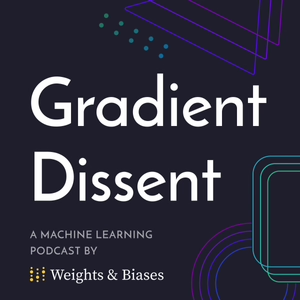
Richard Socher — The Challenges of Making ML Work in the Real World
09/29/20 • 50 min
Previous Episode
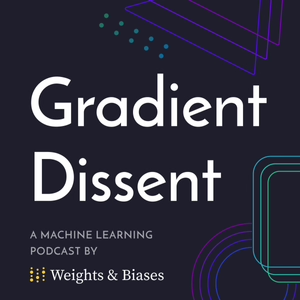
Zack Chase Lipton — The Medical Machine Learning Landscape
How Zack went from being a musician to professor, how medical applications of Machine Learning are developing, and the challenges of counteracting bias in real world applications. Zachary Chase Lipton is an assistant professor of Operations Research and Machine Learning at Carnegie Mellon University. His research spans core machine learning methods and their social impact and addresses diverse application areas, including clinical medicine and natural language processing. Current research focuses include robustness under distribution shift, breast cancer screening, the effective and equitable allocation of organs, and the intersection of causal thinking with messy data. He is the founder of the Approximately Correct (approximatelycorrect.com) blog and the creator of Dive Into Deep Learning, an interactive open-source book drafted entirely through Jupyter notebooks. Zack’s blog - http://approximatelycorrect.com/ Detecting and Correcting for Label Shift with Black Box Predictors: https://arxiv.org/pdf/1802.03916.pdf Algorithmic Fairness from a Non-Ideal Perspective https://www.datascience.columbia.edu/data-good-zachary-lipton-lecture Jonas Peter’s lectures on causality: https://youtu.be/zvrcyqcN9Wo 0:00 Sneak peek: Is this a problem worth solving? 0:38 Intro 1:23 Zack’s journey from being a musician to a professor at CMU 4:45 Applying machine learning to medical imaging 10:14 Exploring new frontiers: the most impressive deep learning applications for healthcare 12:45 Evaluating the models – Are they ready to be deployed in hospitals for use by doctors? 19:16 Capturing the signals in evolving representations of healthcare data 27:00 How does the data we capture affect the predictions we make 30:40 Distinguishing between associations and correlations in data – Horror vs romance movies 34:20 The positive effects of augmenting datasets with counterfactually flipped data 39:25 Algorithmic fairness in the real world 41:03 What does it mean to say your model isn’t biased? 43:40 Real world implications of decisions to counteract model bias 49:10 The pragmatic approach to counteracting bias in a non-ideal world 51:24 An underrated aspect of machine learning 55:11 Why defining the problem is the biggest challenge for machine learning in the real world Visit our podcasts homepage for transcripts and more episodes! www.wandb.com/podcast Get our podcast on YouTube, Apple, and Spotify! YouTube: https://www.youtube.com/c/WeightsBiases Soundcloud: https://bit.ly/2YnGjIq Apple Podcasts: https://bit.ly/2WdrUvI Spotify: https://bit.ly/2SqtadF We started Weights and Biases to build tools for Machine Learning practitioners because we care a lot about the impact that Machine Learning can have in the world and we love working in the trenches with the people building these models. One of the most fun things about these building tools has been the conversations with these ML practitioners and learning about the interesting things they’re working on. This process has been so fun that we wanted to open it up to the world in the form of our new podcast called Gradient Dissent. We hope you have as much fun listening to it as we had making it! Join our bi-weekly virtual salon and listen to industry leaders and researchers in machine learning share their research: http://tiny.cc/wb-salon Join our community of ML practitioners where we host AMA's, share interesting projects and meet other people working in Deep Learning: http://bit.ly/wandb-forum Our gallery features curated machine learning reports by researchers exploring deep learning techniques, Kagglers showcasing winning models, and industry leaders sharing best practices. https://app.wandb.ai/gallery
Next Episode
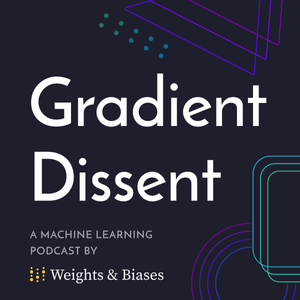
Joaquin Candela — Definitions of Fairness
Joaquin chats about scaling and democratizing AI at Facebook, while understanding fairness and algorithmic bias. --- Joaquin Quiñonero Candela is Distinguished Tech Lead for Responsible AI at Facebook, where he aims to understand and mitigate the risks and unintended consequences of the widespread use of AI across Facebook. He was previously Director of Society and AI Lab and Director of Engineering for Applied ML. Before joining Facebook, Joaquin taught at the University of Cambridge, and worked at Microsoft Research. Connect with Joaquin: Personal website: https://quinonero.net/ Twitter: https://twitter.com/jquinonero LinkedIn: https://www.linkedin.com/in/joaquin-qui%C3%B1onero-candela-440844/ --- Topics Discussed: 0:00 Intro, sneak peak 0:53 Looking back at building and scaling AI at Facebook 10:31 How do you ship a model every week? 15:36 Getting buy-in to use a system 19:36 More on ML tools 24:01 Responsible AI at Facebook 38:33 How to engage with those effected by ML decisions 41:54 Approaches to fairness 53:10 How to know things are built right 59:34 Diversity, inclusion, and AI 1:14:21 Underrated aspect of AI 1:16:43 Hardest thing when putting models into production Transcript: http://wandb.me/gd-joaquin-candela Links Discussed: Race and Gender (2019): https://arxiv.org/pdf/1908.06165.pdf Lessons from Archives: Strategies for Collecting Sociocultural Data in Machine Learning (2019): https://arxiv.org/abs/1912.10389 Gender Shades: Intersectional Accuracy Disparities in Commercial Gender Classification (2018): http://proceedings.mlr.press/v81/buolamwini18a.html --- Get our podcast on these platforms: Apple Podcasts: http://wandb.me/apple-podcasts Spotify: http://wandb.me/spotify Google Podcasts: http://wandb.me/google-podcasts YouTube: http://wandb.me/youtube Soundcloud: http://wandb.me/soundcloud Join our community of ML practitioners where we host AMAs, share interesting projects and meet other people working in Deep Learning: http://wandb.me/slack Check out Fully Connected, which features curated machine learning reports by researchers exploring deep learning techniques, Kagglers showcasing winning models, industry leaders sharing best practices, and more: https://wandb.ai/fully-connected
If you like this episode you’ll love
Episode Comments
Generate a badge
Get a badge for your website that links back to this episode
<a href="https://goodpods.com/podcasts/gradient-dissent-conversations-on-ai-163784/richard-socher-the-challenges-of-making-ml-work-in-the-real-world-8937468"> <img src="https://storage.googleapis.com/goodpods-images-bucket/badges/generic-badge-1.svg" alt="listen to richard socher — the challenges of making ml work in the real world on goodpods" style="width: 225px" /> </a>
Copy