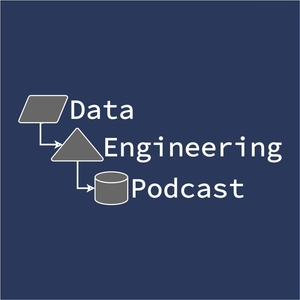
Operational Analytics At Speed With Minimal Busy Work Using Incorta
Data Engineering Podcast04/24/22 • 71 min
Summary
A huge amount of effort goes into modeling and shaping data to make it available for analytical purposes. This is often due to the need to simplify the final queries so that they are performant for visualization or limited exploration. In order to cut down the level of effort involved in making data usable, Matthew Halliday and his co-founders created Incorta as an end-to-end, in-memory analytical engine that removes barriers to insights on your data. In this episode he explains how the system works, the use cases that it empowers, and how you can start using it for your own analytics today.
Announcements
- Hello and welcome to the Data Engineering Podcast, the show about modern data management
- When you’re ready to build your next pipeline, or want to test out the projects you hear about on the show, you’ll need somewhere to deploy it, so check out our friends at Linode. With their managed Kubernetes platform it’s now even easier to deploy and scale your workflows, or try out the latest Helm charts from tools like Pulsar and Pachyderm. With simple pricing, fast networking, object storage, and worldwide data centers, you’ve got everything you need to run a bulletproof data platform. Go to dataengineeringpodcast.com/linode today and get a $100 credit to try out a Kubernetes cluster of your own. And don’t forget to thank them for their continued support of this show!
- Atlan is a collaborative workspace for data-driven teams, like Github for engineering or Figma for design teams. By acting as a virtual hub for data assets ranging from tables and dashboards to SQL snippets & code, Atlan enables teams to create a single source of truth for all their data assets, and collaborate across the modern data stack through deep integrations with tools like Snowflake, Slack, Looker and more. Go to dataengineeringpodcast.com/atlan today and sign up for a free trial. If you’re a data engineering podcast listener, you get credits worth $3000 on an annual subscription
- Modern data teams are dealing with a lot of complexity in their data pipelines and analytical code. Monitoring data quality, tracing incidents, and testing changes can be daunting and often takes hours to days or even weeks. By the time errors have made their way into production, it’s often too late and damage is done. Datafold built automated regression testing to help data and analytics engineers deal with data quality in their pull requests. Datafold shows how a change in SQL code affects your data, both on a statistical level and down to individual rows and values before it gets merged to production. No more shipping and praying, you can now know exactly what will change in your database! Datafold integrates with all major data warehouses as well as frameworks such as Airflow & dbt and seamlessly plugs into CI workflows. Visit dataengineeringpodcast.com/datafold today to book a demo with Datafold.
- Struggling with broken pipelines? Stale dashboards? Missing data? If this resonates with you, you’re not alone. Data engineers struggling with unreliable data need look no further than Monte Carlo, the leading end-to-end Data Observability Platform! Trusted by the data teams at Fox, JetBlue, and PagerDuty, Monte Carlo solves the costly problem of broken data pipelines. Monte Carlo monitors and alerts for data issues across your data warehouses, data lakes, dbt models, Airflow jobs, and business intelligence tools, reducing time to detection and resolution from weeks to just minutes. Monte Carlo also gives you a holistic picture of data health with automatic, end-to-end lineage from ingestion to the BI layer directly out of the box. Start trusting your data with Monte Carlo today! Visit http://www.dataengineeringpodcast.com/montecarlo?utm_source=rss&utm_medium=rss to learn more.
- Your host is Tobias Macey and today I’m interviewing Matthew Halliday about Incorta, an in-memory, unified data and analytics platform as a service
Interview
- Introduction
- How did you get involved in the area of data management?
- Can you describe what Incorta is and the story behind it?
- What are the use cases and customers that you are focused on?
- How does that focus inform the design and priorities of functionality in the product?
- What are the technologies and workflows that Incorta might replace?
- What are the systems and services that it is intended to integrate with and extend?
- Can you describe how Incorta is implemented?
- What are the core technological decisions that were necessary to make t...
04/24/22 • 71 min
1 Listener
Data Engineering Podcast - Operational Analytics At Speed With Minimal Busy Work Using Incorta
Transcript
Hello, and welcome to the Data Engineering Podcast, the show about modern data management.
Have you ever woken up to a crisis because a number on a dashboard is broken and no 1 knows why? Or sent out frustrating Slack messages trying to find the right dataset? Or tried to understand what a column name means?
Our friends at Outland started out as a data team themselves and faced all this collaboratio
Generate a badge
Get a badge for your website that links back to this episode
<a href="https://goodpods.com/podcasts/data-engineering-podcast-203077/operational-analytics-at-speed-with-minimal-busy-work-using-incorta-20706335"> <img src="https://storage.googleapis.com/goodpods-images-bucket/badges/generic-badge-1.svg" alt="listen to operational analytics at speed with minimal busy work using incorta on goodpods" style="width: 225px" /> </a>
Copy