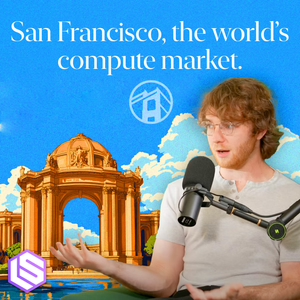
SF Compute: Commoditizing Compute
04/11/25 • 72 min
Evan Conrad, co-founder of SF Compute, joined us to talk about how they started as an AI lab that avoided bankruptcy by selling GPU clusters, why CoreWeave financials look like a real estate business, and how GPUs are turning into a commodities market.
Chapters:
00:00:05 - Introductions
00:00:12 - Introduction of guest Evan Conrad from SF Compute
00:00:12 - CoreWeave Business Model Discussion
00:05:37 - CoreWeave as a Real Estate Business
00:08:59 - Interest Rate Risk and GPU Market Strategy Framework
00:16:33 - Why Together and DigitalOcean will lose money on their clusters
00:20:37 - SF Compute's AI Lab Origins
00:25:49 - Utilization Rates and Benefits of SF Compute Market Model
00:30:00 - H100 GPU Glut, Supply Chain Issues, and Future Demand Forecast
00:34:00 - P2P GPU networks
00:36:50 - Customer stories
00:38:23 - VC-Provided GPU Clusters and Credit Risk Arbitrage
00:41:58 - Market Pricing Dynamics and Preemptible GPU Pricing Model
00:48:00 - Future Plans for Financialization?
00:52:59 - Cluster auditing and quality control
00:58:00 - Futures Contracts for GPUs
01:01:20 - Branding and Aesthetic Choices Behind SF Compute
01:06:30 - Lessons from Previous Startups
01:09:07 - Hiring at SF Compute
Chapters
- 00:00:00 Introduction and Background
- 00:00:58 Analysis of GPU Business Models
- 00:01:53 Challenges with GPU Pricing
- 00:02:48 Revenue and Scaling with GPUs
- 00:03:46 Customer Sensitivity to GPU Pricing
- 00:04:44 Core Weave's Business Strategy
- 00:05:41 Core Weave's Market Perception
- 00:06:40 Hyperscalers and GPU Market Dynamics
- 00:07:37 Financial Strategies for GPU Sales
- 00:08:35 Interest Rates and GPU Market Risks
- 00:09:30 Optimal GPU Contract Strategies
- 00:10:27 Risks in GPU Market Contracts
- 00:11:25 Price Sensitivity and Market Competition
- 00:12:21 Market Dynamics and GPU Contracts
- 00:13:18 Hyperscalers and GPU Market Strategies
- 00:14:15 Nvidia and Market Competition
- 00:15:12 Microsoft's Role in GPU Market
- 00:16:10 Challenges in GPU Market Dynamics
- 00:17:07 Economic Realities of the GPU Market
- 00:18:03 Real Estate Model for GPU Clouds
- 00:18:59 Price Sensitivity and Chip Design
- 00:19:55 SF Compute's Beginnings and Challenges
- 00:20:54 Navigating the GPU Market
- 00:21:54 Pivoting to a GPU Cloud Provider
- 00:22:53 Building a GPU Market
- 00:23:52 SF Compute as a GPU Marketplace
- 00:24:49 Market Liquidity and GPU Pricing
- 00:25:47 Utilization Rates in GPU Markets
- 00:26:44 Brokerage and Market Flexibility
- 00:27:42 H100 Glut and Market Cycles
- 00:28:40 Supply Chain Challenges and GPU Glut
- 00:29:35 Future Predictions for the GPU Market
- 00:30:33 Speculations on Test Time Inference
- 00:31:29 Market Demand and Test Time Inference
- 00:32:26 Open Source vs. Closed AI Demand
- 00:33:24 Future of Inference Demand
- 00:34:24 Peer-to-Peer GPU Markets
- 00:35:17 Decentralized GPU Market Skepticism
- 00:36:15 Redesigning Architectures for New Markets
- 00:37:14 Supporting Grad Students and Startups
- 00:38:11 Successful Startups Using SF Compute
- 00:39:11 VCs and GPU Infrastructure
- 00:40:09 VCs as GPU Credit Transformators
- 00:41:06 Market Timing and GPU Infrastructure
- 00:42:02 Understanding GPU Pricing Dynamics
- 00:43:01 Market Pricing and Preemptible Compute
- 00:43:55 Price Volatility and Market Optimization
- 00:44:52 Customizing Compute Contracts
- 00:45:50 Creating Flexible Compute Guarantees
- 00:46:45 Financialization of GPU Markets
- 00:47:44 Building a Spot Market for GPUs
- 00:48:40 Auditing and Standardizing Clusters
- 00:49:40 Ensuring Cluster Reliability
- 00:50:36 Active Mo...
Evan Conrad, co-founder of SF Compute, joined us to talk about how they started as an AI lab that avoided bankruptcy by selling GPU clusters, why CoreWeave financials look like a real estate business, and how GPUs are turning into a commodities market.
Chapters:
00:00:05 - Introductions
00:00:12 - Introduction of guest Evan Conrad from SF Compute
00:00:12 - CoreWeave Business Model Discussion
00:05:37 - CoreWeave as a Real Estate Business
00:08:59 - Interest Rate Risk and GPU Market Strategy Framework
00:16:33 - Why Together and DigitalOcean will lose money on their clusters
00:20:37 - SF Compute's AI Lab Origins
00:25:49 - Utilization Rates and Benefits of SF Compute Market Model
00:30:00 - H100 GPU Glut, Supply Chain Issues, and Future Demand Forecast
00:34:00 - P2P GPU networks
00:36:50 - Customer stories
00:38:23 - VC-Provided GPU Clusters and Credit Risk Arbitrage
00:41:58 - Market Pricing Dynamics and Preemptible GPU Pricing Model
00:48:00 - Future Plans for Financialization?
00:52:59 - Cluster auditing and quality control
00:58:00 - Futures Contracts for GPUs
01:01:20 - Branding and Aesthetic Choices Behind SF Compute
01:06:30 - Lessons from Previous Startups
01:09:07 - Hiring at SF Compute
Chapters
- 00:00:00 Introduction and Background
- 00:00:58 Analysis of GPU Business Models
- 00:01:53 Challenges with GPU Pricing
- 00:02:48 Revenue and Scaling with GPUs
- 00:03:46 Customer Sensitivity to GPU Pricing
- 00:04:44 Core Weave's Business Strategy
- 00:05:41 Core Weave's Market Perception
- 00:06:40 Hyperscalers and GPU Market Dynamics
- 00:07:37 Financial Strategies for GPU Sales
- 00:08:35 Interest Rates and GPU Market Risks
- 00:09:30 Optimal GPU Contract Strategies
- 00:10:27 Risks in GPU Market Contracts
- 00:11:25 Price Sensitivity and Market Competition
- 00:12:21 Market Dynamics and GPU Contracts
- 00:13:18 Hyperscalers and GPU Market Strategies
- 00:14:15 Nvidia and Market Competition
- 00:15:12 Microsoft's Role in GPU Market
- 00:16:10 Challenges in GPU Market Dynamics
- 00:17:07 Economic Realities of the GPU Market
- 00:18:03 Real Estate Model for GPU Clouds
- 00:18:59 Price Sensitivity and Chip Design
- 00:19:55 SF Compute's Beginnings and Challenges
- 00:20:54 Navigating the GPU Market
- 00:21:54 Pivoting to a GPU Cloud Provider
- 00:22:53 Building a GPU Market
- 00:23:52 SF Compute as a GPU Marketplace
- 00:24:49 Market Liquidity and GPU Pricing
- 00:25:47 Utilization Rates in GPU Markets
- 00:26:44 Brokerage and Market Flexibility
- 00:27:42 H100 Glut and Market Cycles
- 00:28:40 Supply Chain Challenges and GPU Glut
- 00:29:35 Future Predictions for the GPU Market
- 00:30:33 Speculations on Test Time Inference
- 00:31:29 Market Demand and Test Time Inference
- 00:32:26 Open Source vs. Closed AI Demand
- 00:33:24 Future of Inference Demand
- 00:34:24 Peer-to-Peer GPU Markets
- 00:35:17 Decentralized GPU Market Skepticism
- 00:36:15 Redesigning Architectures for New Markets
- 00:37:14 Supporting Grad Students and Startups
- 00:38:11 Successful Startups Using SF Compute
- 00:39:11 VCs and GPU Infrastructure
- 00:40:09 VCs as GPU Credit Transformators
- 00:41:06 Market Timing and GPU Infrastructure
- 00:42:02 Understanding GPU Pricing Dynamics
- 00:43:01 Market Pricing and Preemptible Compute
- 00:43:55 Price Volatility and Market Optimization
- 00:44:52 Customizing Compute Contracts
- 00:45:50 Creating Flexible Compute Guarantees
- 00:46:45 Financialization of GPU Markets
- 00:47:44 Building a Spot Market for GPUs
- 00:48:40 Auditing and Standardizing Clusters
- 00:49:40 Ensuring Cluster Reliability
- 00:50:36 Active Mo...
Previous Episode
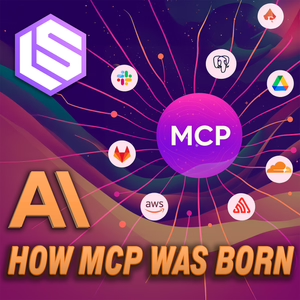
The Creators of Model Context Protocol
Today’s guests, David Soria Parra and Justin Spahr-Summers, are the creators of Anthropic’s Model Context Protocol (MCP). When we first wrote Why MCP Won, we had no idea how quickly it was about to win.
In the past 4 weeks, OpenAI and now Google have now announced the MCP support, effectively confirming our prediction that MCP was the presumptive winner of the agent standard wars. MCP has now overtaken OpenAPI, the incumbent option and most direct alternative, in GitHub stars (3 months ahead of conservative trendline):
For protocol and history nerds, we also asked David and Justin to tell the origin story of MCP, which we leave to the reader to enjoy (you can also skim the transcripts, or, the changelogs of a certain favored IDE). It’s incredible the impact that individual engineers solving their own problems can have on an entire industry.
Timestamps
00:00 Introduction and Guest Welcome
00:37 What is MCP?
02:00 The Origin Story of MCP
05:18 Development Challenges and Solutions
08:06 Technical Details and Inspirations
29:45 MCP vs Open API
32:48 Building MCP Servers
40:39 Exploring Model Independence in LLMs
41:36 Building Richer Systems with MCP
43:13 Understanding Agents in MCP
45:45 Nesting and Tool Confusion in MCP
49:11 Client Control and Tool Invocation
52:08 Authorization and Trust in MCP Servers
01:01:34 Future Roadmap and Stateless Servers
01:10:07 Open Source Governance and Community Involvement
01:18:12 Wishlist and Closing Remarks
Next Episode
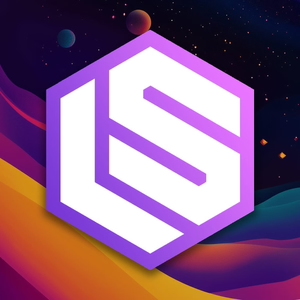
⚡️GPT 4.1: The New OpenAI Workhorse
We’ll keep this brief because we’re on a tight turnaround: GPT 4.1, previously known as the Quasar and Optimus models, is now live as the natural update for 4o/4o-mini (and the research preview of GPT 4.5). Though it is a general purpose model family, the headline features are:
Coding abilities (o1-level SWEBench and SWELancer, but ok Aider)
Instruction Following (with a very notable prompting guide)
Long Context up to 1m tokens (with new MRCR and Graphwalk benchmarks)
Vision (simply o1 level)
Cheaper Pricing (cheaper than 4o, greatly improved prompt caching savings)
We caught up with returning guest Michelle Pokrass and Josh McGrath to get more detail on each!
Chapters
- 00:00:00 Introduction and Guest Welcome
- 00:00:57 GPC 4.1 Launch Overview
- 00:01:54 Developer Feedback and Model Names
- 00:02:53 Model Naming and Starry Themes
- 00:03:49 Confusion Over GPC 4.1 vs 4.5
- 00:04:47 Distillation and Model Improvements
- 00:05:45 Omnimodel Architecture and Future Plans
- 00:06:43 Core Capabilities of GPC 4.1
- 00:07:40 Training Techniques and Long Context
- 00:08:37 Challenges in Long Context Reasoning
- 00:09:34 Context Utilization in Models
- 00:10:31 Graph Walks and Model Evaluation
- 00:11:31 Real Life Applications of Graph Tasks
- 00:12:30 Multi-Hop Reasoning Benchmarks
- 00:13:30 Agentic Workflows and Backtracking
- 00:14:28 Graph Traversals for Agent Planning
- 00:15:24 Context Usage in API and Memory Systems
- 00:16:21 Model Performance in Long Context Tasks
- 00:17:17 Instruction Following and Real World Data
- 00:18:12 Challenges in Grading Instructions
- 00:19:09 Instruction Following Techniques
- 00:20:09 Prompting Techniques and Model Responses
- 00:21:05 Agentic Workflows and Model Persistence
- 00:22:01 Balancing Persistence and User Control
- 00:22:56 Evaluations on Model Edits and Persistence
- 00:23:55 XML vs JSON in Prompting
- 00:24:50 Instruction Placement in Context
- 00:25:49 Optimizing for Prompt Caching
- 00:26:49 Chain of Thought and Reasoning Models
- 00:27:46 Choosing the Right Model for Your Task
- 00:28:46 Coding Capabilities of GPC 4.1
- 00:29:41 Model Performance in Coding Tasks
- 00:30:39 Understanding Coding Model Differences
- 00:31:36 Using Smaller Models for Coding
- 00:32:33 Future of Coding in OpenAI
- 00:33:28 Internal Use and Success Stories
- 00:34:26 Vision and Multi-Modal Capabilities
- 00:35:25 Screen vs Embodied Vision
- 00:36:22 Vision Benchmarks and Model Improvements
- 00:37:19 Model Deprecation and GPU Usage
- 00:38:13 Fine-Tuning and Preference Steering
- 00:39:12 Upcoming Reasoning Models
- 00:40:10 Creative Writing and Model Humor
- 00:41:07 Feedback and Developer Community
- 00:42:03 Pricing and Blended Model Costs
- 00:44:02 Conclusion and Wrap-Up
If you like this episode you’ll love
Episode Comments
Generate a badge
Get a badge for your website that links back to this episode
<a href="https://goodpods.com/podcasts/latent-space-the-ai-engineer-podcast-262472/sf-compute-commoditizing-compute-89402193"> <img src="https://storage.googleapis.com/goodpods-images-bucket/badges/generic-badge-1.svg" alt="listen to sf compute: commoditizing compute on goodpods" style="width: 225px" /> </a>
Copy