yoko Li, as well as Derrick Harris, to discuss the reality and complexity of running AI agents and other multistep AI workflows in production. Tony also why developer tools for generative AI — and their founders — might look very similar to previous generations of these products, and where there are opportunities for improvement.
Here's a sample of the discussion, where Tony shares some advice for engineers looking to build for AI:
"We almost have two parallel tracks right now as, as engineers. We've got the CPU track in which we're all like, 'Oh yeah, CPU-bound, big O notation. What are we doing on the application-level side?' And then we've got the GPU side, in which people are doing like crazy things in order to make numbers faster, in order to make differentiation better and smoother, in order to do gradient descent in a nicer and more powerful way. The two disciplines right now are working together, but are also very, very, very different from an engineering point of view.
"This is one interesting part to think about for like new engineers, people that are just thinking about what to do if they want to go into the engineering field overall. Do you want to be on the side using AI, in which you take all of these models, do all of this stuff, build the application-level stuff, and chain things together to build products? Or do you want to be on the math side of things, in which you do really low-level things in order to make compilers work better, so that your AI things can run faster and more efficiently? Both are engineering, just completely different applications of it."
Learn more:
The Modern Transactional Stack
Follow everyone on X:
Check out everything a16z is doing with artificial intelligence here, including articles, projects, and more podcasts.
1 Listener
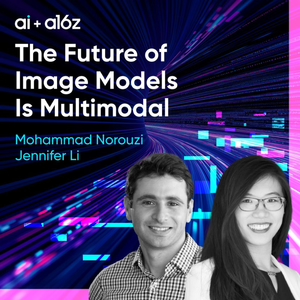
06/07/24 • 37 min
In this episode, Ideogram CEO Mohammad Norouzi joins a16z General Partner Jennifer Li, as well as Derrick Harris, to share his story of growing up in Iran, helping build influential text-to-image models at Google, and ultimately cofounding and running Ideogram. He also breaks down the differences between transformer models and diffusion models, as well as the transition from researcher to startup CEO.
Here's an excerpt where Mohammad discusses the reaction to the original transformer architecture paper, "Attention Is All You Need," within Google's AI team:
"I think [lead author Asish Vaswani] knew right after the paper was submitted that this is a very important piece of the technology. And he was telling me in the hallway how it works and how much improvement it gives to translation. Translation was a testbed for the transformer paper at the time, and it helped in two ways. One is the speed of training and the other is the quality of translation.
"To be fair, I don't think anybody had a very crystal clear idea of how big this would become. And I guess the interesting thing is, now, it's the founding architecture for computer vision, too, not only for language. And then we also went far beyond language translation as a task, and we are talking about general-purpose assistants and the idea of building general-purpose intelligent machines. And it's really humbling to see how big of a role the transformer is playing into this."
Learn more:
Investing in Ideogram
Denoising Diffusion Probabilistic Models
Follow everyone on X:
Check out everything a16z is doing with artificial intelligence here, including articles, projects, and more podcasts.
1 Listener
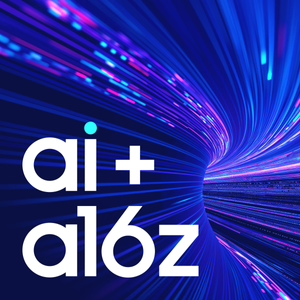
07/05/24 • 33 min
In this archive episode from 2015, a16z's Sonal Chokshi, Frank Chen, and Steven Sinofsky discuss DeepMind's breakthrough AlphaGo system, which mastered the ancient Chinese game Go and introduced the public to reinforcement learning.
Check out everything a16z is doing with artificial intelligence here, including articles, projects, and more podcasts.
1 Listener
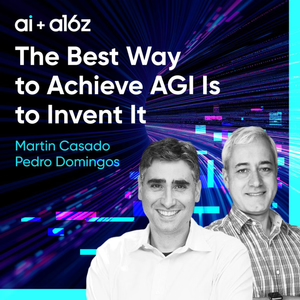
11/04/24 • 38 min
Longtime machine-learning researcher, and University of Washington Professor Emeritus, Pedro Domingos joins a16z General Partner Martin Casado to discuss the state of artificial intelligence, whether we're really on a path toward AGI, and the value of expressing unpopular opinions. It's a very insightful discussion as we head into an era of mainstream AI adoption, and ask big questions about how to ramp up progress and diversify research directions.
Here's an excerpt of Pedro sharing his thoughts on the increasing cost of frontier models and whether that's the right direction:
"if you believe the scaling laws hold and the scaling laws will take us to human-level intelligence, then, hey, it's worth a lot of investment. That's one part, but that may be wrong. The other part, however, is that to do that, we need exploding amounts of compute.
"If if I had to predict what's going to happen, it's that we do not need a trillion dollars to reach AGI at all. So if you spend a trillion dollars reaching AGI, this is a very bad investment."
Learn more:
The Economic Case for Generative AI and Foundation Models
Follow everyone on Z:
Check out everything a16z is doing with artificial intelligence here, including articles, projects, and more podcasts.
1 Listener
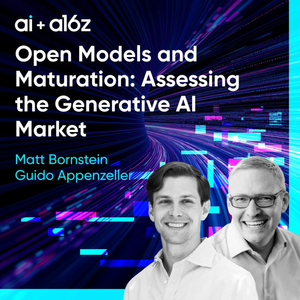
05/17/24 • 40 min
a16z partners Guido Appenzeller and Matt Bornstein join Derrick Harris to discuss the state of the generative AI market, about 18 months after it really kicked into high gear with the release of ChatGPT — everything from the emergence of powerful open source LLMs to the excitement around AI-generated music.
If there's one major lesson to learn, it's that although we've made some very impressive technological strides and companies are generating meaningful revenue, this is still a a very fluid space. As Matt puts it during the discussion:
"For nearly all AI applications and most model providers, growth is kind of a sawtooth pattern, meaning when there's a big new amazing thing announced, you see very fast growth. And when it's been a while since the last release, growth kind of can flatten off. And you can imagine retention can be all over the place, too . . .
"I think every time we're in a flat period, people start to think, 'Oh, it's mature now, the, the gold rush is over. What happens next?' But then a new spike almost always comes, or at least has over the last 18 months or so. So a lot of this depends on your time horizon, and I think we're still in this period of, like, if you think growth has slowed, wait a month and see it change."
Follow everyone on X:
Check out everything a16z is doing with artificial intelligence here, including articles, projects, and more podcasts.
1 Listener
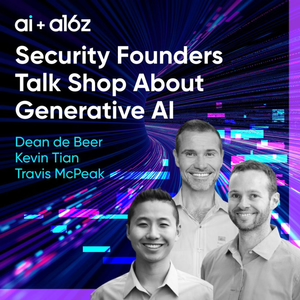
05/15/24 • 22 min
In this bonus episode, recorded live at our San Francisco office, security-startup founders Dean De Beer (Command Zero), Kevin Tian (Doppel), and Travis McPeak (Resourcely) share their thoughts on generative AI, as well as their experiences building with LLMs and dealing with LLM-based threats.
Here's a sample of what Dean had to say about the myriad considerations when choosing, and operating, a large language model:
"The more advanced your use case is, the more requirements you have, the more data you attach to it, the more complex your prompts — ll this is going to change your inference time.
"I liken this to perceived waiting time for an elevator. There's data scientists at places like Otis that actually work on that problem. You know, no one wants to wait 45 seconds for an elevator, but taking the stairs will take them half an hour if they're going to the top floor of . . . something. Same thing here: If I can generate an outcome in 90 seconds, it's still too long from the user's perspective, even if them building out and figuring out the data and building that report [would have] took them four hours . . . two days."
Follow everyone:
Check out everything a16z is doing with artificial intelligence here, including articles, projects, and more podcasts.
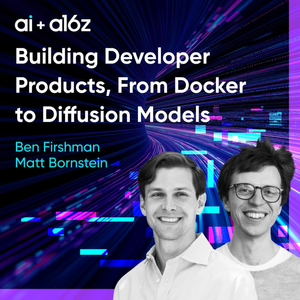
11/15/24 • 41 min
In this episode of AI + a16z, Replicate cofounder and CEO Ben Firshman, and a16z partner Matt Bornstein, discuss the art of building products and companies that appeal to software developers. Ben was the creator of Docker Compose, and Replicate has a thriving community of developers hosting and fine-tuning their own models to power AI-based applications.
Here's an excerpt of Ben and Matt discussing the difference in the variety of applications built using multimedia models compared with language models:
Matt: "I've noticed there's a lot of really diverse multimedia AI apps out there. Meaning that when you give someone an amazing primitive, like a FLUX API call or a Stable Diffusion API call, and Replicate, there's so many things they can do with it. And we actually see that happening — versus with language, where all LLM apps look kind of the same if you squint a little bit.
"It's like you chat with something — there's obviously code, there's language, there's a few different things — but I've been surprised that even today we don't see as many apps built on language models as we do based on, say, image models."
Ben: "It certainly maps with what we're seeing, as well. I think these language models, beyond just chat apps, are particularly good at turning unstructured information into structured information. Which is actually kind of magical. And computers haven't been very good at that before. That is really a kind of cool use case for it.
"But with these image models and video models and things like that, people are creating lots of new products that were not possible before — things that were just impossible for computers to do. So yeah, I'm certainly more excited by all the magical things these multimedia models can make."
"But with these image models and video models and things like that, people are creating lots of new products that were just not possible before — things that were just impossible for computers to do. So yeah, I'm certainly more excited by all the magical things these multimedia models can make."
Follow everyone on X:
Learn more:
Check out everything a16z is doing with artificial intelligence here, including articles, projects, and more podcasts.
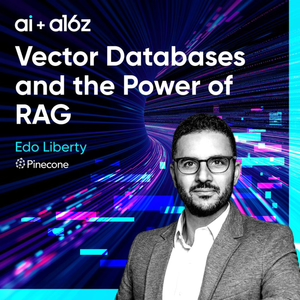
04/26/24 • 36 min
Pinecone Founder and CEO Edo Liberty joins a16z's Satish Talluri and Derrick Harris to discuss the promises, challenges, and opportunities for vector databases and retrieval augmented generation (RAG). He also shares insights and highlights from a decades-long career in machine learning, which includes stints running research teams at both Yahoo and Amazon Web Services.
Because he's been at this a long time, and despite its utility, Edo understands that RAG — like most of today's popular AI concepts — is still very much a progress:
"I think RAG today is where transformers were in 2017. It's clunky and weird and hard to get right. And it has a lot of sharp edges, but it already does something amazing. Sometimes, most of the time, the very early adopters and the very advanced users are already picking it up and running with it and lovingly deal with all the sharp edges ...
"Making progress on RAG, making progress on information retrieval, and making progress on making AI more knowledgeable and less hallucinatory and more dependable, is a complete greenfield today. There's an infinite amount of innovation that will have to go into it."
More about Pinecone and RAG:
Retrieval Augmented Generation (RAG)
Emerging Architectures for LLM Applications
Follow everyone on X:
Check out everything a16z is doing with artificial intelligence here, including articles, projects, and more podcasts.
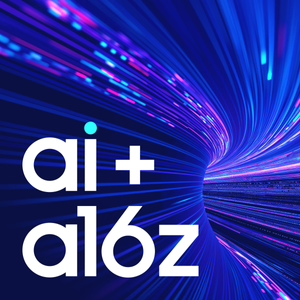
05/24/24 • 65 min
For this holiday weekend (in the United States) episode, we've stitched together two archived episodes from the a16z Podcast, both featuring General Partner Anjney Midha. In the first half, from December, he speaks with Mistral cofounder and CEO Arthur Mensch about the importance of open foundation models, as well as Mistral's approach to building them. In the second half (at 34:40), from February, he speaks with Stanford's Stefano Ermon about the state of the art in video models, including how OpenAI's Sora might work under the hood.
Here's a sample of what Arthur had to say about the debate over how to regulate AI models:
"I think the battle is for the neutrality of the technology. Like a technology, by a sense, is something neutral. You can use it for bad purposes. You can use it for good purposes. If you look at what an LLM does, it's not really different from a programming language. . . .
"So we should regulate the function, the mathematics behind it. But, really, you never use a large language model itself. You always use it in an application, in a way, with a user interface. And so, that's the one thing you want to regulate. And what it means is that companies like us, like foundational model companies, will obviously make the model as controllable as possible so that the applications on top of it can be compliant, can be safe. We'll also build the tools that allow you to measure the compliance and the safety of the application, because that's super useful for the application makers. It's actually needed.
"But there's no point in regulating something that is neutral in itself, that is just a mathematical tool. I think that's the one thing that we've been hammering a lot, which is good, but there's still a lot of effort in making this strong distinction, which is super important to understand what's going on."
Follow everyone on X:
Check out everything a16z is doing with artificial intelligence here, including articles, projects, and more podcasts.
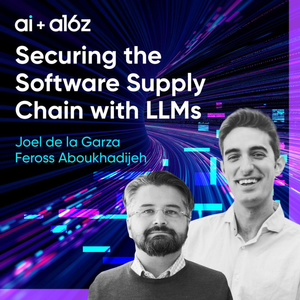
05/03/24 • 38 min
Socket Founder and CEO Feross Aboukhadijeh joins a16z's Joel de la Garza and Derrick Harris to discuss the open-source software supply chain. Feross and Joel share their thoughts and insights on topics ranging from the recent XZutils attack to how large language models can help overcome understaffed security teams and overwhelmed developers.
Despite some increasingly sophisticated attacks making headlines and compromising countless systems, they're optimistic that LLMs, in particular, could be a turning point for security blue teams. As Feross sums up one possibility:
"The way we think about gen AI on the defensive side is that it's not as good as a human looking at the code, but it's something. . . . Our challenge is that we want to scan all the open source code that exists out there. That is not something you can pay humans to do. That is not scalable at all. But, with the right techniques, with the right pre-filtering stages, you can actually put a lot of that stuff through LLMs and out the other side will pop a list of of risky packages.
"And then that's a much smaller number that you can have humans take a look at. And so we're using it as a tool . . . to find the needle in the haystack, what is worth looking at. It's not perfect, but it can help cut down on the noise and it can even make this problem tractable, which previously wasn't even tractable."
More about Socket and cybersecurity:
Follow everyone :
Check out everything a16z is doing with artificial intelligence here, including articles, projects, and more podcasts.
Show more best episodes

Show more best episodes
FAQ
How many episodes does AI + a16z have?
AI + a16z currently has 28 episodes available.
What topics does AI + a16z cover?
The podcast is about Venture Capital, Entrepreneurship, Startups, Podcasts, Technology, Business, Artificial Intelligence and Machine Learning.
What is the most popular episode on AI + a16z?
The episode title 'Building Production Workflows for AI Applications' is the most popular.
What is the average episode length on AI + a16z?
The average episode length on AI + a16z is 40 minutes.
How often are episodes of AI + a16z released?
Episodes of AI + a16z are typically released every 7 days.
When was the first episode of AI + a16z?
The first episode of AI + a16z was released on Apr 8, 2024.
Show more FAQ

Show more FAQ