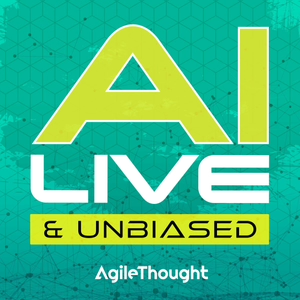
Causality and Artificial Intelligence with Arni Steingrimsson
03/11/22 • 36 min
Dr. Jerry Smith welcomes you to another episode of AI Live and Unbiased to explore the breadth and depth of Artificial Intelligence and to encourage you to change the world, not just observe it!
Dr. Jerry is joined today by Arni Steingrimsson, a Data Science Machine Learning and Artificial Intelligence in the U.S. and Mexico. He is a senior-level Data Scientist, who comes from a biomedical field. Arnie and Dr. Jerry are talking today about Causality and the crucial role it plays in the AI space.
Key Takeaways:
- What is Causality? Why is it important to Artificial Intelligence?
-
- Causality is what is causing the outcome; from a data perspective there are certain features that will be causal to the outcome but there is no guarantee that you can change the outcome by changing those features.
- Defining causality is less important than knowing what is capable.
- Granger causality is defined as a statistical dependence.
- Judea Pearl proposes three levels of causality: Association, Intervention, and Counterfactual.
- Why it is important to actually know the cause of something?
-
- People who want to be ahead and business leaders need to know how they can influence their decisions and make a change, that is why knowing the causality is crucial.
- Counterfactual Causality explains the connection between x and y, but y does not really change the possibility for x to occur or not to occur.
-
- What are counterfactuals? They are a comparison of different states in the same world, but how do you quantitatively compute these two states? It is done by holding to a variable.
- Simpson’s paradox: Something observed at a high level is counter to the thing observed at a low level.
-
- Simpson’s paradox is usually overlooked.
- The study of data is an important part of the causality world.
- Using machine learning in the world of causality:
-
- There are some data scientists that didn’t study causality, and they think that they can just use classical machine learning, isolating features, and feature reduction and that means using causality... but that is not the way of “changing the world”; you need to know why certain inputs changed and what caused this change.
- A reported driver is different than a causal driver.
- The application of Evolutionary principles in the AI world:
-
- The predictors are the blocks that put those inputs which are causal; this way we know the causal input to then create the machine learning model that will tell what will happen as a result of the given inputs but it does not tell us what we should set those inputs to.
- First, we figure out what is causal and make a model for that, then once we have this model of the world, we tell people what conditions need to be set to get the best chances of achieving your outcome.
- What kinds of tools are used for evolutionary computing? Python and their library called Deep.
- What can be done after simulation? What is next?
-
- After simulation, we need to take the inputs that represent causal drivers and put them into action in the field to monitor the change.
- If you want to improve your product you need to put programs (such as marketing and sales efforts) out and collect the data on them, how they are improving and what are the changes.
Stay Connected with AI Live and Unbiased:
Visit our website AgileThought.com
Email your thoughts or suggestions to [email protected] or Tweet @AgileThought using #AgileThoughtPodcast!
Learn more about Dr. Jerry Smith
Mentioned in this episode:
Causality: Models, Reasoning, and Inference, by Judea PearlDr. Jerry Smith welcomes you to another episode of AI Live and Unbiased to explore the breadth and depth of Artificial Intelligence and to encourage you to change the world, not just observe it!
Dr. Jerry is joined today by Arni Steingrimsson, a Data Science Machine Learning and Artificial Intelligence in the U.S. and Mexico. He is a senior-level Data Scientist, who comes from a biomedical field. Arnie and Dr. Jerry are talking today about Causality and the crucial role it plays in the AI space.
Key Takeaways:
- What is Causality? Why is it important to Artificial Intelligence?
-
- Causality is what is causing the outcome; from a data perspective there are certain features that will be causal to the outcome but there is no guarantee that you can change the outcome by changing those features.
- Defining causality is less important than knowing what is capable.
- Granger causality is defined as a statistical dependence.
- Judea Pearl proposes three levels of causality: Association, Intervention, and Counterfactual.
- Why it is important to actually know the cause of something?
-
- People who want to be ahead and business leaders need to know how they can influence their decisions and make a change, that is why knowing the causality is crucial.
- Counterfactual Causality explains the connection between x and y, but y does not really change the possibility for x to occur or not to occur.
-
- What are counterfactuals? They are a comparison of different states in the same world, but how do you quantitatively compute these two states? It is done by holding to a variable.
- Simpson’s paradox: Something observed at a high level is counter to the thing observed at a low level.
-
- Simpson’s paradox is usually overlooked.
- The study of data is an important part of the causality world.
- Using machine learning in the world of causality:
-
- There are some data scientists that didn’t study causality, and they think that they can just use classical machine learning, isolating features, and feature reduction and that means using causality... but that is not the way of “changing the world”; you need to know why certain inputs changed and what caused this change.
- A reported driver is different than a causal driver.
- The application of Evolutionary principles in the AI world:
-
- The predictors are the blocks that put those inputs which are causal; this way we know the causal input to then create the machine learning model that will tell what will happen as a result of the given inputs but it does not tell us what we should set those inputs to.
- First, we figure out what is causal and make a model for that, then once we have this model of the world, we tell people what conditions need to be set to get the best chances of achieving your outcome.
- What kinds of tools are used for evolutionary computing? Python and their library called Deep.
- What can be done after simulation? What is next?
-
- After simulation, we need to take the inputs that represent causal drivers and put them into action in the field to monitor the change.
- If you want to improve your product you need to put programs (such as marketing and sales efforts) out and collect the data on them, how they are improving and what are the changes.
Stay Connected with AI Live and Unbiased:
Visit our website AgileThought.com
Email your thoughts or suggestions to [email protected] or Tweet @AgileThought using #AgileThoughtPodcast!
Learn more about Dr. Jerry Smith
Mentioned in this episode:
Causality: Models, Reasoning, and Inference, by Judea PearlPrevious Episode
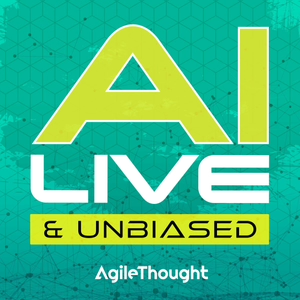
AI and the Democratization of Data of with Alonso Castañeda Andrade
Dr. Jerry Smith welcomes you to another episode of AI Live and Unbiased to explore the breadth and depth of Artificial Intelligence and to encourage you to change the world, not just observe it!
Dr. Jerry is joined today by Alonso Castañeda Andrade, who is the Managing Director of Data Engineering and Analytics at Agile Thought. Dr. Jerry and Alonso are talking today about the role that Data Engineering and Analytics play in AI.
Key Takeaways:
- Why is it a challenge today to create quality data products?
-
- Technically a lot of tools are available today for databases, the cloud allows us to scale quickly to be able to manage all the data, but most of the challenges come from the organizational aspect and processes, which involve the dynamic nature of the data.
- You can do AI without having data. Where do we start to create good quality data?
-
- Data is available in a variety of forms and places.
- Organizing data is a challenging job and tools are needed to assist the Data Engineer to perform his role, like having a good architecture platform for data and having a well-defined flow of information.
- Once we have the organized data, analytics can be run on them.
- What are customers looking for out of their dashboards? What are they really looking to get out of their analytic solutions?
-
- Data Engineering and Analytics are asked to work on the integration of systems.
- Customers expect their business to gain more visibility.
- Customers want to receive trusted data in a timely manner.
- The analytic team, dashboard engineers, and data scientists need to work together for better outcomes.
-
- The democratization of the data: How do we enable everyone in the company to have access to the data that they require, and do that by themselves without depending on others?
- Trends for 2022: The continuous migration to the cloud.
-
- Clouds play a very important role in the data modernization of platforms since they allow businesses to deploy data products faster (up to 50% velocity increase).
- Services become really significant, especially the cognitive services and analytical databases.
- Business requires their data as soon as possible when something happens, for example, five minutes in the banking industry for fraud can cost millions of dollars.
- What is going on today in the world of Data apps?
-
- One of the challenges is to provide the data that the business requires in a timely manner, generally traditional analytics have been waterfall in nature, bringing all the data to create a massive data model; many fail in this process since it is time-consuming and expensive, and once they are ready the data may be obsolete.
- Data is an asset of an organization and being able to make that into a competitive advantage is key.
Stay Connected with AI Live and Unbiased:
Visit our website AgileThought.com
Email your thoughts or suggestions to [email protected] or Tweet @AgileThought using #AgileThoughtPodcast!
Learn more about Dr. Jerry Smith
Next Episode
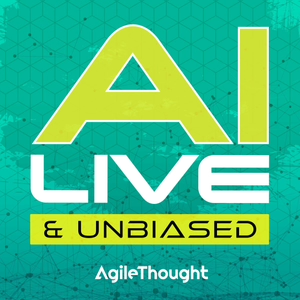
Digital Transformation in the World of Causal AI with Dr. Jerry Smith
Dr. Jerry Smith welcomes you to another episode of AI Live and Unbiased to explore the breadth and depth of Artificial Intelligence and to encourage you to change the world, not just observe it!
Dr. Jerry is talking today about Digital Transformation in the new world of Causal AI. Dr. Jerry has spent many years within the area of computer science, technology, data science, machine learning, and AI, seeking always a way to do a better job of decoupling the brilliant successes of marketing and making them even more usable.
Key Takeaways:
- Digital transformation is failing business expectations.
-
- More than 60% of CEOs believe that the digital transformation did not meet their expectations.
- Digital Transformation is not the same thing as transforming you digitally.
-
- Digital transformation needs to be driven by the will to change a company by changing aspects of its people.
- If you don’t like what you see in the company’s data, to see a change in that data, you need to change people.
- The most important is not about the products a company sells but about the behavior people have with those products.
- Causal AI asks: Why do people do what they do?
-
- Why don’t people buy a company’s products or services?
- Dr. Jerry shares the example of why people buy certain Spaghetti Sauce.
- To change human behavior the first step is to collect information, which is a cost for any company that decides to do this.
- Secondly, what to do with that data? Cognitive and emotional services are what come next in order to interpret that data by creating columns of variables and rows of observations.
- What can be done with that digital surrogate that was created? Optimization!
- How to Optimize:
-
- Two ways to optimize models are Particle Swarm Optimization or Evolutionary Computing.
- Evolutionary computing has the capacity to take suboptimal solutions and combine them to produce an optimal response.
- Data Science, Machine Learning, and AI are different.
-
- Data Science is the science of data, it is about how we look at data from a scientific perspective to get insights and learn from it.
- Machine learning is predicting the future state for something based upon the current state of that matter.
- AI is artificial intelligence; artificial to human beings. AI is about the decision-making process based on predictions of an outcome.
- Not all data is the same.
- Digital psychologists, sociologists, and anthropologists help transform and codify human behavior.
Stay Connected with AI Live and Unbiased:
Visit our website AgileThought.com
Email your thoughts or suggestions to [email protected] or Tweet @AgileThought using #AgileThoughtPodcast!
Learn more about Dr. Jerry Smith
If you like this episode you’ll love
Episode Comments
Generate a badge
Get a badge for your website that links back to this episode
<a href="https://goodpods.com/podcasts/ai-live-and-unbiased-426656/causality-and-artificial-intelligence-with-arni-steingrimsson-58738456"> <img src="https://storage.googleapis.com/goodpods-images-bucket/badges/generic-badge-1.svg" alt="listen to causality and artificial intelligence with arni steingrimsson on goodpods" style="width: 225px" /> </a>
Copy