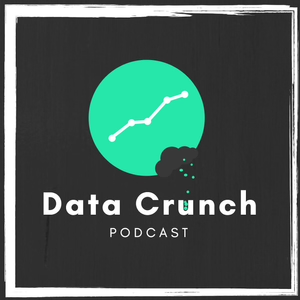
Deep Learning, Microwaves, and Bugs
11/08/19 • 18 min
Previous Episode
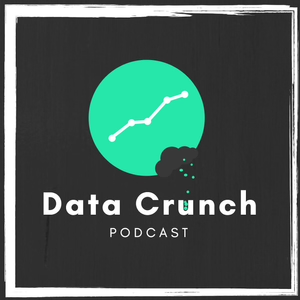
Potential Advantages of Blockchain for Data Scientists
Luciano Pesci is bullish on blockchain and data science. Since blockchain offers a complete historical record, no one can delete or alter prior information written into the record. He sees this characteristic as a massive advantage for data scientists. Luciano Pesci: And the key for data scientists and leaders who are gonna oversee data sciences, you've got to get a narrow enough problem to demonstrate one quick win and I mean in 90 days. If in 90 days you can't come back to the organization and show, "we have made real progress on these metrics in your understanding so that you can make these decisions," they're not going to continue to do it. Ginette Methot: I’m Ginette, Curtis Seare: and I’m Curtis, Ginette: and you are listening to Data Crunch, Curtis: a podcast about how applied data science, machine learning, and artificial intelligence are changing the world. Ginette: Data Crunch is produced by the Data Crunch Corporation, an analytics training and consulting company.Ginette: No matter what your position in a company is, knowing about data, how it works, and what it can do for you is vital to the success of your organization. Fortunately there are ways for you and those in your organization to learn about data. Brilliant dot org, an online educational resource, has on-demand classes in data basics that can help you understand this growing area, providing you with tools and the framework you need to break up complex concepts into bite-sized chunks. You can sign up for free, preview courses, and start learning by going to Brilliant.org/DataCrunch, and also the first 200 people that go to that link will get 20% off the annual premium subscription. Ginette: The CEO of Emperitas, Luciano Pesci, joins us today. Let’s get right into the episode. Curtis: What inspired you to get into data? What inspired you to to start the company you're working at now and how'd you get going? Luciano: All of it was a complete accident. Yeah, none of it, not the schooling, the business, none of it was intentional. Curtis: Okay, let's hear about it. Luciano: My first business was actually recording studio and a record label, and I had signed, among other acts, my own band, and we got a management deal, and we went to LA. We started to tour with national acts, and I thought that was going to be my career path without a doubt, and so I didn't take the ACT/SAT at the time, barely graduated high school, and then the band fell apart. And I was like, "well, what am I going to do?" So I went back to school, had a transformative experience, got drawn into economics, and then within economics really found data. Curtis: And what drew you to economics? Luciano: I like studying people. I think it's the most complete picture of people. So there's a lot of other disciplines that sort of dive deeper when it comes to people's psychological characteristics, their behavioral components. But economics was about the entire system and how an individual functions within that bigger system. And the reason I got to data from that was that the key assumption of modern economics is perfect information. So this is usually where critics of what is called the classical model in economics come in and say, "well, you can't have perfect information, so therefore you can't have optimizing behavior." And one of the beautiful lessons of the last 20 years, especially with data science is it might not be perfect information, but you can get really good information to make optimized choices. And so the represented that, that method of going into the real world and optimizing all these processes that we were learning about in the textbooks and at the abstract theory level. Curtis: Interesting. And that's, there's not a lot of places, if any, that I know of that teach that approach, right? Or have good coursework around that. Did you kind of figure this out on your own or how'd you, how'd you come to that?
Next Episode
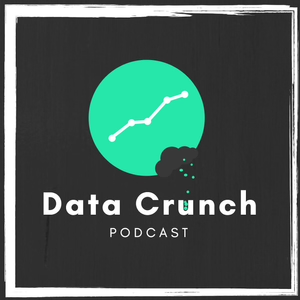
Social Media and Machine Learning
How do you build a comprehensive view of a topic on social media? Jordan Breslauer would say you let a machine learning tool scan the social sphere and add information as conversations evolve, with help from humans in the loop.Ginette Methot: I’m Ginette,Curtis Seare: and I’m Curtis,Ginette: and you are listening to Data Crunch,Curtis: a podcast about how applied data science, machine learning, and artificial intelligence are changing the world.Ginette: Data Crunch is produced by the Data Crunch Corporation, an analytics training and consulting company.Ginette: Many of you want to gain a deeper understanding of data science and machine learning, and Brilliant.org is a great place to dig deeper into these topics. Their classes help you understand algorithms, machine learning concepts, computer science basics, probability, computer memory, and many other important concepts in data science and machine learning. The nice thing about Brilliant.org is that you can learn in bite-sized pieces at your own pace. Their courses have storytelling, code-writing, and interactive challenges, which makes them entertaining, challenging, and educational.Sign up for free and start learning by going to Brilliant.org slash Data Crunch, and also the first 200 people that go to that link will get 20% off the annual premium subscription.Let’s get into our conversation with Jordan Breslauer, senior director of data analytics and customer success at social standards. Jordan: My name is Jordan Breslauer. I'm the senior director of data analytics and customer success at social standards. I've always been a data geek as it pertains to sports. I think of Moneyball when I was younger, I always wanted to be kind of a the next Billy Bean and I, when I started working for sports franchises right after high school and early college days, I just realized that, that type of work culture is wasn't for me, but I was so, so into trying to answer questions with data that had no previously clear answer, you know? I loved answering subjective questions like, or what makes the best player or how do, how do I know who the best player is? And I thought what was always fun was to try and bring some sort of structured subjectivity to those sorts of questions through using data. And that's really what got me passionate about data in the first place. But then I just started to apply it to a number of different business questions that I always thought were quite interesting, which have a great deal of subjectivity. And that led me to Nielsen originally where my main question that I was answering on a day-to-day basis, what was, what makes a great ad? Uh, what I found though is that advertising at least, especially as it pertains to TV, is really where brands were moving away from and a lot of the real consumer analytics that people were looking for were trying to underpin people in their natural environment, particularly on social media. And I hadn't seen any company that had done it well. Uh, and I happened to meet social standards during my time at Nielsen and was truly just blown away with this ability to essentially take a large input of conversations that people were happening or happening, I should say, and bring some sort of structure to them to actually be able to analyze them and understand what people were talking about as it pertained to different types of topics. And so I think that's really what brought me here was the fascination with this huge amount of data behind the ways that people were talking about on social. And the fact that it had some structure to it, which actually allowed for real analytics to be put behind it. Curtis: It's a hard thing to do though. Right? You know, to answer this question of how do we extract real value or real insight from social media and you'd mentioned historically or up to this point, companies that that are trying to do that missed the mark.
If you like this episode you’ll love
Episode Comments
Generate a badge
Get a badge for your website that links back to this episode
<a href="https://goodpods.com/podcasts/data-crunch-98898/deep-learning-microwaves-and-bugs-5229716"> <img src="https://storage.googleapis.com/goodpods-images-bucket/badges/generic-badge-1.svg" alt="listen to deep learning, microwaves, and bugs on goodpods" style="width: 225px" /> </a>
Copy