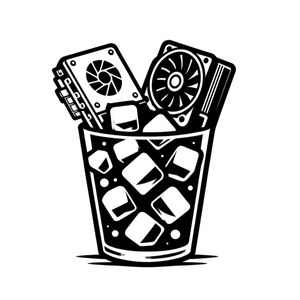
Tech on the Rocks
Kostas, Nitay
All episodes
Best episodes
Top 10 Tech on the Rocks Episodes
Goodpods has curated a list of the 10 best Tech on the Rocks episodes, ranked by the number of listens and likes each episode have garnered from our listeners. If you are listening to Tech on the Rocks for the first time, there's no better place to start than with one of these standout episodes. If you are a fan of the show, vote for your favorite Tech on the Rocks episode by adding your comments to the episode page.
FAQ
How many episodes does Tech on the Rocks have?
Tech on the Rocks currently has 12 episodes available.
What topics does Tech on the Rocks cover?
The podcast is about Infrastructure, Cloud, Data, Podcasts and Technology.
What is the most popular episode on Tech on the Rocks?
The episode title 'Proving Code Correctness: FizzBee and the Future of Formal Methods in Software Design with FizzBee's creator JP' is the most popular.
What is the average episode length on Tech on the Rocks?
The average episode length on Tech on the Rocks is 61 minutes.
How often are episodes of Tech on the Rocks released?
Episodes of Tech on the Rocks are typically released every 14 days, 13 hours.
When was the first episode of Tech on the Rocks?
The first episode of Tech on the Rocks was released on Aug 23, 2024.
Show more FAQ

Show more FAQ