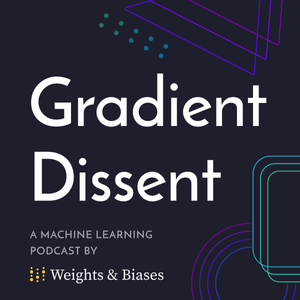
Joaquin Candela — Definitions of Fairness
10/01/20 • 79 min
Previous Episode
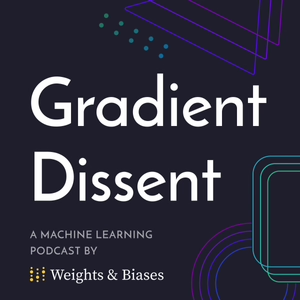
Richard Socher — The Challenges of Making ML Work in the Real World
Richard Socher, ex-Chief Scientist at Salesforce, joins us to talk about The AI Economist, NLP protein generation and biggest challenge in making ML work in the real world. Richard Socher was the Chief scientist (EVP) at Salesforce where he lead teams working on fundamental research(einstein.ai/), applied research, product incubation, CRM search, customer service automation and a cross-product AI platform for unstructured and structured data. Previously, he was an adjunct professor at Stanford’s computer science department and the founder and CEO/CTO of MetaMind(www.metamind.io/) which was acquired by Salesforce in 2016. In 2014, he got my PhD in the [CS Department](www.cs.stanford.edu/) at Stanford. He likes paramotoring and water adventures, traveling and photography. More info: - Forbes article: https://www.forbes.com/sites/gilpress/2017/05/01/emerging-artificial-intelligence-ai-leaders-richard-socher-salesforce/) with more info about Richard's bio. - CS224n - NLP with Deep Learning(http://cs224n.stanford.edu/) the class Richard used to teach. - TEDx talk(https://www.youtube.com/watch?v=8cmx7V4oIR8) about where AI is today and where it's going. Research: Google Scholar Link(https://scholar.google.com/citations?user=FaOcyfMAAAAJ&hl=en) The AI Economist: Improving Equality and Productivity with AI-Driven Tax Policies Arxiv link(https://arxiv.org/abs/2004.13332), blog(https://blog.einstein.ai/the-ai-economist/), short video(https://www.youtube.com/watch?v=4iQUcGyQhdA), Q&A(https://salesforce.com/company/news-press/stories/2020/4/salesforce-ai-economist/), Press: VentureBeat(https://venturebeat.com/2020/04/29/salesforces-ai-economist-taps-reinforcement-learning-to-generate-optimal-tax-policies/), TechCrunch(https://techcrunch.com/2020/04/29/salesforce-researchers-are-working-on-an-ai-economist-for-more-equitable-tax-policy/) ProGen: Language Modeling for Protein Generation: bioRxiv link(https://www.biorxiv.org/content/10.1101/2020.03.07.982272v2), [blog](https://blog.einstein.ai/progen/) ] Dye-sensitized solar cells under ambient light powering machine learning: towards autonomous smart sensors for the internet of things Issue11, (**Chemical Science 2020**). paper link(https://pubs.rsc.org/en/content/articlelanding/2020/sc/c9sc06145b#!divAbstract) CTRL: A Conditional Transformer Language Model for Controllable Generation: Arxiv link(https://arxiv.org/abs/1909.05858), code pre-trained and fine-tuning(https://github.com/salesforce/ctrl), blog(https://blog.einstein.ai/introducing-a-conditional-transformer-language-model-for-controllable-generation/) Genie: a generator of natural language semantic parsers for virtual assistant commands: PLDI 2019 pdf link(https://almond-static.stanford.edu/papers/genie-pldi19.pdf), https://almond.stanford.edu Topics Covered: 0:00 intro 0:42 the AI economist 7:08 the objective function and Gini Coefficient 12:13 on growing up in Eastern Germany and cultural differences 15:02 Language models for protein generation (ProGen) 27:53 CTRL: conditional transformer language model for controllable generation 37:52 Businesses vs Academia 40:00 What ML applications are important to salesforce 44:57 an underrated aspect of machine learning 48:13 Biggest challenge in making ML work in the real world Visit our podcasts homepage for transcripts and more episodes! www.wandb.com/podcast Get our podcast on Soundcloud, Apple, Spotify, and Google! Soundcloud: https://bit.ly/2YnGjIq Apple Podcasts: https://bit.ly/2WdrUvI Spotify: https://bit.ly/2SqtadF Google: http://tiny.cc/GD_Google Weights and Biases makes developer tools for deep learning. Join our bi-weekly virtual salon and listen to industry leaders and researchers in machine learning share their research: http://tiny.cc/wb-salon Join our community of ML practitioners: http://bit.ly/wb-slack Our gallery features curated machine learning reports by ML researchers. https://app.wandb.ai/gallery
Next Episode
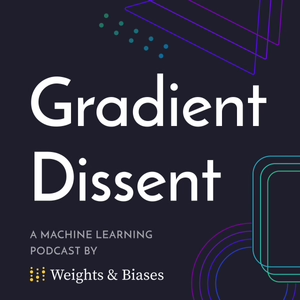
Daeil Kim — The Unreasonable Effectiveness of Synthetic Data
Supercharging computer vision model performance by generating years of training data in minutes. Daeil Kim is the co-founder and CEO of AI.Reverie(https://aireverie.com/), a startup that specializes in creating high quality synthetic training data for computer vision algorithms. Before that, he was a senior data scientist at the New York Times. And before that he got his PhD in computer science from Brown University, focusing on machine learning and Bayesian statistics. He's going to talk about tools that will advance machine learning progress, and he's going to talk about synthetic data. https://twitter.com/daeil Topics covered: 0:00 Diversifying content 0:23 Intro+bio 1:00 From liberal arts to synthetic data 8:48 What is synthetic data? 11:24 Real world examples of synthetic data 16:16 Understanding performance gains using synthetic data 21:32 The future of Synthetic data and AI.Reverie 23:21 The composition of people at AI.reverie and ML 28:28 The evolution of ML tools and systems that Daeil uses 33:16 Most underrated aspect of ML and common misconceptions 34:42 Biggest challenge in making synthetic data work in the real world Visit our podcasts homepage for transcripts and more episodes! www.wandb.com/podcast Get our podcast on Apple, Spotify, and Google! Apple Podcasts: bit.ly/2WdrUvI Spotify: bit.ly/2SqtadF Google:tiny.cc/GD_Google We started Weights and Biases to build tools for Machine Learning practitioners because we care a lot about the impact that Machine Learning can have in the world and we love working in the trenches with the people building these models. One of the most fun things about these building tools has been the conversations with these ML practitioners and learning about the interesting things they’re working on. This process has been so fun that we wanted to open it up to the world in the form of our new podcast called Gradient Dissent. We hope you have as much fun listening to it as we had making it! Join our bi-weekly virtual salon and listen to industry leaders and researchers in machine learning share their research: tiny.cc/wb-salon Join our community of ML practitioners where we host AMA's, share interesting projects and meet other people working in Deep Learning: bit.ly/wb-slack Our gallery features curated machine learning reports by researchers exploring deep learning techniques, Kagglers showcasing winning models, and industry leaders sharing best practices. app.wandb.ai/gallery
If you like this episode you’ll love
Episode Comments
Generate a badge
Get a badge for your website that links back to this episode
<a href="https://goodpods.com/podcasts/gradient-dissent-conversations-on-ai-163784/joaquin-candela-definitions-of-fairness-8937467"> <img src="https://storage.googleapis.com/goodpods-images-bucket/badges/generic-badge-1.svg" alt="listen to joaquin candela — definitions of fairness on goodpods" style="width: 225px" /> </a>
Copy