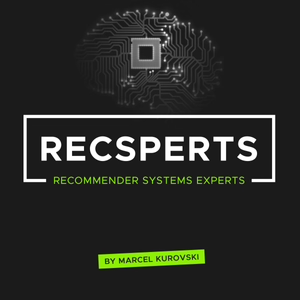
#13: The Netflix Recommender System and Beyond with Justin Basilico
Recsperts - Recommender Systems Experts02/15/23 • 80 min
This episode of Recsperts features Justin Basilico who is director of research and engineering at Netflix. Justin leads the team that is in charge of creating a personalized homepage. We learn more about the evolution of the Netflix recommender system from rating prediction to using deep learning, contextual multi-armed bandits and reinforcement learning to perform personalized page construction. Deep content understanding drives the creation of useful groupings of videos to be shown in a personalized homepage.
Justin and I discuss the misalignment of metrics as just one out of many elements that is making personalization still “super hard”. We hear more about the journey of deep learning for recommender systems where real usefulness comes from taking advantage of the variety of data besides pure user-item interactions, i.e. histories, content, and context. We also briefly touch on RecSysOps for detecting, predicting, diagnosing and resolving issues in a large-scale recommender systems and how it helps to alleviate item cold-start.
In the end of this episode, we talk about the company culture at Netflix. Key elements are freedom and responsibility as well as providing context instead of exerting control. We hear that being really comfortable with feedback is important for high-performance people and teams.
Enjoy this enriching episode of RECSPERTS - Recommender Systems Experts.
Chapters:
- (03:13) - Introduction Justin Basilico
- (07:37) - Evolution of the Netflix Recommender System
- (22:28) - Page Construction of the Personalized Netflix Homepage
- (32:12) - Misalignment of Metrics
- (37:36) - Experience with Deep Learning for Recommender Systens
- (48:10) - RecSysOps for Issue Detection, Diagnosis and Response
- (55:38) - Bandits Recommender Systems
- (01:03:22) - The Netflix Culture
- (01:13:33) - Further Challenges
- (01:15:48) - RecSys 2023 Industry Track
- (01:17:25) - Closing Remarks
Links from the Episode:
- Justin Basilico on Linkedin
- Justin Basilico on Twitter
- Netflix Research Publications
- The Netflix Tech Blog
- CONSEQUENCES+REVEAL Workshop at RecSys 2022
- Learning a Personalized Homepage (Alvino et al., 2015)
- Recent Trends in Personalization at Netflix (Basilico, 2021)
- RecSysOps: Best Practices for Operating a Large-Scale Recommender System (Saberian et al., 2022)
- Netflix Fourth Quarter 2022 Earnings Interview
- No Rules Rules - Netflix and the Culture of Reinvention (Hastings et al., 2020)
- Job Posting for Netflix' Recommendation Team
Papers:
- Steck et al. (2021): Deep Learning for Recommender Systems: A Netflix Case Study
- Steck et al. (2021): Negative Interactions for Improved Collaborative Filtering: Don't go Deeper, go Higher
- More et al. (2019): Recap: Designing a more Efficient Estimator for Off-policy Evaluation in Bandits with Large Action Spaces
- Bhattacharya et al. (2022): Augmenting Netflix Search with In-Session Adapted Recommendations
General Links:
- Follow me on Twitter: https://twitter.com/MarcelKurovski
- Send me your comments, questions and suggestions to [email protected]
- Podcast Website: https://www.recsperts.com/
02/15/23 • 80 min
Generate a badge
Get a badge for your website that links back to this episode
<a href="https://goodpods.com/podcasts/recsperts-recommender-systems-experts-537441/13-the-netflix-recommender-system-and-beyond-with-justin-basilico-69383368"> <img src="https://storage.googleapis.com/goodpods-images-bucket/badges/generic-badge-1.svg" alt="listen to #13: the netflix recommender system and beyond with justin basilico on goodpods" style="width: 225px" /> </a>
Copy