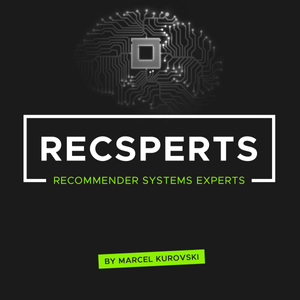
#9: RecPack and Modularized Personalization by Froomle with Lien Michiels and Robin Verachtert
Recsperts - Recommender Systems Experts09/15/22 • 87 min
In episode number nine of Recsperts we talk with the creators of RecPack which is a new Python package for recommender systems. We discuss how Froomle provides modularized personalization for customers in the news and e-commerce sectors. I talk to Lien Michiels and Robin Verachtert who are both industrial PhD students at the University of Antwerp and who work for Froomle. We also hear about their research on filter bubbles as well as model drift along with their RecSys 2022 contributions.
In this episode we introduce RecPack as a new recommender package that is easy to use and to extend and which allows for consistent experimentation. Lien and Robin share with us how RecPack evolved, its structure as well as the problems in research and practice they intend to solve with their open source contribution.
My guests also share many insights from their work at Froomle where they focus on modularized personalization with more than 60 recommendation scenarios and how they integrate these with their customers. We touch on topics like model drift and the need for frequent retraining as well as on the tradeoffs between accuracy, cost, and timeliness in production recommender systems.
In the end we also exchange about Lien's critical reception of using the term 'filter bubble', an operationalized definition of them as well as Robin's research on model degradation and training data selection.
Enjoy this enriching episode of RECSPERTS - Recommender Systems Experts.
Links from the Episode:
- Lien Michiels on LinkedIn
- Lien Michiels on Twitter
- Robin Verachtert on LinkedIn
- RecPack on GitLab
- RecPack Documentation
- FROOMLE
- PERSPECTIVES 2022: Perspectives on the Evaluation of Recommender Systems
- PERSPECTIVES 2022: Preview on "Towards a Broader Perspective in Recommender Evaluation" by Benedikt Loepp
- 5th FAccTRec Workshop: Responsible Recommendation
Papers:
- Verachtert et al. (2022): Are We Forgetting Something? Correctly Evaluate a Recommender System With an Optimal Training Window
- Leysen and Michiels et al. (2022): What Are Filter Bubbles Really? A Review of the Conceptual and Empirical Work
- Michiels and Verachtert et al. (2022): RecPack: An(other) Experimentation Toolkit for Top-N Recommendation using Implicit Feedback Data
- Dahlgren (2021): A critical review of filter bubbles and a comparison with selective exposure
General Links:
- Follow me on Twitter: https://twitter.com/LivesInAnalogia
- Send me your comments, questions and suggestions to [email protected]
- Podcast Website: https://www.recsperts.com/
- (03:23) - Introduction Lien Michiels
- (07:01) - Introduction Robin Verachtert
- (09:29) - RecPack - Python Recommender Package
- (52:31) - Modularized Personalization in News and E-commerce by Froomle
- (01:09:54) - Research on Model Drift and Filter Bubbles
- (01:18:07) - Closing Questions
09/15/22 • 87 min
Generate a badge
Get a badge for your website that links back to this episode
<a href="https://goodpods.com/podcasts/recsperts-recommender-systems-experts-537441/9-recpack-and-modularized-personalization-by-froomle-with-lien-michiel-69383372"> <img src="https://storage.googleapis.com/goodpods-images-bucket/badges/generic-badge-1.svg" alt="listen to #9: recpack and modularized personalization by froomle with lien michiels and robin verachtert on goodpods" style="width: 225px" /> </a>
Copy