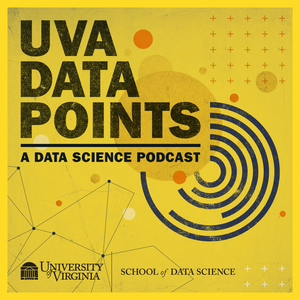
UVA Data Points
UVA School of Data Science
1 Creator
1 Creator
1 Listener
All episodes
Best episodes
Seasons
Top 10 UVA Data Points Episodes
Goodpods has curated a list of the 10 best UVA Data Points episodes, ranked by the number of listens and likes each episode have garnered from our listeners. If you are listening to UVA Data Points for the first time, there's no better place to start than with one of these standout episodes. If you are a fan of the show, vote for your favorite UVA Data Points episode by adding your comments to the episode page.
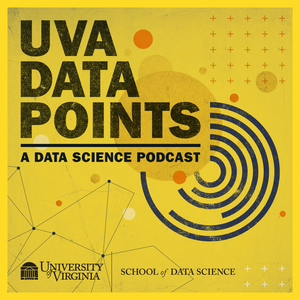
12/01/23 • 58 min
Artificial intelligence has the potential to change our societies, economies, and political systems in both intentional and unintended ways. While it is difficult to understand the full extent of what the long-term impacts may be, we have enough shared knowledge and expertise to predict the likely shapes that these changes may take—both for better and for worse. More importantly, we should ask ourselves what kind of future we want AI to help us create: what we want from the future of AI should ultimately determine the future of AI. This panel will bring together experts to discuss the intersection of AI and society and offer suggestions for how AI might work within a just, inclusive, sustainable, and fair digital future.
Panelist
- Farhana Faruqe, Assistant Professor of Data Science
- Sarah Lebovitz, Assistant Professor of Commerce
- Larry Medsker, Research Professor, George Washington University
- Mar Hicks, Associate Professor of Data Science (moderator)
1 Listener
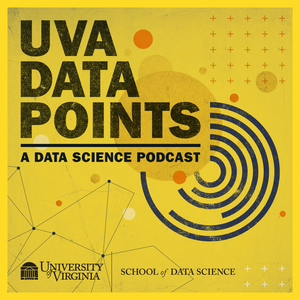
Surviving the Data Deluge
UVA Data Points
02/20/25 • 36 min
One of the most pressing challenges in our increasingly data-driven world is the Data Deluge—the overwhelming flood of information that we generate and record every single day. With us are three experts from the University of Virginia’s School of Data Science. Phil Bourne, a professor of biomedical engineering and the founding dean of the school of data science, is joined by Terence Johnson and Alex Gates, both assistant professors of data science. Together, they have been exploring innovative methods to make sense of the vast oceans of data we’re all swimming in.
This episode unpacks the challenges of the data deluge—what it means for businesses, researchers, and society at large—and explore the strategies we can use to navigate it. How do we make sense of so much information? How do we ensure the ethical use of this data? And what opportunities does this overwhelming flood of data open up for the future?
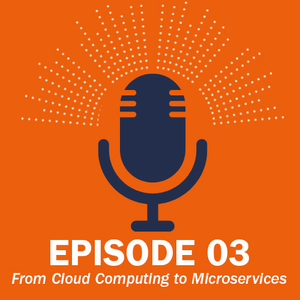
Reshaping Cloud Platforms with Microservices
UVA Data Points
11/01/22 • 41 min
This episode on Systems explores the challenges of cloud computing within the framework of biomedical research. Phil Bourne, Dean of the UVA School of Data Science, speaks with computational biologist and associate professor Nathan Sheffield about a paper they co-wrote on systemic issues from cloud platforms that do not support FAIRness, including platform lock-in, poor integration across platforms, and duplicated efforts for users and developers. They suggest instead prioritizing microservices and access to modular data in smaller chunks or summarized form. Emphasizing modularity and interoperability would lead to a more powerful Unix-like ecosystem of web services for biomedical analysis and data retrieval. The two discuss how funders, developers, and researchers can support microservices as the next generation of cloud-based bioinformatics.
From Cloud Computing to Microservices: Next Steps in FAIR Data and Analysis
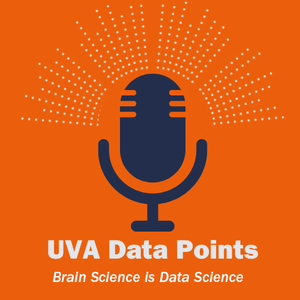
Brain Science is Data Science
UVA Data Points
03/01/23 • 45 min
This episode explores the intersection of neuroscience and data science with three experts in the field, Drs. John Darrell van Horn, Tanya Evans, and Teague Henry. As we know, the brain is complicated. People have been charting paths through the brain for decades, making breakthroughs and discoveries that have changed the world. In recent years though, new methodologies in brain research have made significant impacts. Advances in computing power, as well as techniques like machine learning, neural networks, and computer vision, have allowed researchers to ask questions and make discoveries that were not possible even ten years ago. Given these new approaches to studying the world’s most complicated organ, one could say that brain science is data science. Our guests make a compelling case.
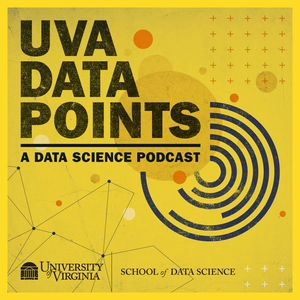
¡Viva la Ciencia de Datos en UVA!
UVA Data Points
11/14/24 • 49 min
Data science is an incredibly diverse and global field of study and practice. In order to tackle some of our most challenging issues ranging from climate change to cognition, we need data and data scientists from all over the world to make advances in research, technology and innovation. To talk about their research interests and the importance of having diverse, global perspectives in the field of data science, this episode of UVA Data Points features a conversation by Javier Rasero, Assistant Professor of Data Science, and two University of Virginia data science students: Marco Gutiérrez Chavezis a first-year Ph.D. student from Peru and Mercedes Mora-Figueroa de Liñán is an M.S. in Data Science student from Spain.
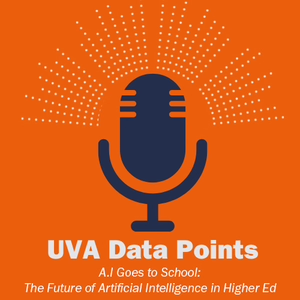
06/20/23 • 40 min
In this episode we’re looking at the past, present, and future of artificial intelligence in higher education.
To explore this topics we’re featuring a conversation between Phil Bourne, the dean of the UVA School of Data Science, and Jeffrey Blume, the Associate Dean for Academic and Faculty Affairs, also at UVA Data Science.
Jeffrey and Phil discuss the recent trends in artificial intelligence and they look at how this will impact the student experience, the faculty and staff experience, and the research landscape in higher education.
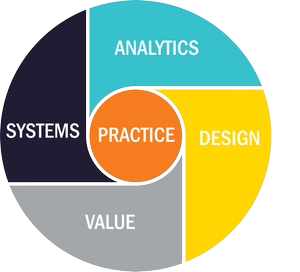
4 + 1 Model of Data Science
UVA Data Points
08/24/22 • 8 min
Before diving into the complex world of data science it seemed to wise to establish a shared definition of the field. Here at the UVA School of Data Science, we have defined data science with the 4 + 1 Model. This model serves an outline for the first series of UVA Data Points. It also serves as a guiding definition within the School of Data Science, touching everything from research to course planning.
In this introduction trailer, host Monica Manney discusses the history, development, and function of the 4 + 1 Model of Data Science with its main author, Raf Alvarado.
Below is a brief expect from An Outline of the 4 + 1 Model of Data Science by Raf Alvarado:
“The point of the 4 + 1 model, abstract as it is, is to provide a practical template for strategically planning the various elements of a school of data science. To serve as an effective template, a model must be general. But generality if often purchased at the cost of intuitive understanding. The following caveats may help make sense of the model when considering its usefulness when applied to various concrete activities.
The model describes areas of academic expertise, not objective reality. It is a map of a division of labor writ large. Although each of the areas has clear connections to the others, the question to ask when deciding where an activity belongs is: who would be an expert at doing it? The realms help refine this question: the analytics area, for example, contains people who are good at working with abstract machinery. The four areas have the virtue of isolating intuitively correct communities of expertise. For example, people who are great at data product design may not know the esoteric depths of machine learning, and that adepts at machine learning are not usually experts in understanding human society and normative culture.
Each area in the model contains a collection of subfields that need to be teased out. Some areas will have more subfields than others. Although some areas may be smaller than others in terms of number of experts (faculty) and courses, each area has a major impact on the overall practice of data science and the quality of the school’s activities. In addition, these subfields are in an important sense “more real” than the categories. We can imagine them forming a dense network in which the areas define communities with centroids, and which are more interconnected than the clean-cut image of the model implies.
The areas of the model are like the components of a principal component analysis of the vector space of data science. They capture the variance that exists within the field, and, crucially, provide a framework for realigning (rebasing) the academy along a new set of axes. One effect of this is to both disperse and recombine older fields, such as computer science, statistics, and operations research, into new clusters. Thus we separate computer science subfields such as complexity analysis and database design. One possible salutary result of this will be the formation of new syntheses of fields that share concerns but differ in vocabularies and customs..."
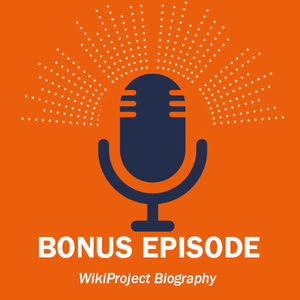
WikiProject Biography
UVA Data Points
11/21/22 • 36 min
This bonus episode features a conversation between Lane Rasberry, Wikimedian-In-Residence at the UVA School of Data Science, and Lloyd Sy, a Ph.D. candidate in the UVA Department of English. In this conversation, Lane and Lloyd take a deep dive into the expansive world of Wikidata and ask the existential question, "What makes a person a person?" Or, more specifically, what data points make up a person? To help answer this question, Lloyd developed a large-scale data model of the biographical data contained within the Wikidata platform. This project serves as the foundation for their conversation. They also take a wide view of biographical data as it pertains to research and academia, including the process of gathering the data, the ethics of utilizing the data, personal ownership of the data, and much more. Anyone interested in these concepts should find this discussion valuable.
Links:
Music:
"Screen Saver" Kevin MacLeod (incompetech.com)
Licensed under Creative Commons: By Attribution 4.0 License
http://creativecommons.org/licenses/by/4.0/
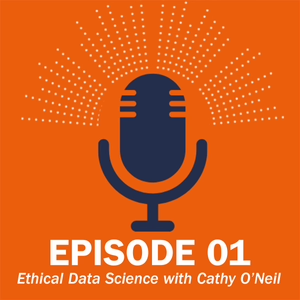
Ethical Data Science with Cathy O'Neil
UVA Data Points
09/01/22 • 27 min
UVA Data Points sits down with Cathy O'Neil, author of Weapons of Math Destruction, and Brian Wright, Assistant Professor of Data Science at the University of Virginia. The candid dialogue ranges from O'Neil's new book The Shame Machine to her work as an algorithm audit consultant. The two also draw comparisons between data science problems and knitting, as well as discuss educating future data scientists.
Links:
https://mathbabe.org (Cathy O'Neil's website)
https://datascience.virginia.edu (UVA School of Data Science website)
Books mentioned:
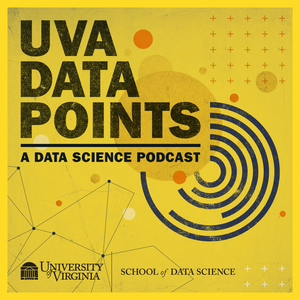
Exploring the Protein Universe via AI
UVA Data Points
04/23/25 • 30 min
Here we explore how data science is revolutionizing our understanding of protein structures, with a special focus on the exciting developments in protein folding and evolution. We’re joined by two experts in the field: Philip Bourne, the founding dean of the UVA School of Data Science, and Cam Mura, a biomolecular data scientist. From new tools like DeepUrfold to the future of biomedical applications, Bourne and Mura provide a unique look into how cutting-edge technology is transforming the world of molecular biology.
Show more best episodes

Show more best episodes
FAQ
How many episodes does UVA Data Points have?
UVA Data Points currently has 32 episodes available.
What topics does UVA Data Points cover?
The podcast is about Mathematics, Podcasts, Technology and Science.
What is the most popular episode on UVA Data Points?
The episode title 'The Future Impact of AI on Society Panel | Live from Datapalooza' is the most popular.
What is the average episode length on UVA Data Points?
The average episode length on UVA Data Points is 50 minutes.
How often are episodes of UVA Data Points released?
Episodes of UVA Data Points are typically released every 27 days, 23 hours.
When was the first episode of UVA Data Points?
The first episode of UVA Data Points was released on Aug 24, 2022.
Show more FAQ

Show more FAQ