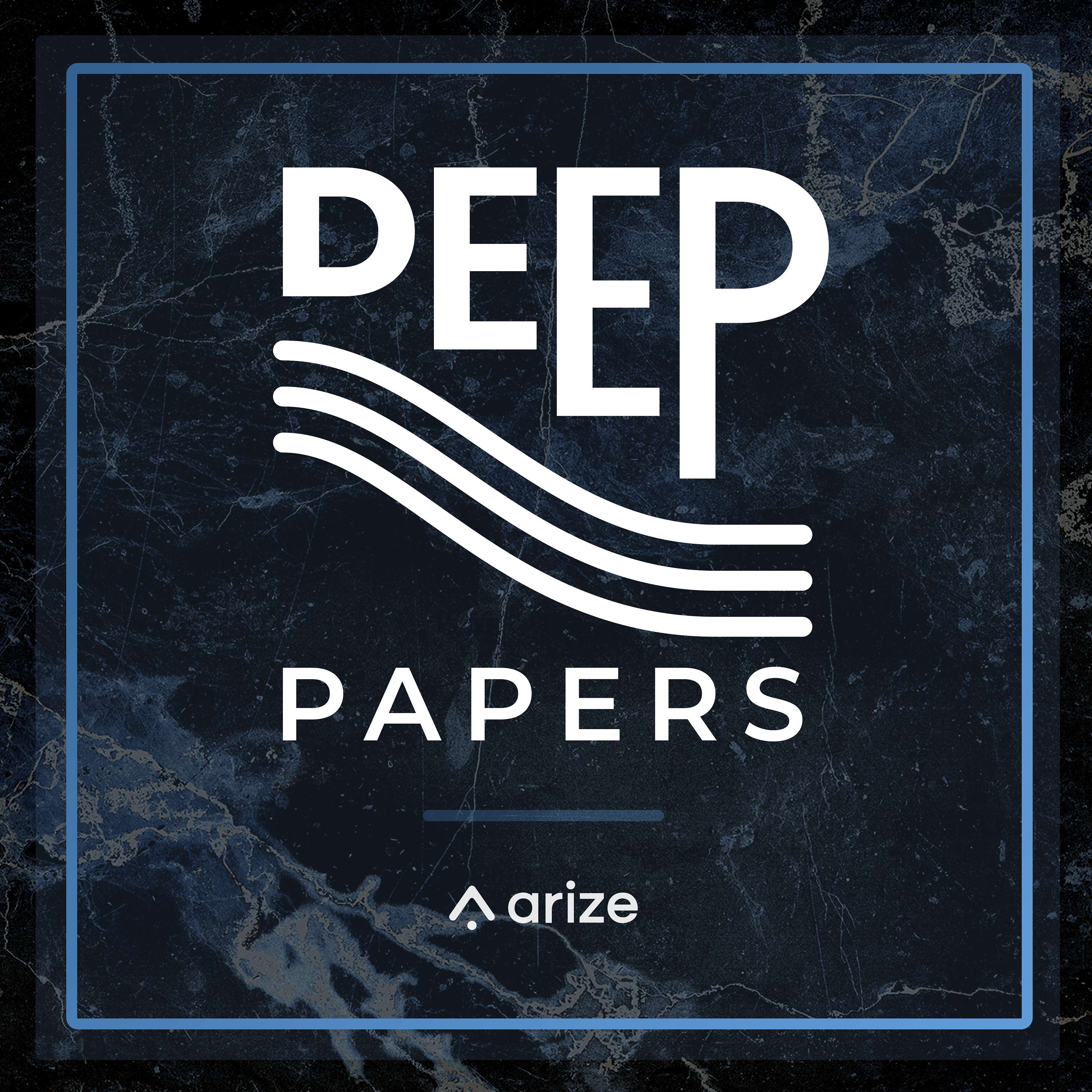
Deep Papers is a podcast series featuring deep dives on today’s seminal AI papers and research. Hosted by AI Pub creator Brian Burns and Arize AI founders Jason Lopatecki and Aparna Dhinakaran, each episode profiles the people and techniques behind cutting-edge breakthroughs in machine learning.
In this episode, we talk about Orca. Recent research focuses on improving smaller models through imitation learning using outputs from large foundation models (LFMs). Challenges include limited imitation signals, homogeneous training data, and a lack of rigorous evaluation, leading to overestimation of small model capabilities.
To address this, Orca is a 13-billion parameter model that learns to imitate LFMs’ reasoning process. Orca leverages rich signals from GPT-4, surpassing state-of-the-art models by over 100% in complex zero-shot reasoning benchmarks. It also shows competitive performance in professional and academic exams without CoT. Learning from step-by-step explanations, generated by humans or advanced AI models, enhances model capabilities and skills.
Full transcript and more here: https://arize.com/blog/orca-progressive-learning-from-complex-explanation-traces-of-gpt-4-paper-reading/
Learn more about AI observability and evaluation in our course, join the Arize AI Slack community or get the latest on LinkedIn and X.
07/21/23 • 42 min
1 Listener
Generate a badge
Get a badge for your website that links back to this episode
<a href="https://goodpods.com/podcasts/deep-papers-251735/orca-progressive-learning-from-complex-explanation-traces-of-gpt-4-31797893"> <img src="https://storage.googleapis.com/goodpods-images-bucket/badges/generic-badge-1.svg" alt="listen to orca: progressive learning from complex explanation traces of gpt-4 on goodpods" style="width: 225px" /> </a>
Copy