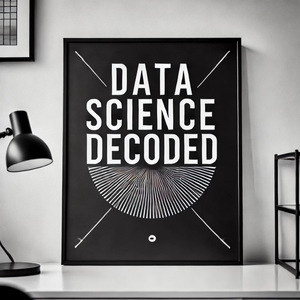
Data Science #10 - The original principal component analysis (PCA) paper by Harold Hotelling (1935)
Data Science Decoded09/12/24 • 55 min
Hotelling, Harold. "Analysis of a complex of statistical variables into principal components." Journal of educational psychology 24.6 (1933): 417.
This seminal work by Harold Hotelling on PCA remains highly relevant to modern data science because PCA is still widely used for dimensionality reduction, feature extraction, and data visualization. The foundational concepts of eigenvalue decomposition and maximizing variance in orthogonal directions form the backbone of PCA, which is now automated through numerical methods such as Singular Value Decomposition (SVD). Modern PCA handles much larger datasets with advanced variants (e.g., Kernel PCA, Sparse PCA), but the core ideas from the paper—identifying and interpreting key components to reduce dimensionality while preserving the most important information—are still crucial in handling high-dimensional data efficiently today.
09/12/24 • 55 min
Generate a badge
Get a badge for your website that links back to this episode
<a href="https://goodpods.com/podcasts/data-science-decoded-556870/data-science-10-the-original-principal-component-analysis-pca-paper-by-73786762"> <img src="https://storage.googleapis.com/goodpods-images-bucket/badges/generic-badge-1.svg" alt="listen to data science #10 - the original principal component analysis (pca) paper by harold hotelling (1935) on goodpods" style="width: 225px" /> </a>
Copy