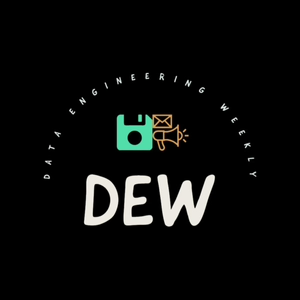
Hey folks, have you heard about the Data Council conference in Austin? The three-day event was jam-packed with exciting discussions and innovative ideas on data engineering and infrastructure, data science and algorithms, MLOps, generative AI, streaming infrastructure, analytics, and data culture and community.
"People are so nice in the data community. Meeting them and brainstorming with many ideas and various thought processes is amazing. It was an amazing experience; The conference is mostly like a jam of different thought processes, ideas, and entrepreneurship.
The keynote by Shrishanka from AcrylData talked about how data catalogs are becoming the control center for pipelines, a game-changer for the industry.
I also had a chance to attend a session on Malloy, a new way of thinking about SQL queries. It was experimental but had some cool ideas on abstracting complicated SQL queries. ChatGPT will change the game in terms of data engineering jobs and productivity. Charge GPT, for example, has improved my productivity by 60%. And generative AI is becoming so advanced that it can produce dynamic SQL code in just a few lines.
But of course, with all this innovation and change, there are still questions about the future. Will Snowflake and Databricks outsource data governance experience to other companies? Will the modern data stack become more mature and consolidated? These are the big questions we need to ask as we move forward in the world of data.
The talk by Uber on their Ubermetric system migrating from ElasticSearch to Apache Pinot - which, by the way, is an incredibly flexible and powerful system. We also chatted about Pinot's semi-structured storage support, which is important in modern data engineering.
Now, let's talk about something (non)controversial: the idea that big data is dead. DuckDB brought up three intriguing points to back up this claim.
- Not every company has Big Data.
- The availability of instances with higher memory is becoming a commodity
- Even with the companies have big data; they do only incremental processing, which can be small enough
Abhi Sivasailam presented a thought-provoking approach to metric standardization. He introduced the concept of "metric trees" - connecting high-level metrics to other metrics and building semantics around them. The best part? You can create a whole tree structure that shows the impact of one metric on another. Imagine the possibilities! You could simulate your business performance by tweaking the metric tree, which is mind-blowing!
Another amazing talk was about cross-company data exchange, where Pardis discussed various ways companies share data, like APIs, file uploads, or even Snowflake sharing. But the real question is: How do we deal with revenue sharing, data governance, and preventing sensitive data leaks? Pardis's startup General Folders, is tackling this issue, becoming the "Dropbox" of data exchange. How cool is that?
To wrap it up, three key learnings from the conference were:
- The intriguing idea is that "big data is dead" and how it impacts data infrastructure architecture.
- Data Catalog as a control plane for modern data stack? Is it a dream or reality?
- The growing importance of data contracts and the fascinating idea of metric trees.
Overall, the Data Council conference was an incredible experience, and I can't wait to see what they have in store for us next year.
04/06/23 • 36 min
Generate a badge
Get a badge for your website that links back to this episode
<a href="https://goodpods.com/podcasts/data-engineering-weekly-249164/what-happened-at-data-council-2023-29243985"> <img src="https://storage.googleapis.com/goodpods-images-bucket/badges/generic-badge-1.svg" alt="listen to what happened at data council 2023? on goodpods" style="width: 225px" /> </a>
Copy