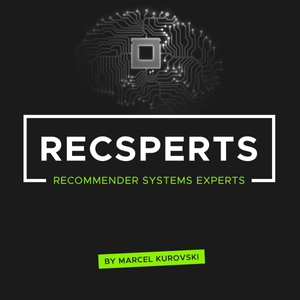
#11: Personalized Advertising, Economic and Generative Recommenders with Flavian Vasile
12/15/22 • 71 min
In this episode of Recsperts we talk to Flavian Vasile about the work of his team at Criteo AI Lab on personalized advertising. We learn about the different stakeholders like advertisers, publishers, and users and the role of recommender systems in this marketplace environment. We learn more about the pros and cons of click versus conversion optimization and transition to econ(omic) reco(mmendations), a new approach to model the effect of a recommendations system on the users' decision making process. Economic theory plays an important role for this conceptual shift towards better recommender systems.
In addition, we discuss generative recommenders as an approach to directly translate a user’s preference model into a textual and/or visual product recommendation. This can be used to spark product innovation and to potentially generate what users really want. Besides that, it also allows to provide recommendations from the existing item corpus.
In the end, we catch up on additional real-world challenges like two-tower models and diversity in recommendations.
Enjoy this enriching episode of RECSPERTS - Recommender Systems Experts.
Chapters:
- (02:37) - Introduction Flavian Vasile
- (06:46) - Personalized Advertising at Criteo
- (18:29) - Moving from Click to Conversion optimization
- (23:04) - Econ(omic) Reco(mmendations)
- (41:56) - Generative Recommender Systems
- (01:04:03) - Additional Real-World Challenges in RecSys
- (01:08:00) - Final Remarks
Links from the Episode:
- Flavian Vasile on LinkedIn
- Flavian Vasile on Twitter
- Modern Recommendation for Advanced Practitioners - Part I (2019)
- Modern Recommendation for Advanced Practitioners - Part II (2019)
- CONSEQUENCES+REVEAL Workshop at RecSys 2022: Causality, Counterfactuals, Sequential Decision-Making & Reinforcement Learning for Recommender Systems
Papers:
- Heymann et al. (2022): Welfare-Optimized Recommender Systems
- Samaran et al. (2021): What Users Want? WARHOL: A Generative Model for Recommendation
- Bonner et al (2018): Causal Embeddings for Recommendation
- Vasile et al. (2016): Meta-Prod2Vec: Product Embeddings Using Side-Information for Recommendation
General Links:
- Follow me on Twitter: https://twitter.com/LivesInAnalogia
- Send me your comments, questions and suggestions to [email protected]
- Podcast Website: https://www.recsperts.com/
In this episode of Recsperts we talk to Flavian Vasile about the work of his team at Criteo AI Lab on personalized advertising. We learn about the different stakeholders like advertisers, publishers, and users and the role of recommender systems in this marketplace environment. We learn more about the pros and cons of click versus conversion optimization and transition to econ(omic) reco(mmendations), a new approach to model the effect of a recommendations system on the users' decision making process. Economic theory plays an important role for this conceptual shift towards better recommender systems.
In addition, we discuss generative recommenders as an approach to directly translate a user’s preference model into a textual and/or visual product recommendation. This can be used to spark product innovation and to potentially generate what users really want. Besides that, it also allows to provide recommendations from the existing item corpus.
In the end, we catch up on additional real-world challenges like two-tower models and diversity in recommendations.
Enjoy this enriching episode of RECSPERTS - Recommender Systems Experts.
Chapters:
- (02:37) - Introduction Flavian Vasile
- (06:46) - Personalized Advertising at Criteo
- (18:29) - Moving from Click to Conversion optimization
- (23:04) - Econ(omic) Reco(mmendations)
- (41:56) - Generative Recommender Systems
- (01:04:03) - Additional Real-World Challenges in RecSys
- (01:08:00) - Final Remarks
Links from the Episode:
- Flavian Vasile on LinkedIn
- Flavian Vasile on Twitter
- Modern Recommendation for Advanced Practitioners - Part I (2019)
- Modern Recommendation for Advanced Practitioners - Part II (2019)
- CONSEQUENCES+REVEAL Workshop at RecSys 2022: Causality, Counterfactuals, Sequential Decision-Making & Reinforcement Learning for Recommender Systems
Papers:
- Heymann et al. (2022): Welfare-Optimized Recommender Systems
- Samaran et al. (2021): What Users Want? WARHOL: A Generative Model for Recommendation
- Bonner et al (2018): Causal Embeddings for Recommendation
- Vasile et al. (2016): Meta-Prod2Vec: Product Embeddings Using Side-Information for Recommendation
General Links:
- Follow me on Twitter: https://twitter.com/LivesInAnalogia
- Send me your comments, questions and suggestions to [email protected]
- Podcast Website: https://www.recsperts.com/
Previous Episode
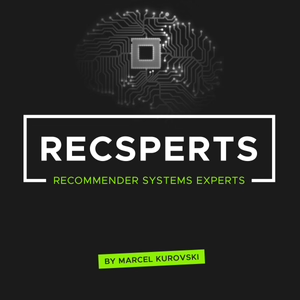
#10: Recommender Systems in Human Resources with David Graus
In episode number ten of Recsperts I welcome David Graus who is the Data Science Chapter Lead at Randstad Groep Nederland, a global leader in providing Human Resource services. We talk about the role of recommender systems in the HR domain which includes vacancy recommendations for candidates, but also generating talent recommendations for recruiters at Randstad. We also learn which biases might have an influence when using recommenders for decision support in the recruiting process as well as how Randstad mitigates them.
In this episode we learn more about another domain where recommender systems can serve humans by effective decision support: Human Resources. Here, everything is about job recommendations, matching candidates with vacancies, but also exploiting knowledge about career path to propose learning opportunities and assist with career development. David Graus leads those efforts at Randstad and has previously worked in the news recommendation domain after obtaining his PhD from the University of Amsterdam.
We discuss the most recent contribution by Randstad on mitigating bias in candidate recommender systems by introducing fairness-oriented post- and preprocessing to a recommendation pipeline. We learn that one can maintain user satisfaction while improving fairness at the same time (demographic parity measuring gender balance in this case).
David and I also touch on his engagement in co-organizing the RecSys in HR workshops since RecSys 2021.
Enjoy this enriching episode of RECSPERTS - Recommender Systems Experts.
Links from the Episode:
- David Graus on LinkedIn
- David Graus on Twitter
- David's Website
- RecSys in HR 2022: Workshop on Recommender Systems for Human Recources
- Randstad Annual Report 2021
- Talk by David Graus at Anti-Discrimination Hackaton on "Algorithmic matching, bias, and bias mitigation"
Papers:
- Arafan et al. (2022): End-to-End Bias Mitigation in Candidate Recommender Systems with Fairness Gates
- Geyik et al. (2019): Fairness-Aware Ranking in Search & Recommendation Systems with Application to LinkedIn Talent Search
General Links:
- Follow me on Twitter: https://twitter.com/LivesInAnalogia
- Send me your comments, questions and suggestions to [email protected]
- Podcast Website: https://www.recsperts.com/
- (02:23) - Introduction David Graus
- (13:55) - About Randstad and the Staffing Industry
- (17:09) - Use Cases for RecSys Application in HR
- (22:04) - Talent and Vacancy Recommender System
- (33:46) - RecSys in HR Workshop
- (38:48) - Fairness for RecSys in HR
- (52:40) - Other HR RecSys Challenges
- (56:40) - Further RecSys Challenges
Next Episode
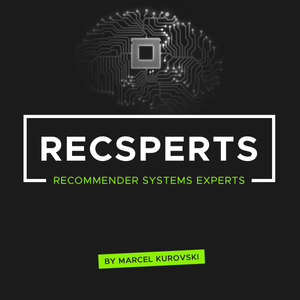
#12: From User Intent to Multi-Stakeholder Recommenders and Creator Economy with Rishabh Mehrotra
In this episode of Recsperts we talk to Rishabh Mehrotra, the Director of Machine Learning at ShareChat, about users and creators in multi-stakeholder recommender systems. We learn more about users intents and needs, which brings us to the important matter of user satisfaction (and dissatisfaction). To draw conclusions about user satisfaction we have to perceive real-time user interaction data conditioned on user intents. We learn that relevance does not imply satisfaction as well as that diversity and discovery are two very different concepts.
Rishabh takes us even further on his industry research journey where we also touch on relevance, fairness and satisfaction and how to balance them towards a fair marketplace. He introduces us into the creator economy of ShareChat. We discuss the post lifecycle of items as well as the right mixture of content and behavioral signals for generating recommendations that strike a balance between revenue and retention.
In the end, we also conclude our interview with the benefits of end-to-end ownership and accountability in industrial RecSys work and how it makes people independent and effective. We receive some advice for how to grow and strive in tough job market times.
Enjoy this enriching episode of RECSPERTS - Recommender Systems Experts.
Chapters:
- (03:44) - Introduction Rishabh Mehrotra
- (19:09) - Ubiquity of Recommender Systems
- (23:32) - Moving from UCL to Spotify Research
- (33:17) - Moving from Research to Engineering
- (36:33) - Recommendations in a Marketplace
- (46:24) - Discovery vs. Diversity and Specialists vs. Generalists
- (55:24) - User Intent, Satisfaction and Relevant Recommendations
- (01:09:48) - Estimation of Satisfaction vs. Dissatisfaction
- (01:19:10) - RecSys Challenges at ShareChat
- (01:27:58) - Post Lifecycle and Mixing Content with Behavioral Signals
- (01:39:28) - Detect Fatigue and Contextual MABs for Ad Placement
- (01:47:24) - Unblock Yourself and Upskill
- (02:00:59) - RecSys Challenge 2023 by ShareChat
- (02:02:36) - Farewell Remarks
Links from the Episode:
Papers:
- Mehrotra et al. (2017): Auditing Search Engines for Differential Satisfaction Across Demographics
- Mehrotra et al. (2018): Towards a Fair Marketplace: Counterfactual Evaluation of the trade-off between Relevance, Fairness & Satisfaction in Recommender Systems
- Mehrotra et al. (2019): Jointly Leveraging Intent and Interaction Signals to Predict User Satisfaction with Slate Recommendations
- Anderson et al. (2020): Algorithmic Effects on the Diversity of Consumption on Spotify
- Mehrotra et al. (2020): Bandit based Optimization of Multiple Objectives on a Music Streaming Platform
- Hansen et al. (2021): Shifting Consumption towards Diverse Content on Music Streaming Platforms
- Mehrotra (2021): Algorithmic Balancing of Familiarity, Similarity & Discovery in Music Recommendations
- Jeunen et al. (2022): Disentangling Causal Effects from Sets of Interventions in the Presence of Unobserved Confounders
General Links:
- Follow me on Twitter: https://twitter.com/LivesInAnalogia
- Send me your comments, questions and suggestions to [email protected]
- Podcast Website: https://www.recsperts.com/
If you like this episode you’ll love
Episode Comments
Generate a badge
Get a badge for your website that links back to this episode
<a href="https://goodpods.com/podcasts/recsperts-recommender-systems-experts-537441/11-personalized-advertising-economic-and-generative-recommenders-with-69383370"> <img src="https://storage.googleapis.com/goodpods-images-bucket/badges/generic-badge-1.svg" alt="listen to #11: personalized advertising, economic and generative recommenders with flavian vasile on goodpods" style="width: 225px" /> </a>
Copy