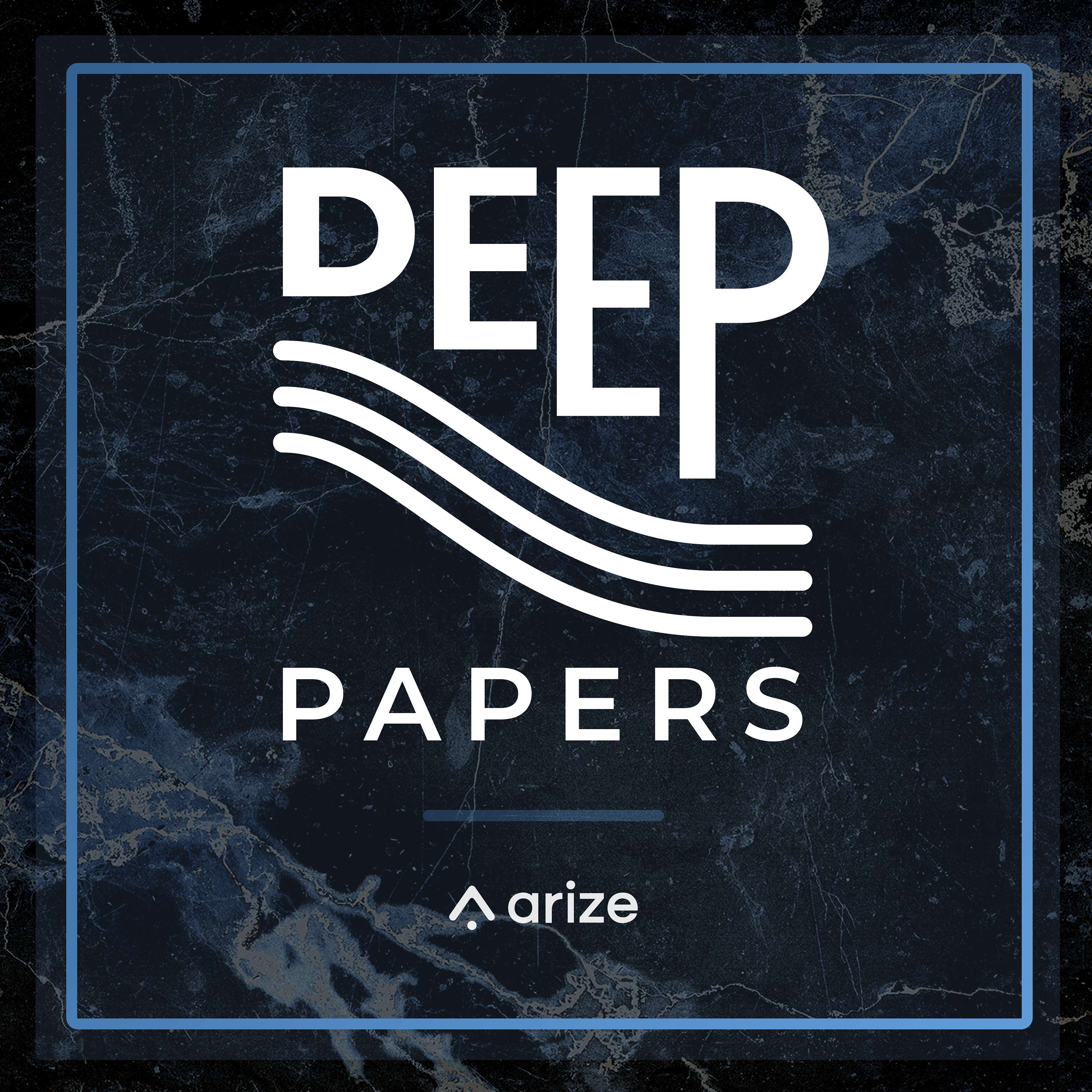
AI Benchmark Deep Dive: Gemini 2.5 and Humanity's Last Exam
04/04/25 • 26 min
1 Listener
This week we talk about modern AI benchmarks, taking a close look at Google's recent Gemini 2.5 release and its performance on key evaluations, notably Humanity's Last Exam (HLE). In the session we covered Gemini 2.5's architecture, its advancements in reasoning and multimodality, and its impressive context window. We also talked about how benchmarks like HLE and ARC AGI 2 help us understand the current state and future direction of AI.
Read it on the blog: https://arize.com/blog/ai-benchmark-deep-dive-gemini-humanitys-last-exam/
Sign up to watch the next live recording: https://arize.com/resource/community-papers-reading/
Learn more about AI observability and evaluation, join the Arize AI Slack community or get the latest on LinkedIn and X.
This week we talk about modern AI benchmarks, taking a close look at Google's recent Gemini 2.5 release and its performance on key evaluations, notably Humanity's Last Exam (HLE). In the session we covered Gemini 2.5's architecture, its advancements in reasoning and multimodality, and its impressive context window. We also talked about how benchmarks like HLE and ARC AGI 2 help us understand the current state and future direction of AI.
Read it on the blog: https://arize.com/blog/ai-benchmark-deep-dive-gemini-humanitys-last-exam/
Sign up to watch the next live recording: https://arize.com/resource/community-papers-reading/
Learn more about AI observability and evaluation, join the Arize AI Slack community or get the latest on LinkedIn and X.
Previous Episode
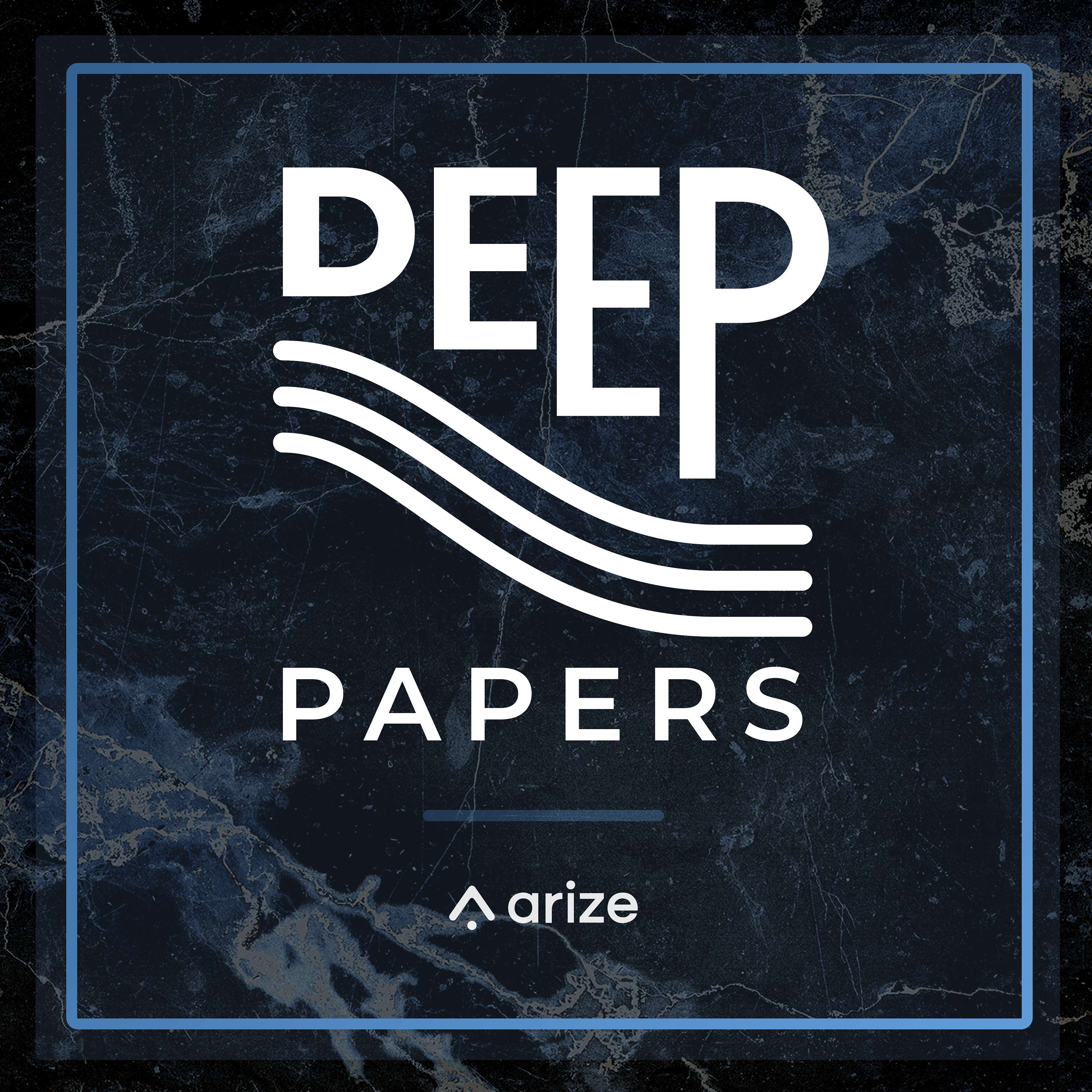
Model Context Protocol (MCP)
We cover Anthropic’s groundbreaking Model Context Protocol (MCP). Though it was released in November 2024, we've been seeing a lot of hype around it lately, and thought it was well worth digging into.
Learn how this open standard is revolutionizing AI by enabling seamless integration between LLMs and external data sources, fundamentally transforming them into capable, context-aware agents. We explore the key benefits of MCP, including enhanced context retention across interactions, improved interoperability for agentic workflows, and the development of more capable AI agents that can execute complex tasks in real-world environments.
Learn more about AI observability and evaluation, join the Arize AI Slack community or get the latest on LinkedIn and X.
Next Episode
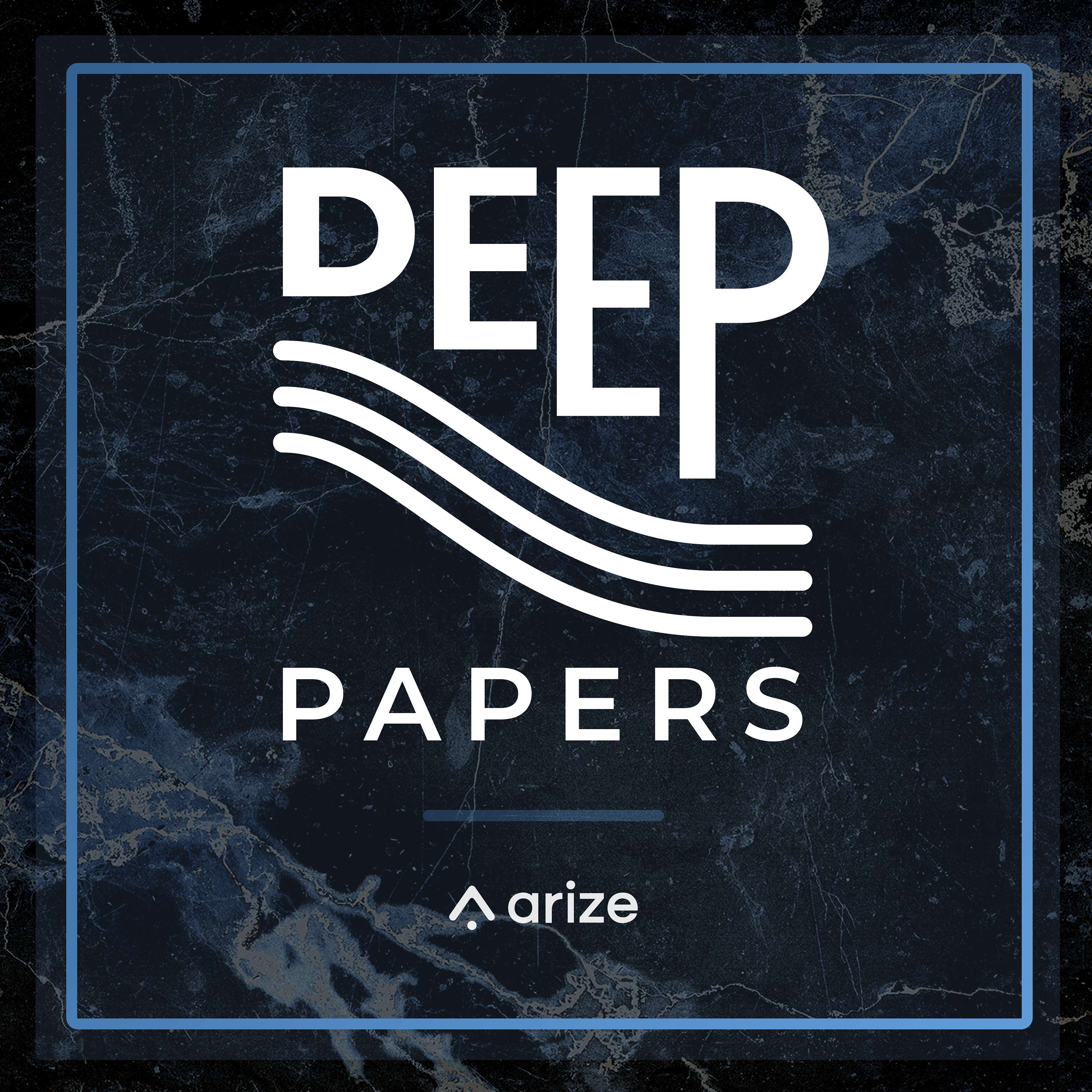
LibreEval: The Largest Open Source Benchmark for RAG Hallucination Detection
For this week's paper read, we actually dive into our own research.
We wanted to create a replicable, evolving dataset that can keep pace with model training so that you always know you're testing with data your model has never seen before. We also saw the prohibitively high cost of running LLM evals at scale, and have used our data to fine-tune a series of SLMs that perform just as well as their base LLM counterparts, but at 1/10 the cost.
So, over the past few weeks, the Arize team generated the largest public dataset of hallucinations, as well as a series of fine-tuned evaluation models.
We talk about what we built, the process we took, and the bottom line results.
📃 Read the paper: https://arize.com/llm-hallucination-dataset/
Learn more about AI observability and evaluation, join the Arize AI Slack community or get the latest on LinkedIn and X.
If you like this episode you’ll love
Episode Comments
Generate a badge
Get a badge for your website that links back to this episode
<a href="https://goodpods.com/podcasts/deep-papers-251735/ai-benchmark-deep-dive-gemini-25-and-humanitys-last-exam-88741465"> <img src="https://storage.googleapis.com/goodpods-images-bucket/badges/generic-badge-1.svg" alt="listen to ai benchmark deep dive: gemini 2.5 and humanity's last exam on goodpods" style="width: 225px" /> </a>
Copy