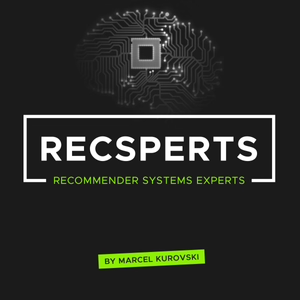
#28: Multistakeholder Recommender Systems with Robin Burke
04/15/25 • 95 min
In episode 28 of Recsperts, I sit down with Robin Burke, professor of information science at the University of Colorado Boulder and a leading expert with over 30 years of experience in recommender systems. Together, we explore multistakeholder recommender systems, fairness, transparency, and the role of recommender systems in the age of evolving generative AI.
We begin by tracing the origins of recommender systems, traditionally built around user-centric models. However, Robin challenges this perspective, arguing that all recommender systems are inherently multistakeholder—serving not just consumers as the recipients of recommendations, but also content providers, platform operators, and other key players with partially competing interests. He explains why the common “Recommended for You” label is, at best, an oversimplification and how greater transparency is needed to show how stakeholder interests are balanced.
Our conversation also delves into practical approaches for handling multiple objectives, including reranking strategies versus integrated optimization. While embedding multistakeholder concerns directly into models may be ideal, reranking offers a more flexible and efficient alternative, reducing the need for frequent retraining.
Towards the end of our discussion, we explore post-userism and the impact of generative AI on recommendation systems. With AI-generated content on the rise, Robin raises a critical concern: if recommendation systems remain overly user-centric, generative content could marginalize human creators, diminishing their revenue streams.
Enjoy this enriching episode of RECSPERTS - Recommender Systems Experts.
Don't forget to follow the podcast and please leave a review
- (00:00) - Introduction
- (03:24) - About Robin Burke and First Recommender Systems
- (26:07) - From Fairness and Advertising to Multistakeholder RecSys
- (34:10) - Multistakeholder RecSys Terminology
- (40:16) - Multistakeholder vs. Multiobjective
- (42:43) - Reciprocal and Value-Aware RecSys
- (59:14) - Objective Integration vs. Reranking
- (01:06:31) - Social Choice for Recommendations under Fairness
- (01:17:40) - Post-Userist Recommender Systems
- (01:26:34) - Further Challenges and Closing Remarks
Links from the Episode:
- Robin Burke on LinkedIn
- Robin's Website
- That Recommender Systems Lab
- Reference to Broder's Keynote on Computational Advertising and Recommender Systems from RecSys 2008
- Multistakeholder Recommender Systems (from Recommender Systems Handbook), chapter by Himan Abdollahpouri & Robin Burke
- POPROX: The Platform for OPen Recommendation and Online eXperimentation
- AltRecSys 2024 (Workshop at RecSys 2024)
Papers:
- Burke et al. (1996): Knowledge-Based Navigation of Complex Information Spaces
- Burke (2002): Hybrid Recommender Systems: Survey and Experiments
- Resnick et al. (1997): Recommender Systems
- Goldberg et al. (1992): Using collaborative filtering to weave an information tapestry
- Linden et al. (2003): Amazon.com Recommendations - Item-to-Item Collaborative Filtering
- Aird et al. (2024): Social Choice for Heterogeneous Fairness in Recommendation
- Aird et al. (2024): Dynamic Fairness-aware Recommendation Through Multi-agent Social Choice
- Burke et al. (2024): Post-Userist Recommender Systems : A Manifesto
- Baumer et al. (2017): Post-userism
- Burke et al. (2024): Conducting Recommender Systems User Studies Using POPROX
General Links:
- Follow me on
In episode 28 of Recsperts, I sit down with Robin Burke, professor of information science at the University of Colorado Boulder and a leading expert with over 30 years of experience in recommender systems. Together, we explore multistakeholder recommender systems, fairness, transparency, and the role of recommender systems in the age of evolving generative AI.
We begin by tracing the origins of recommender systems, traditionally built around user-centric models. However, Robin challenges this perspective, arguing that all recommender systems are inherently multistakeholder—serving not just consumers as the recipients of recommendations, but also content providers, platform operators, and other key players with partially competing interests. He explains why the common “Recommended for You” label is, at best, an oversimplification and how greater transparency is needed to show how stakeholder interests are balanced.
Our conversation also delves into practical approaches for handling multiple objectives, including reranking strategies versus integrated optimization. While embedding multistakeholder concerns directly into models may be ideal, reranking offers a more flexible and efficient alternative, reducing the need for frequent retraining.
Towards the end of our discussion, we explore post-userism and the impact of generative AI on recommendation systems. With AI-generated content on the rise, Robin raises a critical concern: if recommendation systems remain overly user-centric, generative content could marginalize human creators, diminishing their revenue streams.
Enjoy this enriching episode of RECSPERTS - Recommender Systems Experts.
Don't forget to follow the podcast and please leave a review
- (00:00) - Introduction
- (03:24) - About Robin Burke and First Recommender Systems
- (26:07) - From Fairness and Advertising to Multistakeholder RecSys
- (34:10) - Multistakeholder RecSys Terminology
- (40:16) - Multistakeholder vs. Multiobjective
- (42:43) - Reciprocal and Value-Aware RecSys
- (59:14) - Objective Integration vs. Reranking
- (01:06:31) - Social Choice for Recommendations under Fairness
- (01:17:40) - Post-Userist Recommender Systems
- (01:26:34) - Further Challenges and Closing Remarks
Links from the Episode:
- Robin Burke on LinkedIn
- Robin's Website
- That Recommender Systems Lab
- Reference to Broder's Keynote on Computational Advertising and Recommender Systems from RecSys 2008
- Multistakeholder Recommender Systems (from Recommender Systems Handbook), chapter by Himan Abdollahpouri & Robin Burke
- POPROX: The Platform for OPen Recommendation and Online eXperimentation
- AltRecSys 2024 (Workshop at RecSys 2024)
Papers:
- Burke et al. (1996): Knowledge-Based Navigation of Complex Information Spaces
- Burke (2002): Hybrid Recommender Systems: Survey and Experiments
- Resnick et al. (1997): Recommender Systems
- Goldberg et al. (1992): Using collaborative filtering to weave an information tapestry
- Linden et al. (2003): Amazon.com Recommendations - Item-to-Item Collaborative Filtering
- Aird et al. (2024): Social Choice for Heterogeneous Fairness in Recommendation
- Aird et al. (2024): Dynamic Fairness-aware Recommendation Through Multi-agent Social Choice
- Burke et al. (2024): Post-Userist Recommender Systems : A Manifesto
- Baumer et al. (2017): Post-userism
- Burke et al. (2024): Conducting Recommender Systems User Studies Using POPROX
General Links:
- Follow me on
Previous Episode
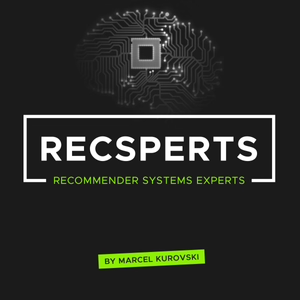
#27: Recommender Systems at the BBC with Alessandro Piscopo and Duncan Walker
In episode 27 of Recsperts, we meet Alessandro Piscopo, Lead Data Scientist in Personalization and Search, and Duncan Walker, Principal Data Scientist in the iPlayer Recommendations Team, both from the BBC. We discuss how the BBC personalizes recommendations across different offerings like news or video and audio content recommendations. We learn about the core values for the oldest public service media organization and the collaboration with editors in that process.
The BBC once started with short video recommendations for BBC+ and nowadays has to consider recommendations across multiple domains: news, the iPlayer, BBC Sounds, BBC Bytesize, and more. With a reach of about 500M+ users who access services every week there is a huge potential. My guests discuss the challenges of aligning recommendations with public service values and the role of editors and constant exchange, alignment, and learning between the algorithmic and editorial lines of recommender systems.
We also discuss the potential of cross-domain recommendations to leverage the content across different products as well as the organizational setup of teams working on recommender systems at the BBC. We learn about skews in the data due to the nature of an online service that also has a linear offering with TV and radio services.
Towards the end, we also touch a bit on QUARE @ RecSys, which is the Workshop on Measuring the Quality of Explanations in Recommender Systems.
Enjoy this enriching episode of RECSPERTS - Recommender Systems Experts.
Don't forget to follow the podcast and please leave a review
- (00:00) - Introduction
- (03:10) - About Alessandro Piscopo and Duncan Walker
- (14:53) - RecSys Applications at the BBC
- (20:22) - Journey of Building Public Service Recommendations
- (28:02) - Role and Implementation of Public Service Values
- (36:52) - Algorithmic and Editorial Recommendation
- (01:01:54) - Further RecSys Challenges at the BBC
- (01:15:53) - Quare Workshop
- (01:23:27) - Closing Remarks
Links from the Episode:
- Alessandro Piscopo on LinkedIn
- Duncan Walker on LinkedIn
- BBC
- QUARE @ RecSys 2023 (2nd Workshop on Measuring the Quality of Explanations in Recommender Systems)
Papers:
- Clarke et al. (2023): Personalised Recommendations for the BBC iPlayer: Initial approach and current challenges
- Boididou et al. (2021): Building Public Service Recommenders: Logbook of a Journey
- Piscopo et al. (2019): Data-Driven Recommendations in a Public Service Organisation
General Links:
- Follow me on LinkedIn
- Follow me on X
- Send me your comments, questions and suggestions to [email protected]
- Recsperts Website
If you like this episode you’ll love
Episode Comments
Generate a badge
Get a badge for your website that links back to this episode
<a href="https://goodpods.com/podcasts/recsperts-recommender-systems-experts-537441/28-multistakeholder-recommender-systems-with-robin-burke-89540380"> <img src="https://storage.googleapis.com/goodpods-images-bucket/badges/generic-badge-1.svg" alt="listen to #28: multistakeholder recommender systems with robin burke on goodpods" style="width: 225px" /> </a>
Copy