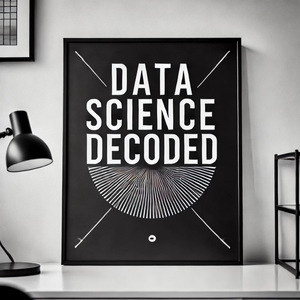
We review the original Monte Carlo paper from 1949 by Metropolis, Nicholas, and Stanislaw Ulam. "The monte carlo method." Journal of the American statistical association 44.247 (1949): 335-341. The Monte Carlo method uses random sampling to approximate solutions for problems that are too complex for analytical methods, such as integration, optimization, and simulation. Its power lies in leveraging randomness to solve high-dimensional and nonlinear problems, making it a fundamental tool in computational science. In modern data science and AI, Monte Carlo drives key techniques like Bayesian inference (via MCMC) for probabilistic modeling, reinforcement learning for policy evaluation, and uncertainty quantification in predictions. It is essential for handling intractable computations in machine learning and AI systems. By combining scalability and flexibility, Monte Carlo methods enable breakthroughs in areas like natural language processing, computer vision, and autonomous systems. Its ability to approximate solutions underpins advancements in probabilistic reasoning, decision-making, and optimization in the era of AI and big data.
11/18/24 • 38 min
Generate a badge
Get a badge for your website that links back to this episode
Select type & size
<a href="https://goodpods.com/podcasts/data-science-decoded-556870/data-science-17-the-monte-carlo-algorithm-1949-78508520"> <img src="https://storage.googleapis.com/goodpods-images-bucket/badges/generic-badge-1.svg" alt="listen to data science #17 - the monte carlo algorithm (1949) on goodpods" style="width: 225px" /> </a>
Copy