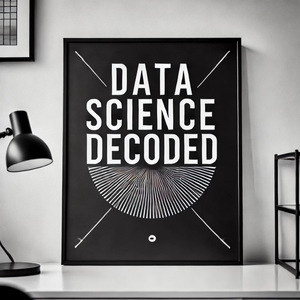
Data Science #7 - "The use of multiple measurements in taxonomic problems." (1936), Fisher RA
08/12/24 • 47 min
This paper introduced linear discriminant analysis(LDA), a statistical technique that revolutionized classification in biology and beyond.
Fisher demonstrated how to use multiple measurements to distinguish between different species of iris flowers, laying the foundation for modern multivariate statistics.
His work showed that combining several characteristics could provide more accurate classification than relying on any single trait.
This paper not only solved a practical problem in botany but also opened up new avenues for statistical analysis across various fields.
Fisher's method became a cornerstone of pattern recognition and machine learning, influencing diverse areas from medical diagnostics to AI.
The iris dataset he used, now known as the "Fisher iris" or "Anderson iris" dataset, remains a popular example in data science education and research.
This paper introduced linear discriminant analysis(LDA), a statistical technique that revolutionized classification in biology and beyond.
Fisher demonstrated how to use multiple measurements to distinguish between different species of iris flowers, laying the foundation for modern multivariate statistics.
His work showed that combining several characteristics could provide more accurate classification than relying on any single trait.
This paper not only solved a practical problem in botany but also opened up new avenues for statistical analysis across various fields.
Fisher's method became a cornerstone of pattern recognition and machine learning, influencing diverse areas from medical diagnostics to AI.
The iris dataset he used, now known as the "Fisher iris" or "Anderson iris" dataset, remains a popular example in data science education and research.
Previous Episode
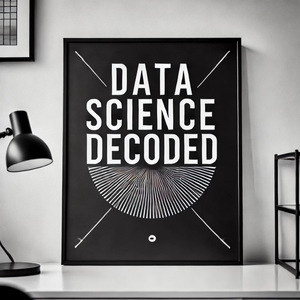
Data Science #6 -"On the problem of the most efficient tests of statistical hypotheses." (1933) N&P
This paper is considered one of the foundational works in modern statistical hypothesis testing.
Key insights and influences:
- Neyman-Pearson Lemma: The paper introduced the Neyman-Pearson Lemma, which provides a method for finding the most powerful test for a simple hypothesis against a simple alternative.
- Type I and Type II errors: It formalized the concepts of Type I (false positive) and Type II (false negative) errors in hypothesis testing.
- Power of a test: The paper introduced the concept of the power of a statistical test, which is the probability of correctly rejecting a false null hypothesis.
- Likelihood ratio tests: It laid the groundwork for likelihood ratio tests, which are widely used in modern statistics.
- Optimal testing: The paper provided a framework for finding optimal statistical tests, balancing the tradeoff between Type I and Type II errors.
These concepts have had a profound influence on modern statistical theory and practice, forming the basis of much of classical hypothesis testing used today in various fields of science and research.
Next Episode
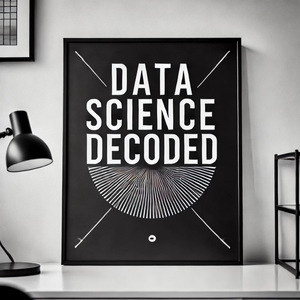
Data Science #8 - The Turing test by Turing Alan "Computing machinery and intelligence" Mind (1950)
This paper is a foundational text in the field of artificial intelligence (AI) and explores the question: "Can machines think?" Turing introduces what is now known as the "Turing Test" as a way to operationalize this question, he called it the imitation game. Are there imaginable digital computers that could perform well in the imitation game? The imitation game involves an interrogator trying to distinguish between a human and a machine based on their responses to various questions. Turing argues that if a machine could perform well enough in this game to be indistinguishable from a human, then it could be said to "think." He explores various objections to the idea that machines can think, including theological, mathematical, and arguments from consciousness.
Turing addresses each objection, ultimately suggesting that machines can indeed be said to think if they can perform human-like tasks, especially those that involve reasoning, learning, and language.
If you like this episode you’ll love
Episode Comments
Generate a badge
Get a badge for your website that links back to this episode
<a href="https://goodpods.com/podcasts/data-science-decoded-556870/data-science-7-the-use-of-multiple-measurements-in-taxonomic-problems-71086113"> <img src="https://storage.googleapis.com/goodpods-images-bucket/badges/generic-badge-1.svg" alt="listen to data science #7 - "the use of multiple measurements in taxonomic problems." (1936), fisher ra on goodpods" style="width: 225px" /> </a>
Copy