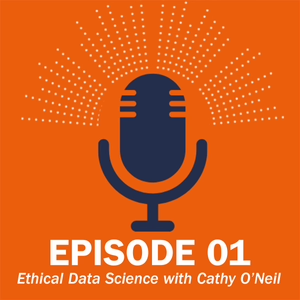
UVA Data Points sits down with Cathy O'Neil, author of Weapons of Math Destruction, and Brian Wright, Assistant Professor of Data Science at the University of Virginia. The candid dialogue ranges from O'Neil's new book The Shame Machine to her work as an algorithm audit consultant. The two also draw comparisons between data science problems and knitting, as well as discuss educating future data scientists.
Links:
https://mathbabe.org (Cathy O'Neil's website)
https://datascience.virginia.edu (UVA School of Data Science website)
Books mentioned:
09/01/22 • 27 min
Generate a badge
Get a badge for your website that links back to this episode
<a href="https://goodpods.com/podcasts/uva-data-points-247993/ethical-data-science-with-cathy-oneil-28253834"> <img src="https://storage.googleapis.com/goodpods-images-bucket/badges/generic-badge-1.svg" alt="listen to ethical data science with cathy o'neil on goodpods" style="width: 225px" /> </a>
Copy