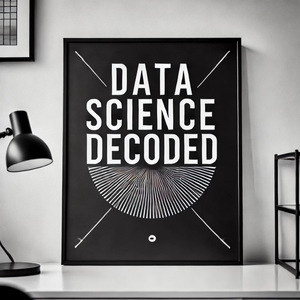
Data Science #22 - The theory of dynamic programming, Paper review 1954
01/07/25 • 47 min
Previous Episode
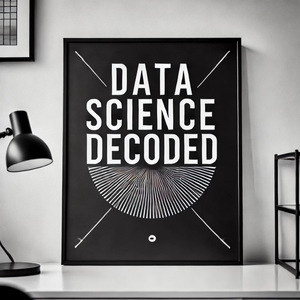
Data Science #21 - Steps Toward Artificial Intelligence
In the 1st episode of the second season we review the legendary Marvin Minsky's "Steps Toward Artificial Intelligence" from 1961. Itis a foundational work in the field of AI that outlines the challenges and methodologies for developing intelligent problem-solving systems. The paper categorizes AI challenges into five key areas: Search, Pattern Recognition, Learning, Planning, and Induction. It emphasizes how computers, limited by their ability to perform only programmed actions, can enhance problem-solving efficiency through heuristic methods, learning from patterns, and planning solutions to narrow down possible options. The significance of this work lies in its conceptual framework, which established a systematic approach to AI development. Minsky highlighted the need for machines to mimic cognitive functions like recognizing patterns and learning from experience, which form the basis of modern machine learning algorithms. His emphasis on heuristic methods provided a pathway to make computational processes more efficient and adaptive by reducing exhaustive searches and using past data to refine problem-solving strategies. The paper is pivotal as it set the stage for advancements in AI by introducing the integration of planning, adaptive learning, and pattern recognition into computational systems. Minsky's insights continue to influence AI research and development, including neural networks, reinforcement learning, and autonomous systems, bridging theoretical exploration and practical applications in the quest for artificial intelligence.
Next Episode
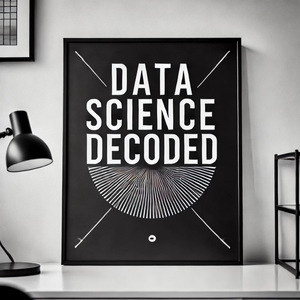
Data Science #23- The Markov Chain Monte Carl MCMC Paper review (1953)
In the 23rd episode we review the The 1953 paper Metropolis, Nicholas, et al. "Equation of state calculations by fast computing machines."
The journal of chemical physics 21.6 (1953): 1087-1092 which introduced the Monte Carlo method for simulating molecular systems, particularly focusing on two-dimensional rigid-sphere models.
The study used random sampling to compute equilibrium properties like pressure and density, demonstrating a feasible approach for solving analytically intractable statistical mechanics problems. The work pioneered the Metropolis algorithm, a key development in what later became known as Markov Chain Monte Carlo (MCMC) methods.
By validating the Monte Carlo technique against free volume theories and virial expansions, the study showcased its accuracy and set the stage for MCMC as a powerful tool for exploring complex probability distributions. This breakthrough has had a profound impact on modern AI and ML, where MCMC methods are now central to probabilistic modeling, Bayesian inference, and optimization.
These techniques enable applications like generative models, reinforcement learning, and neural network training, supporting the development of robust, data-driven AI systems.
Youtube: https://www.youtube.com/watch?v=gWOawt7hc88&t
If you like this episode you’ll love
Episode Comments
Generate a badge
Get a badge for your website that links back to this episode
<a href="https://goodpods.com/podcasts/data-science-decoded-556870/data-science-22-the-theory-of-dynamic-programming-paper-review-1954-81444169"> <img src="https://storage.googleapis.com/goodpods-images-bucket/badges/generic-badge-1.svg" alt="listen to data science #22 - the theory of dynamic programming, paper review 1954 on goodpods" style="width: 225px" /> </a>
Copy