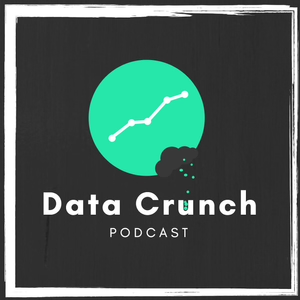
Getting into Data Science
03/01/19 • 22 min
Previous Episode
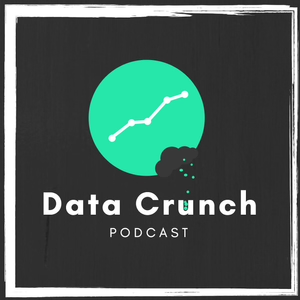
Automated Machine Learning with TransmogrifAI
Would you rather take a year to develop a proprietary algorithm for your company that has an accuracy of 95% or use an open source platform that takes a day to develop an algorithm that has nearly the same accuracy? In most business cases, you'd choose the latter. In this episode, we talk to Till Bergmann who works on a team that developed TransmogriAI, an open source project that helps you build models quickly.
Next Episode
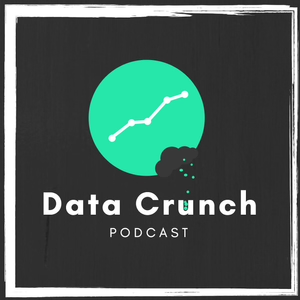
Statistics Done Wrong—A Woeful Podcast Episode
Beginning: Statistics are misused and abused, sometimes even unintentionally, in both scientific and business settings. Alex Reinhart, author of the book "Statistics Done Wrong: The Woefully Complete Guide" talks about the most common errors people make when trying to figure things out using statistics, and what happens as a result. He shares practical insights into how both scientists and business analysts can make sure their statistical tests have high enough power, how they can avoid “truth inflation,” and how to overcome multiple comparisons problems.Ginette: In 2009, neuroscientist Craig Bennett undertook a landmark experiment in a Dartmouth lab. A high tech fMRI machine was used on test subjects, who were “shown a series of photographs depicting human individuals in social situations with a specified emotional valence” and asked “to determine what emotion the individual in the photo must have been experiencing.” Would it be found that different parts of the brain were associated with different emotional associations? In fact, it was. The experiment was a success. The results came in showing brain activity changes for the different tasks, and the p-value came out to 0.001, indicating a significant result.The problem? The only participant was a 3.8 pound 18-inch mature Atlantic salmon, who was “not alive at the time of scanning.”Ginette: I’m Ginette.Curtis: And I’m Curtis.Ginette: And you are listening to Data Crunch.Curtis: A podcast about how applied data science, machine learning, and artificial intelligence are changing the world.Ginette: Data Crunch is produced by the Data Crunch Corporation, an analytics training and consulting company.Ginette: This study was real. It was real data, robust analysis, and an actual dead fish. It even has an official sounding scientific study name—”Neural correlates of interspecies perspective taking in the post-mortem Atlantic Salmon”.Craig Bennett did the experiment to show that statistics can be dangerous territory. They can be abused and misleading—whether or not the experimenter has nefarious intentions. Still, statistics are a legitimate and powerful tool to discover actual truths and find important insights, so they cannot be ignored. It becomes our task to wield them correctly, and to be careful when accepting or rejecting statistical assertions we come across.Today we talk to Alex Reinhart, author of the book “Statistics done wrong—The Woefully complete guide”. Alex is an expert on how to do statistics wrong. And incidentally, how to do them right.Alex: We end up using statistical methods in science and in business to answer questions, often very simple questions, of just “does this intervention or this treatment or this change that I made, does it have an effect?” Often in a difficult situation, because there are many things going on, you know, if you're doing a medical treatment there’s many different reasons that people recover in different times, and there's a lot of variation, and it’s hard to predict these things. If you’re doing an A-B test on a website, your visitors are all different. Some of them will want to buy your product or whatever it is, and some of them won’t, and so there’s a lot of variation that happens naturally, and we’re always in the position of having to ask, “This thing/change I made or invention I did, does it have an effect, and can I distinguish that effect from all the other things that are going on.” And this leads to a lot of problems, so statistical methods exist to help you answer that questions by seeing how much variation is there naturally, and this effect I saw, is it more than I would have expected had my intervention not worked or not done anything, but it doesn’t give you certainty. It gives us nice words, which is like “statistically significant,” which sounds important, but it doesn't give you certainty. You're often asking the question, “Is this effect that I’m seeing from my experim...
If you like this episode you’ll love
Episode Comments
Generate a badge
Get a badge for your website that links back to this episode
<a href="https://goodpods.com/podcasts/data-crunch-98898/getting-into-data-science-5229733"> <img src="https://storage.googleapis.com/goodpods-images-bucket/badges/generic-badge-1.svg" alt="listen to getting into data science on goodpods" style="width: 225px" /> </a>
Copy