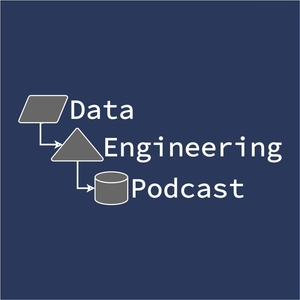
DataOps As A Service For Your Data Integration Workflows With Rivery
04/11/22 • 58 min
Summary
Data engineering is a practice that is multi-faceted and requires integration with a large number of systems. This often means working across multiple tools to get the job done which can introduce significant cost to productivity due to the number of context switches. Rivery is a platform designed to reduce this incidental complexity and provide a single system for working across the different stages of the data lifecycle. In this episode CEO and founder Itamar Ben hemo explains how his experiences in the industry led to his vision for the Rivery platform as a single place to build end-to-end analytical workflows, including how it is architected and how you can start using it today for your own work.
Announcements
- Hello and welcome to the Data Engineering Podcast, the show about modern data management
- When you’re ready to build your next pipeline, or want to test out the projects you hear about on the show, you’ll need somewhere to deploy it, so check out our friends at Linode. With their managed Kubernetes platform it’s now even easier to deploy and scale your workflows, or try out the latest Helm charts from tools like Pulsar and Pachyderm. With simple pricing, fast networking, object storage, and worldwide data centers, you’ve got everything you need to run a bulletproof data platform. Go to dataengineeringpodcast.com/linode today and get a $100 credit to try out a Kubernetes cluster of your own. And don’t forget to thank them for their continued support of this show!
- Atlan is a collaborative workspace for data-driven teams, like Github for engineering or Figma for design teams. By acting as a virtual hub for data assets ranging from tables and dashboards to SQL snippets & code, Atlan enables teams to create a single source of truth for all their data assets, and collaborate across the modern data stack through deep integrations with tools like Snowflake, Slack, Looker and more. Go to dataengineeringpodcast.com/atlan today and sign up for a free trial. If you’re a data engineering podcast listener, you get credits worth $3000 on an annual subscription
- Modern data teams are dealing with a lot of complexity in their data pipelines and analytical code. Monitoring data quality, tracing incidents, and testing changes can be daunting and often takes hours to days or even weeks. By the time errors have made their way into production, it’s often too late and damage is done. Datafold built automated regression testing to help data and analytics engineers deal with data quality in their pull requests. Datafold shows how a change in SQL code affects your data, both on a statistical level and down to individual rows and values before it gets merged to production. No more shipping and praying, you can now know exactly what will change in your database! Datafold integrates with all major data warehouses as well as frameworks such as Airflow & dbt and seamlessly plugs into CI workflows. Visit dataengineeringpodcast.com/datafold today to book a demo with Datafold.
- Are you looking for a structured and battle-tested approach for learning data engineering? Would you like to know how you can build proper data infrastructures that are built to last? Would you like to have a seasoned industry expert guide you and answer all your questions? Join Pipeline Academy, the worlds first data engineering bootcamp. Learn in small groups with likeminded professionals for 9 weeks part-time to level up in your career. The course covers the most relevant and essential data and software engineering topics that enable you to start your journey as a professional data engineer or analytics engineer. Plus we have AMAs with world-class guest speakers every week! The next cohort starts in April 2022. Visit dataengineeringpodcast.com/academy and apply now!
- Your host is Tobias Macey and today I’m interviewing Itamar Ben Hemo about Rivery, a SaaS platform designed to provide an end-to-end solution for Ingestion, Transformation, Orchestration, and Data Operations
Interview
- Introduction
- How did you get involved in the area of data management?
- Can you describe what Rivery is and the story behind it?
- What are the primary goals of Rivery as a platform and company?
- What are the target personas for the Rivery platform?
- What are the points of interaction/workflows for each of those personas?
- What are some of the positive and negative sources of inspiration that you looked to while deciding on the scope of t...
Summary
Data engineering is a practice that is multi-faceted and requires integration with a large number of systems. This often means working across multiple tools to get the job done which can introduce significant cost to productivity due to the number of context switches. Rivery is a platform designed to reduce this incidental complexity and provide a single system for working across the different stages of the data lifecycle. In this episode CEO and founder Itamar Ben hemo explains how his experiences in the industry led to his vision for the Rivery platform as a single place to build end-to-end analytical workflows, including how it is architected and how you can start using it today for your own work.
Announcements
- Hello and welcome to the Data Engineering Podcast, the show about modern data management
- When you’re ready to build your next pipeline, or want to test out the projects you hear about on the show, you’ll need somewhere to deploy it, so check out our friends at Linode. With their managed Kubernetes platform it’s now even easier to deploy and scale your workflows, or try out the latest Helm charts from tools like Pulsar and Pachyderm. With simple pricing, fast networking, object storage, and worldwide data centers, you’ve got everything you need to run a bulletproof data platform. Go to dataengineeringpodcast.com/linode today and get a $100 credit to try out a Kubernetes cluster of your own. And don’t forget to thank them for their continued support of this show!
- Atlan is a collaborative workspace for data-driven teams, like Github for engineering or Figma for design teams. By acting as a virtual hub for data assets ranging from tables and dashboards to SQL snippets & code, Atlan enables teams to create a single source of truth for all their data assets, and collaborate across the modern data stack through deep integrations with tools like Snowflake, Slack, Looker and more. Go to dataengineeringpodcast.com/atlan today and sign up for a free trial. If you’re a data engineering podcast listener, you get credits worth $3000 on an annual subscription
- Modern data teams are dealing with a lot of complexity in their data pipelines and analytical code. Monitoring data quality, tracing incidents, and testing changes can be daunting and often takes hours to days or even weeks. By the time errors have made their way into production, it’s often too late and damage is done. Datafold built automated regression testing to help data and analytics engineers deal with data quality in their pull requests. Datafold shows how a change in SQL code affects your data, both on a statistical level and down to individual rows and values before it gets merged to production. No more shipping and praying, you can now know exactly what will change in your database! Datafold integrates with all major data warehouses as well as frameworks such as Airflow & dbt and seamlessly plugs into CI workflows. Visit dataengineeringpodcast.com/datafold today to book a demo with Datafold.
- Are you looking for a structured and battle-tested approach for learning data engineering? Would you like to know how you can build proper data infrastructures that are built to last? Would you like to have a seasoned industry expert guide you and answer all your questions? Join Pipeline Academy, the worlds first data engineering bootcamp. Learn in small groups with likeminded professionals for 9 weeks part-time to level up in your career. The course covers the most relevant and essential data and software engineering topics that enable you to start your journey as a professional data engineer or analytics engineer. Plus we have AMAs with world-class guest speakers every week! The next cohort starts in April 2022. Visit dataengineeringpodcast.com/academy and apply now!
- Your host is Tobias Macey and today I’m interviewing Itamar Ben Hemo about Rivery, a SaaS platform designed to provide an end-to-end solution for Ingestion, Transformation, Orchestration, and Data Operations
Interview
- Introduction
- How did you get involved in the area of data management?
- Can you describe what Rivery is and the story behind it?
- What are the primary goals of Rivery as a platform and company?
- What are the target personas for the Rivery platform?
- What are the points of interaction/workflows for each of those personas?
- What are some of the positive and negative sources of inspiration that you looked to while deciding on the scope of t...
Previous Episode
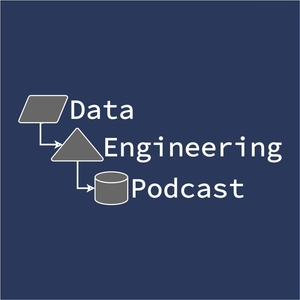
Synthetic Data As A Service For Simplifying Privacy Engineering With Gretel
Summary
Any time that you are storing data about people there are a number of privacy and security considerations that come with it. Privacy engineering is a growing field in data management that focuses on how to protect attributes of personal data so that the containing datasets can be shared safely. In this episode Gretel co-founder and CTO John Myers explains how they are building tools for data engineers and analysts to incorporate privacy engineering techniques into their workflows and validate the safety of their data against re-identification attacks.
Announcements
- Hello and welcome to the Data Engineering Podcast, the show about modern data management
- When you’re ready to build your next pipeline, or want to test out the projects you hear about on the show, you’ll need somewhere to deploy it, so check out our friends at Linode. With their managed Kubernetes platform it’s now even easier to deploy and scale your workflows, or try out the latest Helm charts from tools like Pulsar and Pachyderm. With simple pricing, fast networking, object storage, and worldwide data centers, you’ve got everything you need to run a bulletproof data platform. Go to dataengineeringpodcast.com/linode today and get a $100 credit to try out a Kubernetes cluster of your own. And don’t forget to thank them for their continued support of this show!
- This episode is brought to you by Acryl Data, the company behind DataHub, the leading developer-friendly data catalog for the modern data stack. Open Source DataHub is running in production at several companies like Peloton, Optum, Udemy, Zynga and others. Acryl Data provides DataHub as an easy to consume SaaS product which has been adopted by several companies. Signup for the SaaS product at dataengineeringpodcast.com/acryl
- Are you looking for a structured and battle-tested approach for learning data engineering? Would you like to know how you can build proper data infrastructures that are built to last? Would you like to have a seasoned industry expert guide you and answer all your questions? Join Pipeline Academy, the worlds first data engineering bootcamp. Learn in small groups with likeminded professionals for 9 weeks part-time to level up in your career. The course covers the most relevant and essential data and software engineering topics that enable you to start your journey as a professional data engineer or analytics engineer. Plus we have AMAs with world-class guest speakers every week! The next cohort starts in April 2022. Visit dataengineeringpodcast.com/academy and apply now!
- RudderStack helps you build a customer data platform on your warehouse or data lake. Instead of trapping data in a black box, they enable you to easily collect customer data from the entire stack and build an identity graph on your warehouse, giving you full visibility and control. Their SDKs make event streaming from any app or website easy, and their state-of-the-art reverse ETL pipelines enable you to send enriched data to any cloud tool. Sign up free... or just get the free t-shirt for being a listener of the Data Engineering Podcast at dataengineeringpodcast.com/rudder.
- Your host is Tobias Macey and today I’m interviewing John Myers about privacy engineering and use cases for synthetic data
Interview
- Introduction
- How did you get involved in the area of data management?
- Can you describe what Gretel is and the story behind it?
- How do you define "privacy engineering"?
- In an organization or data team, who is typically responsible for privacy engineering?
- How would you characterize the current state of the art and adoption for privacy engineering?
- Who are the target users of Gretel and how does that inform the features and design of the product?
- What are the stages of the data lifecycle where Gretel is used?
- Can you describe a typical workflow for integrating Gretel into data pipelines for business analytics or ML model training?
- How is the Gretel platform implemented?
- How have the design and goals of the system changed or evolved since you started working on it?
- What are some of the nuances of synthetic data generation or masking that data engineers/data analysts need to be aware of as they start using Gretel?
- What are the most interesting, innovative, or unexpected ways that you have seen Gretel used?
- What are the most interesting, unexpected, or challenging lessons that you h...
Next Episode
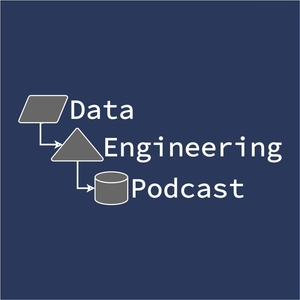
What Does It Really Mean To Do MLOps And What Is The Data Engineer's Role?
Summary
Putting machine learning models into production and keeping them there requires investing in well-managed systems to manage the full lifecycle of data cleaning, training, deployment and monitoring. This requires a repeatable and evolvable set of processes to keep it functional. The term MLOps has been coined to encapsulate all of these principles and the broader data community is working to establish a set of best practices and useful guidelines for streamlining adoption. In this episode Demetrios Brinkmann and David Aponte share their perspectives on this rapidly changing space and what they have learned from their work building the MLOps community through blog posts, podcasts, and discussion forums.
Announcements
- Hello and welcome to the Data Engineering Podcast, the show about modern data management
- When you’re ready to build your next pipeline, or want to test out the projects you hear about on the show, you’ll need somewhere to deploy it, so check out our friends at Linode. With their managed Kubernetes platform it’s now even easier to deploy and scale your workflows, or try out the latest Helm charts from tools like Pulsar and Pachyderm. With simple pricing, fast networking, object storage, and worldwide data centers, you’ve got everything you need to run a bulletproof data platform. Go to dataengineeringpodcast.com/linode today and get a $100 credit to try out a Kubernetes cluster of your own. And don’t forget to thank them for their continued support of this show!
- This episode is brought to you by Acryl Data, the company behind DataHub, the leading developer-friendly data catalog for the modern data stack. Open Source DataHub is running in production at several companies like Peloton, Optum, Udemy, Zynga and others. Acryl Data provides DataHub as an easy to consume SaaS product which has been adopted by several companies. Signup for the SaaS product at dataengineeringpodcast.com/acryl
- RudderStack helps you build a customer data platform on your warehouse or data lake. Instead of trapping data in a black box, they enable you to easily collect customer data from the entire stack and build an identity graph on your warehouse, giving you full visibility and control. Their SDKs make event streaming from any app or website easy, and their state-of-the-art reverse ETL pipelines enable you to send enriched data to any cloud tool. Sign up free... or just get the free t-shirt for being a listener of the Data Engineering Podcast at dataengineeringpodcast.com/rudder.
- Your host is Tobias Macey and today I’m interviewing Demetrios Brinkmann and David Aponte about what you need to know about MLOps as a data engineer
Interview
- Introduction
- How did you get involved in the area of data management?
- Can you describe what MLOps is?
- How does it relate to DataOps? DevOps? (is it just another buzzword?)
- What is your interest and involvement in the space of MLOps?
- What are the open and active questions in the MLOps community?
- Who is responsible for MLOps in an organization?
- What is the role of the data engineer in that process?
- What are the core capabilities that are necessary to support an "MLOps" workflow?
- How do the current platform technologies support the adoption of MLOps workflows?
- What are the areas that are currently underdeveloped/underserved?
- Can you describe the technical and organizational design/architecture decisions that need to be made when endeavoring to adopt MLOps practices?
- What are some of the common requirements for supporting ML workflows?
- What are some of the ways that requirements become bespoke to a given organization or project?
- What are the opportunities for standardization or consolidation in the tooling for MLOps?
- What are the pieces that are always going to require custom engineering?
- What are the most interesting, innovative, or unexpected approaches to MLOps workflows/platforms that you have seen?
- What are the most interesting, unexpected, or challenging lessons that you have learned while working on supporting the MLOps community?
- What are your predictions for the future of MLOps?
- What are you keeping a close eye on?
Contact Info
- Demetrios
Data Engineering Podcast - DataOps As A Service For Your Data Integration Workflows With Rivery
Transcript
Hello, and welcome to the Data Engineering Podcast, the show about modern data management.
Have you ever woken up to a crisis because a number on a dashboard is broken and no 1 knows why? Or sent out frustrating Slack messages trying to find the right dataset? Or tried to understand what a column name means?
Our friends at Outland started out as a data team themselves and faced all this collaboratio
If you like this episode you’ll love
Episode Comments
Generate a badge
Get a badge for your website that links back to this episode
<a href="https://goodpods.com/podcasts/data-engineering-podcast-203077/dataops-as-a-service-for-your-data-integration-workflows-with-rivery-20706339"> <img src="https://storage.googleapis.com/goodpods-images-bucket/badges/generic-badge-1.svg" alt="listen to dataops as a service for your data integration workflows with rivery on goodpods" style="width: 225px" /> </a>
Copy