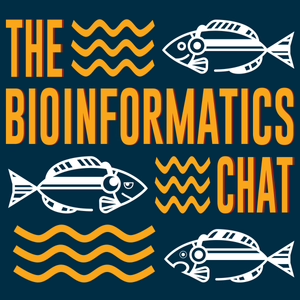
#69 Suffix arrays in optimal compressed space and δ-SA with Tomasz Kociumaka and Dominik Kempa
09/29/23 • 56 min
Today on the podcast we have Tomasz Kociumaka and Dominik Kempa, the authors of the preprint Collapsing the Hierarchy of Compressed Data Structures: Suffix Arrays in Optimal Compressed Space.
The suffix array is one of the foundational data structures in bioinformatics, serving as an index that allows fast substring searches in a large text. However, in its raw form, the suffix array occupies the space proportional to (and several times larger than) the original text.
In their paper, Tomasz and Dominik construct a new index, δ-SA, which on the one hand can be used in the same way (answer the same queries) as the suffix array and the inverse suffix array, and on the other hand, occupies the space roughly proportional to the gzip’ed text (or, more precisely, to the measure δ that they define — hence the name).
Moreover, they mathematically prove that this index is optimal, in the sense that any index that supports these queries — or even much weaker queries, such as simply accessing the i-th character of the text — cannot be significantly smaller (as a function of δ) than δ-SA.
Links:
- Collapsing the Hierarchy of Compressed Data Structures: Suffix Arrays in Optimal Compressed Space (Dominik Kempa, Tomasz Kociumaka)
Thank you to Jake Yeung and other Patreon members for supporting this episode.
Today on the podcast we have Tomasz Kociumaka and Dominik Kempa, the authors of the preprint Collapsing the Hierarchy of Compressed Data Structures: Suffix Arrays in Optimal Compressed Space.
The suffix array is one of the foundational data structures in bioinformatics, serving as an index that allows fast substring searches in a large text. However, in its raw form, the suffix array occupies the space proportional to (and several times larger than) the original text.
In their paper, Tomasz and Dominik construct a new index, δ-SA, which on the one hand can be used in the same way (answer the same queries) as the suffix array and the inverse suffix array, and on the other hand, occupies the space roughly proportional to the gzip’ed text (or, more precisely, to the measure δ that they define — hence the name).
Moreover, they mathematically prove that this index is optimal, in the sense that any index that supports these queries — or even much weaker queries, such as simply accessing the i-th character of the text — cannot be significantly smaller (as a function of δ) than δ-SA.
Links:
- Collapsing the Hierarchy of Compressed Data Structures: Suffix Arrays in Optimal Compressed Space (Dominik Kempa, Tomasz Kociumaka)
Thank you to Jake Yeung and other Patreon members for supporting this episode.
Previous Episode
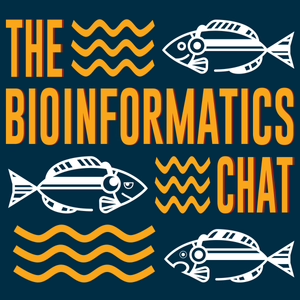
#68 Phylogenetic inference from raw reads and Read2Tree with David Dylus
In this episode, David Dylus talks about Read2Tree, a tool that builds alignment matrices and phylogenetic trees from raw sequencing reads. By leveraging the database of orthologous genes called OMA, Read2Tree bypasses traditional, time-consuming steps such as genome assembly, annotation and all-versus-all sequence comparisons.
Links:
- Inference of phylogenetic trees directly from raw sequencing reads using Read2Tree (David Dylus, Adrian Altenhoff, Sina Majidian, Fritz J. Sedlazeck, Christophe Dessimoz)
- Background story
- Read2Tree on GitHub
- OMA browser
- The Guardian’s podcast about Victoria Amelina and Volodymyr Vakulenko
If you enjoyed this episode, please consider supporting the podcast on Patreon.
Next Episode
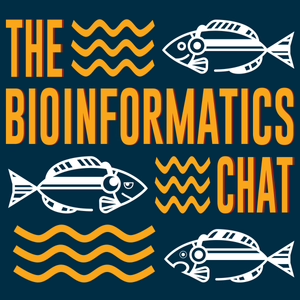
#70 Prioritizing drug target genes with Marie Sadler
In this episode, Marie Sadler talks about her recent Cell Genomics paper, Multi-layered genetic approaches to identify approved drug targets.
Previous studies have found that the drugs that target a gene linked to the disease are more likely to be approved. Yet there are many ways to define what it means for a gene to be linked to the disease. Perhaps the most straightforward approach is to rely on the genome-wide association studies (GWAS) data, but that data can also be integrated with quantitative trait loci (eQTL or pQTL) information to establish less obvious links between genetic variants (which often lie outside of genes) and genes. Finally, there’s exome sequencing, which, unlike GWAS, captures rare genetic variants. So in this paper, Marie and her colleagues set out to benchmark these different methods against one another.
Listen to the episode to find out how these methods work, which ones work better, and how network propagation can improve the prediction accuracy.
Links:
- Multi-layered genetic approaches to identify approved drug targets (Marie C. Sadler, Chiara Auwerx, Patrick Deelen, Zoltán Kutalik)
- Marie on GitHub
- Interview with Mariana Mamonova, the Ukrainian marine infantry combat medic who spent 6 months in russian captivity while pregnant
Thank you to Jake Yeung, Michael Weinstein, and other Patreon members for supporting this episode.
If you like this episode you’ll love
Episode Comments
Generate a badge
Get a badge for your website that links back to this episode
<a href="https://goodpods.com/podcasts/the-bioinformatics-chat-292186/69-suffix-arrays-in-optimal-compressed-space-and-%ce%b4-sa-with-tomasz-koci-38251720"> <img src="https://storage.googleapis.com/goodpods-images-bucket/badges/generic-badge-1.svg" alt="listen to #69 suffix arrays in optimal compressed space and δ-sa with tomasz kociumaka and dominik kempa on goodpods" style="width: 225px" /> </a>
Copy