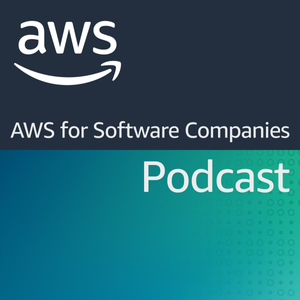
Ep077: Developing an AI Strategy for Software Companies
02/04/25 • 25 min
In this AWS panel discussion, Naveen Rao, VP of AI of Databricks and Vijay Karunamurthy, Field CTO of Scale AI share practical insights on implementing generative AI in enterprises, leveraging private data effectively, and building reliable production systems.
Topics Include:
- Sherry Marcus introduces panel discussion on generative AI adoption
- Scale AI helps make AI models more reliable
- Databricks focuses on customizing AI with company data
- Companies often stressed about where to start with AI
- Board-level pressure driving many enterprise AI initiatives
- Start by defining specific goals and success metrics
- Build evaluations first before implementing AI solutions
- Avoid rushing into demos without proper planning
- Enterprise data vastly exceeds public training data volume
- Customer support histories valuable for AI training
- Models learning to anticipate customer follow-up questions
- Production concerns: cost, latency, and accuracy trade-offs
- Good telemetry crucial for diagnosing AI application issues
- Speed matters more for prose, accuracy for legal documents
- Cost becomes important once systems begin scaling up
- Organizations struggle with poor quality existing data
- Privacy crucial when leveraging internal business data
- Role-based access control essential for regulated industries
- AI can help locate relevant data across legacy systems
- Models need organizational awareness to find data effectively
- Private data behind firewalls most valuable for AI
- Customization gives competitive advantage over generic models
- Current AI models primarily do flexible data recall
- Next few years: focus on deriving business value
- Future developments in causal inference expected post-5 years
- Complex multi-agent systems becoming more important
- Scale AI developing "humanity's last exam" evaluation metric
- Discussion of responsibility and liability in AI decisions
- Companies must stand behind their AI system outputs
- Existing compliance frameworks can be adapted for AI
Participants:
- Naveen Rao – VP of AI, Databricks
- Vijay Karunamurthy – Field CTO, Scale AI
- Sherry Marcus Ph.D. - Director, Applied Science, AWS
See how Amazon Web Services gives you the freedom to migrate, innovate, and scale your software company at https://aws.amazon/isv/
In this AWS panel discussion, Naveen Rao, VP of AI of Databricks and Vijay Karunamurthy, Field CTO of Scale AI share practical insights on implementing generative AI in enterprises, leveraging private data effectively, and building reliable production systems.
Topics Include:
- Sherry Marcus introduces panel discussion on generative AI adoption
- Scale AI helps make AI models more reliable
- Databricks focuses on customizing AI with company data
- Companies often stressed about where to start with AI
- Board-level pressure driving many enterprise AI initiatives
- Start by defining specific goals and success metrics
- Build evaluations first before implementing AI solutions
- Avoid rushing into demos without proper planning
- Enterprise data vastly exceeds public training data volume
- Customer support histories valuable for AI training
- Models learning to anticipate customer follow-up questions
- Production concerns: cost, latency, and accuracy trade-offs
- Good telemetry crucial for diagnosing AI application issues
- Speed matters more for prose, accuracy for legal documents
- Cost becomes important once systems begin scaling up
- Organizations struggle with poor quality existing data
- Privacy crucial when leveraging internal business data
- Role-based access control essential for regulated industries
- AI can help locate relevant data across legacy systems
- Models need organizational awareness to find data effectively
- Private data behind firewalls most valuable for AI
- Customization gives competitive advantage over generic models
- Current AI models primarily do flexible data recall
- Next few years: focus on deriving business value
- Future developments in causal inference expected post-5 years
- Complex multi-agent systems becoming more important
- Scale AI developing "humanity's last exam" evaluation metric
- Discussion of responsibility and liability in AI decisions
- Companies must stand behind their AI system outputs
- Existing compliance frameworks can be adapted for AI
Participants:
- Naveen Rao – VP of AI, Databricks
- Vijay Karunamurthy – Field CTO, Scale AI
- Sherry Marcus Ph.D. - Director, Applied Science, AWS
See how Amazon Web Services gives you the freedom to migrate, innovate, and scale your software company at https://aws.amazon/isv/
Previous Episode
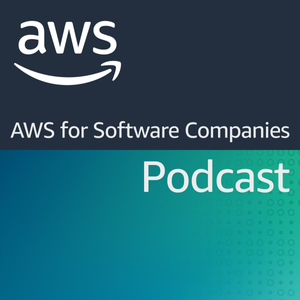
Ep076: Incident Response in the Age of Personal CISO Liability with Suresh Vasudevan of Sysdig
Suresh Vasudevan, CEO of Sysdig, discusses the evolving challenges of cloud security incident response and the need for new approaches to mitigate organizational risk.
Topics Include:
- Cybersecurity regulations mandate incident response reporting.
- Challenges of cloud breach detection and response.
- Complex cloud attack patterns: reconnaissance, lateral movement, exploit.
- Rapid exploitation - minutes vs. days for on-prem.
- Importance of runtime, identity, and control plane monitoring.
- Limitations of EDR and SIEM tools for cloud.
- Coordinated incident response across security, DevOps, executives.
- Criticality of pre-defined incident response plans.
- Increased CISO personal liability risk and mitigation.
- Documenting security team's diligence to demonstrate due care.
- Establishing strong partnerships with legal and audit teams.
- Covering defensive steps in internal communications.
- Sysdig's cloud-native security approach and Falco project.
- Balancing prevention, detection, and response capabilities.
- Integrating security tooling with customer workflows and SOCs.
- Providing 24/7 monitoring and rapid response services.
- Correlating workload, identity, and control plane activities.
- Detecting unusual reconnaissance and lateral movement behaviors.
- Daisy-chaining events to identify potential compromise chains.
- Tracking historical identity activity patterns for anomaly detection.
- Aligning security with business impact assessment and reporting.
- Adapting SOC team skills for cloud-native environments.
- Resource and disruption cost concerns for cloud agents.
- Importance of "do no harm" philosophy for response.
- Enhancing existing security data sources with cloud context.
- Challenges of post-incident forensics vs. real-time response.
- Bridging security, DevOps, and executive domains.
- Establishing pre-approved incident response stakeholder roles.
- Maintaining documentation to demonstrate proper investigation.
- Evolving CISO role and personal liability considerations.
- Proactive management of cyber risk at board level.
- Developing strong general counsel and audit relationships.
- Transparency in internal communications to avoid discovery risks.
- Security teams as business partners, not just technicians.
- Sysdig's cloud security expertise and open-source contributions.
Participants:
· Suresh Vasudevan – CEO, Sysdig
See how Amazon Web Services gives you the freedom to migrate, innovate, and scale your software company at https://aws.amazon/isv/
Next Episode
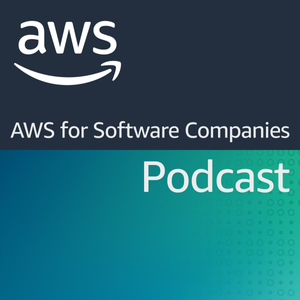
Ep078: Scaling Through Partnerships: Snowflake's Cloud Engineering Success
Through case studies of Graviton implementation and GPU integration, Justin Fitzhugh, Snowflake’s VP of Engineering, demonstrates how cloud-native architecture combined with strategic partnerships can drive technical innovation and build business value.
Topics Include:
- Cloud engineering and AWS partnership
- Traditional databases had fixed hardware ratios for compute/storage
- Snowflake built cloud-native with separated storage and compute
- Company has never owned physical infrastructure
- Applications must be cloud-optimized to leverage elastic scaling
- Snowflake uses credit system for customer billing
- Credits loosely based on compute resources provided
- Company maintains cloud-agnostic approach across providers
- Initially aimed for identical pricing across cloud providers
- Now allows price variation while maintaining consistent experience
- Consumption-based revenue model ties to actual usage
- Performance improvements can actually decrease revenue
- Company tracked ARM's move to data centers
- Initially skeptical of Graviton performance claims
- Porting to ARM required complete pipeline reconstruction
- Discovered floating point rounding differences between architectures
- Amazon partnership crucial for library optimization
- Graviton migration took two years instead of one
- Achieved 25% performance gain with 20% cost reduction
- Team requested thousands of GPUs within two months
- GPU infrastructure was new territory for Snowflake
- Needed flexible pricing for uncertain future needs
- Signed three to five-year contracts with flexibility
- Team pivoted from building to fine-tuning models
- Partnership allowed adaptation to business changes
- Emphasizes importance of leveraging provider expertise
- Recommends early engagement with cloud providers
- Build relationships before infrastructure needs arise
- Maintain personal connections with provider executives
Participants:
- Justin Fitzhugh – VP of Engineering, Snowflake
See how Amazon Web Services gives you the freedom to migrate, innovate, and scale your software company at https://aws.amazon/isv/
If you like this episode you’ll love
Episode Comments
Generate a badge
Get a badge for your website that links back to this episode
<a href="https://goodpods.com/podcasts/aws-for-software-companies-podcast-448037/ep077-developing-an-ai-strategy-for-software-companies-83613239"> <img src="https://storage.googleapis.com/goodpods-images-bucket/badges/generic-badge-1.svg" alt="listen to ep077: developing an ai strategy for software companies on goodpods" style="width: 225px" /> </a>
Copy