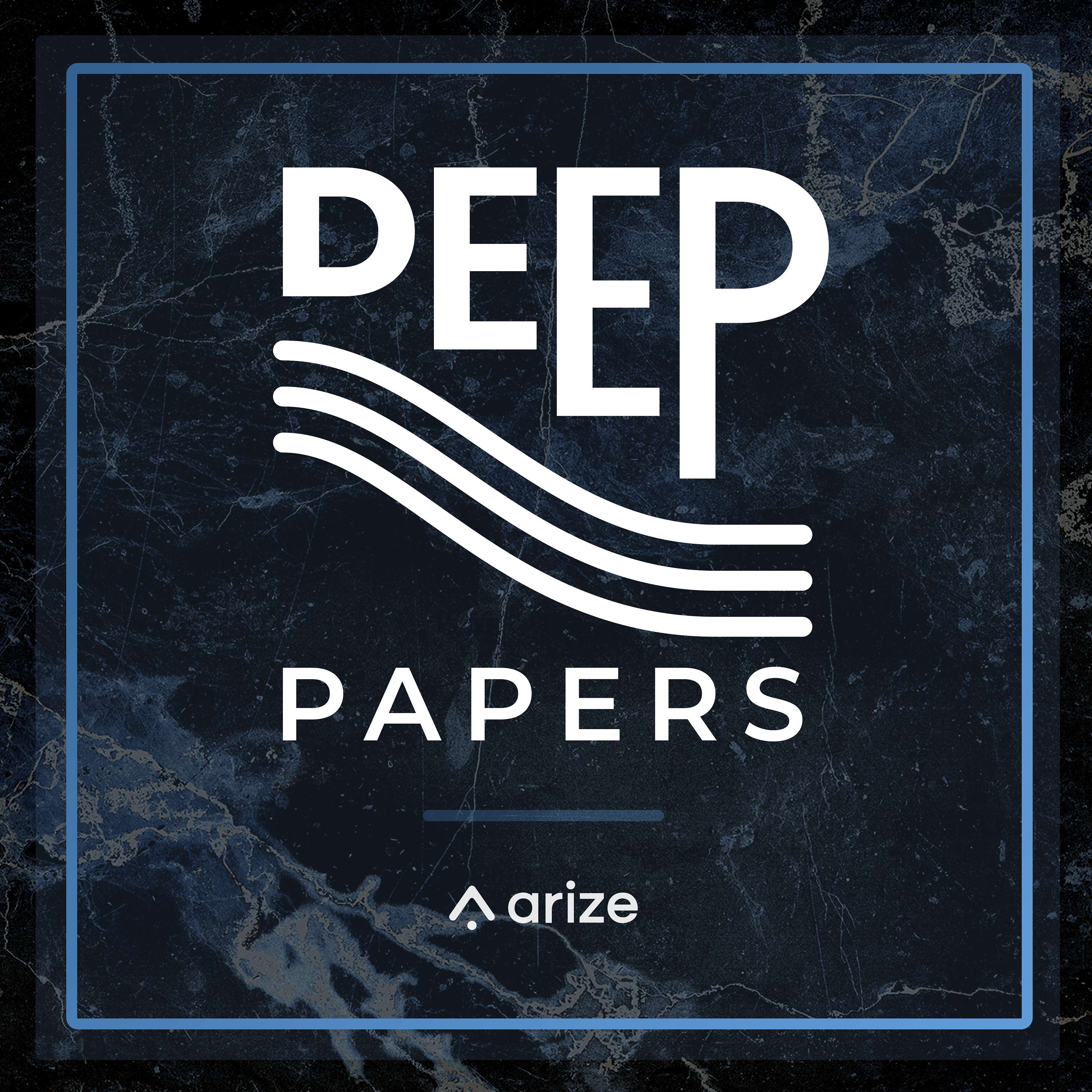
RAG vs Fine-Tuning
02/08/24 • 39 min
This week, we’re discussing "RAG vs Fine-Tuning: Pipelines, Tradeoff, and a Case Study on Agriculture." This paper explores a pipeline for fine-tuning and RAG, and presents the tradeoffs of both for multiple popular LLMs, including Llama2-13B, GPT-3.5, and GPT-4.
The authors propose a pipeline that consists of multiple stages, including extracting information from PDFs, generating questions and answers, using them for fine-tuning, and leveraging GPT-4 for evaluating the results. Overall, the results point to how systems built using LLMs can be adapted to respond and incorporate knowledge across a dimension that is critical for a specific industry, paving the way for further applications of LLMs in other industrial domains.
Learn more about AI observability and evaluation, join the Arize AI Slack community or get the latest on LinkedIn and X.
This week, we’re discussing "RAG vs Fine-Tuning: Pipelines, Tradeoff, and a Case Study on Agriculture." This paper explores a pipeline for fine-tuning and RAG, and presents the tradeoffs of both for multiple popular LLMs, including Llama2-13B, GPT-3.5, and GPT-4.
The authors propose a pipeline that consists of multiple stages, including extracting information from PDFs, generating questions and answers, using them for fine-tuning, and leveraging GPT-4 for evaluating the results. Overall, the results point to how systems built using LLMs can be adapted to respond and incorporate knowledge across a dimension that is critical for a specific industry, paving the way for further applications of LLMs in other industrial domains.
Learn more about AI observability and evaluation, join the Arize AI Slack community or get the latest on LinkedIn and X.
Previous Episode
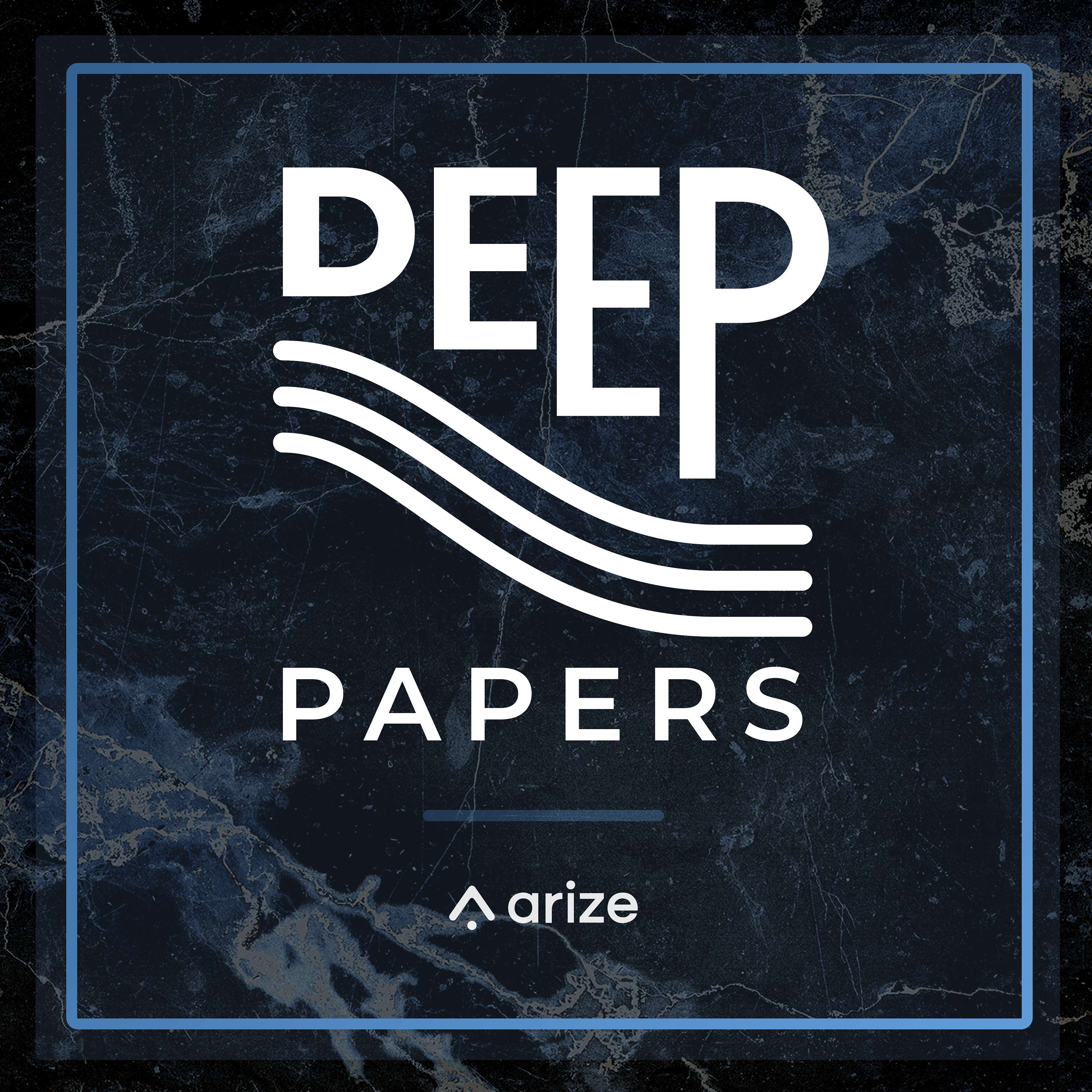
HyDE: Precise Zero-Shot Dense Retrieval without Relevance Labels
We discuss HyDE: a thrilling zero-shot learning technique that combines GPT-3’s language understanding with contrastive text encoders.
HyDE revolutionizes information retrieval and grounding in real-world data by generating hypothetical documents from queries and retrieving similar real-world documents. It outperforms traditional unsupervised retrievers, rivaling fine-tuned retrievers across diverse tasks and languages. This leap in zero-shot learning efficiently retrieves relevant real-world information without task-specific fine-tuning, broadening AI model applicability and effectiveness.
Link to transcript and live recording: https://arize.com/blog/hyde-paper-reading-and-discussion/
Learn more about AI observability and evaluation, join the Arize AI Slack community or get the latest on LinkedIn and X.
Next Episode
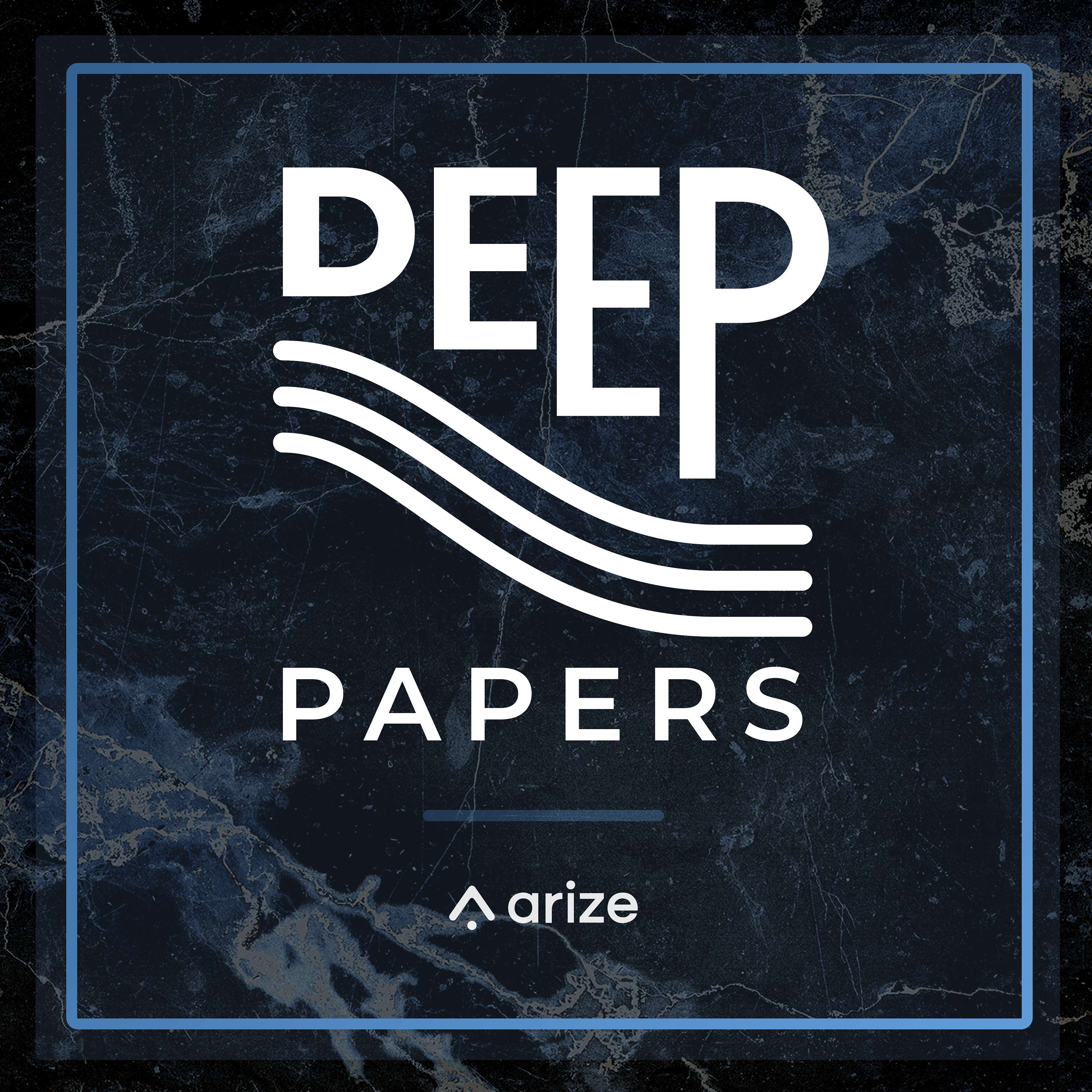
Sora: OpenAI’s Text-to-Video Generation Model
This week, we discuss the implications of Text-to-Video Generation and speculate as to the possibilities (and limitations) of this incredible technology with some hot takes. Dat Ngo, ML Solutions Engineer at Arize, is joined by community member and AI Engineer Vibhu Sapra to review OpenAI’s technical report on their Text-To-Video Generation Model: Sora.
According to OpenAI, “Sora can generate videos up to a minute long while maintaining visual quality and adherence to the user’s prompt.” At the time of this recording, the model had not been widely released yet, but was becoming available to red teamers to assess risk, and also to artists to receive feedback on how Sora could be helpful for creatives.
At the end of our discussion, we also explore EvalCrafter: Benchmarking and Evaluating Large Video Generation Models. This recent paper proposed a new framework and pipeline to exhaustively evaluate the performance of the generated videos, which we look at in light of Sora.
Learn more about AI observability and evaluation, join the Arize AI Slack community or get the latest on LinkedIn and X.
If you like this episode you’ll love
Episode Comments
Generate a badge
Get a badge for your website that links back to this episode
<a href="https://goodpods.com/podcasts/deep-papers-251735/rag-vs-fine-tuning-44502361"> <img src="https://storage.googleapis.com/goodpods-images-bucket/badges/generic-badge-1.svg" alt="listen to rag vs fine-tuning on goodpods" style="width: 225px" /> </a>
Copy