![DataCafé - [Bite] Documenting Data Science Projects](https://storage.googleapis.com/goodpods-images-bucket/episode_images/739ed39b986964a82e145773e96fef06c9d22c667d080658425600d0e911113c.avif)
[Bite] Documenting Data Science Projects
06/29/22 • 16 min
Do you ever find yourself wondering what the data was you used in a project? When was it obtained and where is it stored? Or even just the way to run a piece of code that produced a previous output and needs to be revisited?
Chances are the answer is yes. And it’s likely you have been frustrated by not knowing how to reproduce an output or rerun a codebase or even who to talk to to obtain a refresh of the data - in some way, shape, or form.
The problem that a lot of project teams face, and data scientists in particular, is the agreement and effort to document their work in a robust and reliable fashion. Documentation is a broad term and can refer to all manner of project details, from the actions captured in a team meeting to the technical guides for executing an algorithm.
In this bite episode of DataCafé we discuss the challenges around documentation in data science projects (though it applies more broadly). We motivate the need for good documentation through agreement of the responsibilities, expectations, and methods of capturing notes and guides. This can be everything from a summary of the data sources and how to preprocess input data, to project plans and meeting minutes, through to technical details on the dependencies and setups for running codes.
Thanks for joining us in the DataCafé. You can follow us on twitter @DataCafePodcast and feel free to contact us about anything you've heard here or think would be an interesting topic in the future.
Do you ever find yourself wondering what the data was you used in a project? When was it obtained and where is it stored? Or even just the way to run a piece of code that produced a previous output and needs to be revisited?
Chances are the answer is yes. And it’s likely you have been frustrated by not knowing how to reproduce an output or rerun a codebase or even who to talk to to obtain a refresh of the data - in some way, shape, or form.
The problem that a lot of project teams face, and data scientists in particular, is the agreement and effort to document their work in a robust and reliable fashion. Documentation is a broad term and can refer to all manner of project details, from the actions captured in a team meeting to the technical guides for executing an algorithm.
In this bite episode of DataCafé we discuss the challenges around documentation in data science projects (though it applies more broadly). We motivate the need for good documentation through agreement of the responsibilities, expectations, and methods of capturing notes and guides. This can be everything from a summary of the data sources and how to preprocess input data, to project plans and meeting minutes, through to technical details on the dependencies and setups for running codes.
Thanks for joining us in the DataCafé. You can follow us on twitter @DataCafePodcast and feel free to contact us about anything you've heard here or think would be an interesting topic in the future.
Previous Episode
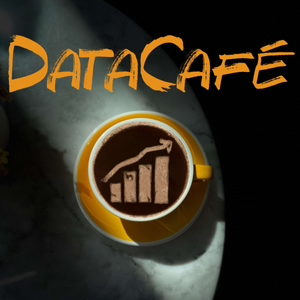
Landing Data Science Projects: The Art of Change Management & Implementation
Are people resistant to change? And if so, how do you manage that when trying to introduce and deliver innovation through Data Science?
In this episode of the DataCafé we discuss the challenges faced when trying to land a data science project. There are a number of potential barriers to success that need to be carefully managed. We talk about "change management" and aspects of employee behaviours and stakeholder management that influence the chances of landing a project. This is especially important for embedding innovation in your company or organisation, and implementing a plan to sustain the changes needed to deliver long-term value.
Further reading & references
- Kotter's 8 Step Change Plan
- Armenakis, Achilles & Harris, Stanley & Mossholder, Kevin. (1993). Creating Readiness for Organizational Change. Human Relations. 46. 681-704. 10.1177/001872679304600601.
- Lewin, K (1944a) Constructs in Field Theory. In D Cartwright(Ed):(1952) Field Theory in Social Science: Selected Theoretical Papers by Kurt Lewin. London: Social Science Paperbacks. pp30-42
- Lewin, K. (1947) ‘Frontiers in Group Dynamics: Concept, Method and Reality in Social Science; Social Equilibria and Social Change’, Human Relations, 1(1), pp. 5–41. doi: 10.1177/001872674700100103.
Some links above may require payment or login. We are not endorsing them or receiving any payment for mentioning them. They are provided as is. Often free versions of papers are available and we would encourage you to investigate.
Recording date: 10 February 2022
Thanks for joining us in the DataCafé. You can follow us on twitter @DataCafePodcast and feel free to contact us about anything you've heard here or think would be an interesting topic in the future.
Next Episode
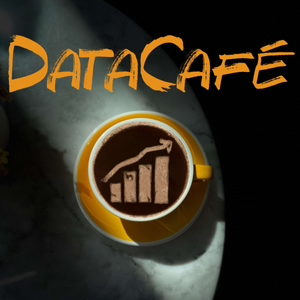
Scaling the Internet
Do you have multiple devices connected to your internet fighting for your bandwidth? Are you asking your children (or even neighbours!) to get off the network so you can finish an important call? Recent lockdowns caused huge network contention as everyone moved to online meetings and virtual classrooms. This is an optimisation challenge that requires advanced modelling and simulation to tackle. How can a network provider know how much bandwidth to provision to a town or a city to cope with peak demands? That's where agent-based simulations come in - to allow network designers to anticipate and then plan for high-demand events, applications and trends.
In this episode of the DataCafé we hear from Dr. Lucy Gullon, AI and Optimisation Research Specialist at Applied Research, BT. She tells us about the efforts underway to assess the need for bandwidth across different households and locations, and the work they lead to model, simulate, and optimise the provision of that bandwidth across the network of the UK. We hear how planning for peak use, where, say, the nation is streaming a football match is an important consideration. At the same time, reacting to times of low throughput can help to switch off unused circuits and equipment and save a lot of energy.
Interview Guest: Dr. Lucy Gullon, AI and Optimisation Research Specialist from Applied Research, BT.
Further reading:
- BT Research and Development (https://www.bt.com/about/bt/research-and-development)
- Anylogic agent-based simulator (https://www.anylogic.com/use-of-simulation/agent-based-modeling/)
- Article: Agent-based modelling (via Wikipedia)
- Article:Prisoner's Dilemma (via Wikipedia)
- Article: Crowd Simulation (via Wikipedia)
- Book: Science and the City (via Bloomsbury)
- Research group: Traffic Modelling (via mit.edu)
Some links above may require payment or login. We are not endorsing them or receiving any payment for mentioning them. They are provided as is. Often free versions of papers are available and we would encourage you to investigate.
Recording date: 5 May 2022
Interview date: 27 Apr 2022
Thanks for joining us in the DataCafé. You can follow us on twitter @DataCafePodcast and feel free to contact us about anything you've heard here or think would be an interesting topic in the future.
If you like this episode you’ll love
Episode Comments
Generate a badge
Get a badge for your website that links back to this episode
<a href="https://goodpods.com/podcasts/datacaf%c3%a9-241830/bite-documenting-data-science-projects-26902026"> <img src="https://storage.googleapis.com/goodpods-images-bucket/badges/generic-badge-1.svg" alt="listen to [bite] documenting data science projects on goodpods" style="width: 225px" /> </a>
Copy